User login
Adverse events occur to patients after their discharge from acute care hospitals.1, 2 Most of these injuries are adverse drug events, procedure‐related events, nosocomial infections, or falls.1 Postdischarge adverse events are associated with several days of symptoms, nonpermanent disability, emergency department visits, or hospital readmission.1, 3 When adverse events are preventable or ameliorable, the most common root cause is poor communication between hospital personnel and either the patient or the outpatient primary care physician.1 In addition, there may be deficits in discharge processes related to assessment and communication of unresolved problems.1 Systematic reviews have shown that discharge communication is an inefficient and error‐prone process.46
One potential solution to poor discharge communication is health information technology.7 An example of technology is discharge software with a computerized physician order entry (CPOE) system. By definition, a CPOE system is a computer‐based system that automates direct entry of orders by physicians and ensures standardized, legible, and complete orders.8 The benefits of CPOE have been tested in other inpatient settings.8, 9 It is logical to consider software applications with CPOE for discharge interventions.7
Several mechanisms explain the potential benefit of discharge software with CPOE.7 Applications with CPOE decrease medication errors.8, 10 Software with decision support could prompt physicians to enter posthospitalization appointment dates and orders for preventive services.11, 12 Discharge software could facilitate medication reconciliation and generate patient instructions and information.4, 1315 The potential benefits of discharge software with CPOE provide a rationale for clinical trials to measure benefits.
Previous studies addressed discharge applications of health information technology. Observational studies recorded outcomes such as physician satisfaction.16, 17 Prior randomized clinical trials measured quality and timeliness of discharge summaries.18 However, these previous trials did not assess clinically relevant outcomes like readmissions, emergency department visits, or adverse events. We performed a cluster‐randomized trial to assess the value of a discharge software application of CPOE. The rationale for our clustered design complied with recommendations from a systematic review of discharge interventions.5 Our objective was to assess the benefit of discharge software with CPOE when used to discharge patients at high risk for repeat admission. After the intervention, we compared the rates of hospital readmission, emergency department visits, and postdischarge adverse events due to medical management.
Methods
The trial design was a cluster randomized, controlled trial with blinded outcome assessment. Follow‐up occurred until 6 months after discharge from index hospitalization at a 730‐bed, tertiary care, teaching hospital in central Illinois. The Peoria Institutional Review Board approved the protocol for human research.
Participants
The cluster definition was the hospital physician. Patients discharged by the physician comprised the cluster. Hospital physicians and patients were enrolled between November 2004 and January 2007. Internal medicine resident or attending physicians were eligible. We excluded hospital physicians if their assignments to inpatient duties were less than 2 months during the 27‐month enrollment period. The rationale for the physician exclusion was a consequence of the patient enrollment rate of 3 to 5 patients per physician per month. Physicians with brief assignments could not achieve the goal of 9 or more patients per cluster. After physicians gave informed consent to screen their patients, trained research coordinators applied inclusion and exclusion criteria and obtained informed consent from patients. Research personnel identified all consecutive, unique, adult inpatients who were discharged to home. Patient inclusion required a probability of repeat admission (Pra) score 0.40.19, 20 The Pra score came from a logistic model of age, gender, prior hospitalizations, prior doctor visits, self‐rated health status, presence of informal caregiver in the home, and comorbid coronary heart disease and diabetes mellitus. Research coordinators calculated the Pra within 2 days before discharge from the index hospitalization. Other details about exclusion criteria have been published.21 If a patient's outpatient primary care physician treated the patient during the index hospitalization, then there was no perceived barrier in physician‐to‐physician communication and we excluded the patient.
Intervention
The research intervention was a CPOE software application that facilitated communication at the time of hospital discharge to patients, retail pharmacists, and community physicians. Details about the discharge software appeared in a previous publication.7 Software features included required fields, pick lists, standard drug doses, alerts, reminders, and online reference information. The software prompted the discharging physician to enter pending tests and order tests after discharge. Hospital physicians used the software on the day of discharge and automatically generated 4 discharge documents. The first document was a personalized letter to the outpatient physician with discharge diagnoses, reconciled medication list, diet and activity instructions, patient education materials provided, and follow‐up appointments and studies. Second, the software printed legible prescriptions along with specific information for the dispensing pharmacist about changes and deletions in the patient's previous regimen. Third, the software created patient instructions with addresses and telephone numbers for follow‐up appointments and tests. Fourth, the software printed a legible discharge order including all of the aforementioned information.
The control intervention was the usual care discharge process as described previously.7 Hospital physicians and ward nurses completed handwritten discharge forms on the day of discharge. The forms contained blanks for discharge diagnoses, discharge medications, medication instructions, postdischarge activities and restrictions, postdischarge diet, postdischarge diagnostic and therapeutic interventions, and appointments. Patients received handwritten copies of the forms, 1 page of which also included medication instructions and prescriptions. A previous publication gave details about the standard care available to all patients regardless of intervention.7
Randomization
The unit of randomization was the hospital physician who performed the discharge process. Random allocation was to discharge software or usual care discharge process. The randomization ratio was 1:1, the block size was 2, and there was no stratification or matching. There was concealed allocation and details are available from the investigators. Hospital physicians subsequently used their randomly assigned process when discharging their patients who enrolled in the study. After random allocation, it was not possible to conceal the test or control intervention from physicians or their patients. Likewise, it was not possible to conceal the outcome ascertainment, including readmission, from the hospital physicians.
All hospital physicians received training on the usual care discharge process. Physicians assigned to discharge software completed additional training via multimedia demonstration with 1‐on‐1 coaching as needed. Physicians assigned to usual care did not receive training on the discharge software and were blocked from using the software. After informed consent, patients were passive recipients of the research intervention performed by their discharging physician. Patients received the research intervention on the day of discharge from the index hospitalization.
The baseline assessment of patient characteristics occurred during the index hospitalization. Trained data abstractors recorded patient demographic data plus variables to calculate the Pra score for probability for repeat admission. We recorded additional variables because of their possible association with readmission.15, 2229 Data came from the patient or proxy for physical functioning and mental health (SF‐36, Version 2; Medical Outcomes Trust, Boston, MA). Other data for predictor variables came from interviews or hospital records.
Outcome Assessment
The primary study outcome was the proportion of patients readmitted at least once within 6 months after the index hospitalization. Readmission was for any reason and included observation and full admission status. Secondary outcomes were emergency department visits that did not result in hospital admission. Outcome assessment occurred at the patient level. We obtained data for readmissions and emergency department visits from 6 hospitals in central Illinois where study patients were likely to seek care. We validated readmissions and emergency department visits via patient/proxy telephone interviews that occurred 6 months after index hospital discharge. Interviewers were blind to intervention assignment. We evaluated the adequacy of the blind and asked interviewers to guess the patient's intervention assignment.
Another secondary outcome was the proportion of patients who experienced an adverse event related to medical management within 1 month after discharge. For adverse event ascertainment, we employed the process of Forster et al.1, 2 Within 20 to 40 days after discharge, an internal medicine physician performed telephone interviews with the patient or proxy. The interviewer recorded symptoms, drug information, other treatment, hospital readmissions, and emergency department visits. Another physician compiled case summaries from interview data and information abstracted from the electronic medical record, including dictated discharge summaries from the index hospitalization and postdischarge emergency department visits, diagnostic test results, and readmission reports. Two additional internal medicine physicians adjudicated each case summary separately. We counted adverse events only when adjudicators agreed that medical management probably or definitely caused the event. The initial rating by each adjudicator revealed moderate‐to‐good agreement (Kappa = 0.52).30 When initial adjudications were discordant, then adjudicators met and resolved all discrepancies. The adjudicators also scored the severity of the adverse event. The severity scale options were serious laboratory abnormality only, 1 day of symptoms, several days of symptoms, nonpermanent disability, permanent disability, or death. The adjudicators also scored the adverse event as preventable (yes/no), ameliorable (yes/no), and recorded system problems associated with preventable and ameliorable adverse events.1 For adverse drug events, the adjudicators recorded preventability categories defined by previous investigators.31 We designed the adverse event outcome ascertainment as a blinded process. We evaluated the success of the blind and asked adjudicators to guess the patient's intervention assignment.
Sample Size
The sample size analysis employed several assumptions regarding the proportion of readmitted patients. The estimated readmission rate after usual care was 37%.24, 3236 The minimum clinically relevant difference in readmission rates was 13%, an empirical boundary for quantitative significance.37 Estimates for intracluster correlation were not available when we designed the trial. We projected intracluster correlations with low, medium, and high values. The cluster number and size were selected to maintain test significance level, 1‐sided alpha, <0.05 and power >80%. The sample size assumed no interim analysis. The initial sample size estimates were 11 physician clusters per intervention with 25 patients per cluster. During the first 2 months of patient recruitment, we observed that we could not consistently achieve clusters with 25 patients. We recalculated the sample size. Using the same assumptions, we found we could achieve similar test significance and power with 35 physician clusters per intervention and 9 patients per cluster. The sample size calculator was nQuery (Statistical Solutions, Saugus, MA).
Statistical Methods
Analyses were performed with SPSS PC (Version 15.0.1; SPSS Inc, Chicago, IL). Using descriptive statistics, we reported baseline variables as means and standard deviations (SD) for interval variables, and percentages for categorical variables. For outcome variables, we utilized the principle of intention‐to‐treat and assumed patient exposure to the intervention randomly assigned to their discharging physician. We inspected scatter plots and correlations for all variables to test assumptions regarding normal distribution, homogeneity of variance, and linearity of relationships between independent and dependent variables. When assumptions failed, we stratified variables (median or thirds) or performed transformations to satisfy assumptions. For patient‐level outcome variables, we calculated intracluster correlation coefficients. The assessment of the blind was unaffected by the cluster assumption so we used the chi‐square procedure. For analysis of time to event, we used Kaplan‐Meier plots.
The primary hypothesis was a significant decrease in the primary readmission outcome for patients assigned to discharge software. We tested the primary hypothesis with generalized estimating equations that corrected for clustering by hospital physician and adjusted for covariates that predicted readmission. The intervention variable was discharge software versus usual care handwritten discharge. We reported parameter estimates of the intervention variable coefficient and Wald 95% confidence interval (95% CI) with and without correction for cluster. For the secondary, patient‐level outcomes, we performed similar analyses with generalized estimating equations that corrected for clustering by hospital physician.
During covariate analysis, we screened all baseline variables for their correlation with readmission. The variable with the highest correlation and P value <0.05 entered initially in the general estimating equation. After initial variable entry, we evaluated subsequent variables with partial correlations that controlled for variables entered previously. At each iterative step, we entered into the model the variable with the highest partial correlation and P value <0.05.
In exploratory analyses, we examined intervention group differences within strata defined by covariates that predicted readmission. We used generalized estimating equations and adjusted for the other covariates that predicted readmission.
Results
We screened 127 physicians who were general internal medicine hospital physicians. Seventy physicians consented and received random allocation to discharge software or usual care. The physician characteristics appear in Table 1. Most of the hospital physicians were interns in the first year of postgraduate training (58.6%; 41/70). We excluded 57 physicians for reasons shown in the trial flow diagram (Figure 1). The most common reason for hospital physician exclusion applied to resident physicians in their last months of training before graduation or emergency department residents temporarily assigned to internal medicine training. We approached 6,884 patients during their index hospitalization. After excluding 6,253 ineligible patients, we enrolled and followed 631 patients who received the discharge intervention (Figure 1). During 6 months of follow‐up, a small proportion of patients died (3%; 20/631). Hospital records were available for deceased patients and they were included in the analysis. A small proportion (6%; 41/631) of patients withdrew consent or left the trial for other reasons during 6 months. There was no differential dropout between the interventions. Protocol deviations were rare (0.5%; 3/631). Three patients erroneously received usual care discharge from physicians assigned to discharge software. All 631 patients were included in the intention‐to‐treat analysis. The baseline characteristics of the randomly‐assigned hospital physicians and their patients are in Table 1.
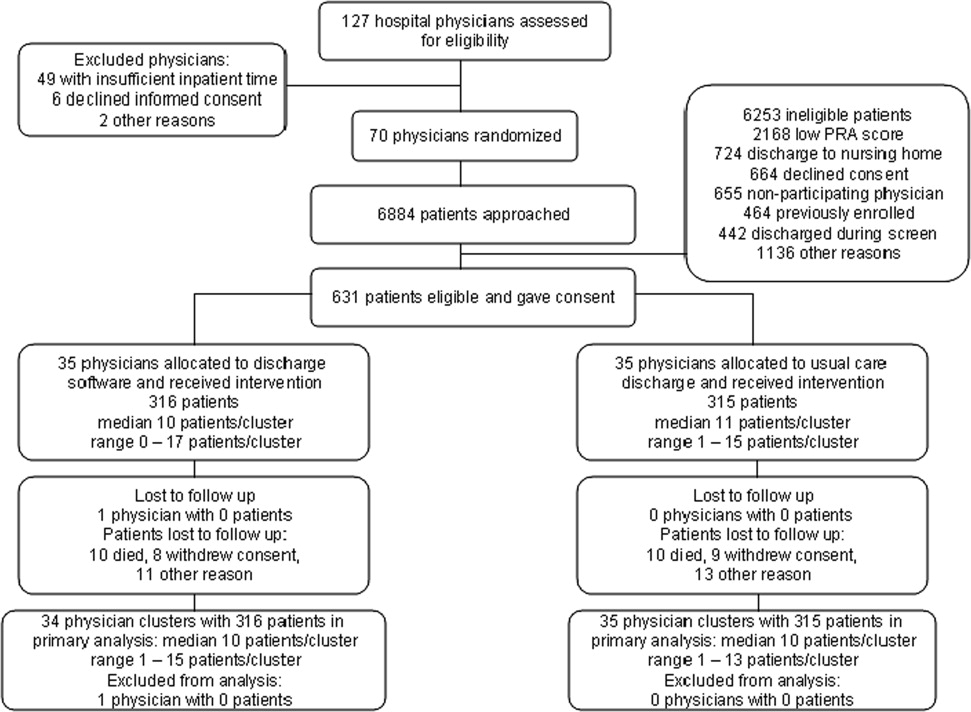
Discharge Software | Usual Care | |
---|---|---|
| ||
Hospital physician characteristics, n (%) | (n = 35) | (n = 35) |
Postgraduate year 1 | 18 (51.4) | 23 (65.7) |
Postgraduate years 2‐4 | 10 (28.6) | 7 (20.0) |
Attending physician | 7 (20.0) | 5 (14.3) |
Patient characteristics | (n = 316) | (n = 315) |
Gender, female, n (%) | 180 (57.0) | 168 (53.3) |
Age, years, n (%) | ||
18‐44 | 68 (21.5) | 95 (30.2) |
45‐54 | 79 (25.0) | 76 (24.1) |
55‐64 | 86 (27.2) | 74 (23.5) |
65‐98 | 83 (26.3) | 70 (22.2) |
Race, n (%) | ||
Caucasian | 239 (75.6) | 229 (72.7) |
Black | 72 (22.8) | 85 (27.0) |
Other | 5 (1.6) | 1 (0.3) |
Self‐rated health status, n (%) | ||
Poor | 82 (25.9) | 108 (34.3) |
Fair | 169 (53.5) | 147 (46.7) |
Good | 54 (17.1) | 46 (14.6) |
Very good | 10 (3.2) | 11 (3.5) |
Excellent | 1 (0.3) | 3 (1.0) |
Diabetes mellitus, n (%) | 172 (54.4) | 177 (56.2) |
Chronic obstructive pulmonary disease, n (%) | ||
None | 259 (82.0) | 257 (81.6) |
Without oral steroid or home oxygen | 28 (8.9) | 26 (8.3) |
With chronic oral steroid | 10 (3.2) | 8 (2.5) |
With home oxygen oral steroid | 19 (6.0) | 24 (7.6) |
Coronary heart disease, n (%) | 133 (42.1) | 120 (38.1) |
Heart failure, n (%) | 80 (25.3) | 67 (21.3) |
Informal caregiver available, yes, n (%) | 313 (99.1) | 313 (99.4) |
Taking loop diuretic, n (%) | 110 (34.8) | 88 (27.9) |
Physical functioning from SF‐36, n (%) | ||
Lowest third | 128 (40.5) | 121 (38.4) |
Upper two‐thirds | 188 (59.5) | 194 (61.6) |
Mental health from SF‐36, n (%) | ||
Lowest third | 113 (35.8) | 117 (37.1)* |
Upper two‐thirds | 203 (64.2) | 197 (62.5)* |
Hospital admissions during year prior to index admission, n (%) | ||
0 or 1 | 247 (78.2) | 224 (71.1) |
2 or more | 69 (21.8) | 91 (28.9) |
Emergency department visits during 6 months before index admission, n (%) | ||
0 or 1 | 194 (61.4) | 168 (53.3) |
2 or more | 122 (38.6) | 147 (46.7) |
Outpatient doctor or clinic visits during year prior to index admission | ||
0 to 4 | 97 (30.7%) | 77 (24.4%) |
5 to 8 | 68 (21.5%) | 81 (25.7%) |
9 to 12 | 82 (25.9%) | 84 (26.7%) |
13 or more | 69 (21.8%) | 73 (23.2%) |
Insurance or payor | ||
Medicare, age less than 65 years | 18 (5.7%) | 13 (4.1%) |
Medicare, age 65 years and older | 56 (17.7%) | 40 (12.7%) |
Medicaid, age less than 65 years | 98 (31.0%) | 130 (41.3%) |
Medicaid, age 65 years and older | 17 (5.4%) | 20 (6.3%) |
Commercial or veteran | 85 (26.9%) | 61 (19.4%) |
Self‐pay | 42 (13.3%) | 51 (16.2%) |
Religious participation | ||
Never | 159 (50.3%) | 164 (52.1%) |
1‐24 times per year | 55 (17.4%) | 51 (16.2%) |
1‐7 times per week | 102 (32.3%) | 100 (31.7%) |
Volunteer activity, 1 or more hour/month | 31 (9.8%) | 39 (12.4%) |
Employment status | ||
Not working | 229 (72.5%) | 233 (74.4%)* |
Part‐time (<37.5 hours/week) | 30 (9.5%) | 25 (8.0%)* |
Full‐time (at least 37.5 hour/week) | 57 (18.0%) | 55 (17.6%)* |
Number of discharge medications, mean (SD) | 10.5 (4.8) | 9.9 (5.1) |
Severity of illness, mean (SD) | 1.8 (1.2) | 1.6 (1.3) |
Charlson‐Deyo comorbidity, mean (SD) | 1.7 (1.4) | 1.6 (1.9) |
Index hospital length of stay, days, mean (SD) | 3.9 (3.5) | 3.5 (3.5) |
Blood urea nitrogen, mean (SD) | 17.9 (12.9) | 19.1 (12.9) |
Probability of repeat admission, Pra, mean (SD) | 0.486 (0.072) | 0.495 (0.076) |
We asked outpatient physicians about their receipt of discharge communication from hospital physicians. The text of the question was, How soon after discharge did you receive any information (in any form) relating to this patient's hospital admission and discharge plans? We mailed the question 10 days after discharge to outpatient physicians designated by patients enrolled in the study. Among patients in the discharge software group, 75.0% (237/316) of their outpatient physicians responded to the question. The response rate was 80.6% (254/315) from physicians who followed patients in the usual care group. Respondents from the discharge software group said within 1‐2 days or within a week for 56.0% (177/316) of patients. Respondents from the usual care group said within 1‐2 days or within a week for 57.1% (180/315) of patients. The difference between the intervention groups, 1.1% (95% CI, 9.2% to 6.9%), was not significant.
The primary, prespecified, outcome of the study was the proportion of patients with at least 1 readmission to the hospital. After intervention with discharge software versus usual care, there was no significant difference in readmission rates (Table 2) or time to first readmission (Figure 2). We screened all baseline variables in Table 1 and sought predictors of readmission to employ in adjusted models. For example, we evaluated physician level of training because we wondered if experience or seniority affected readmission when hospital physicians used the discharge software or usual care discharge. The candidate variable, physician level of training, did not correlate with readmission (rho = 0.066; P = 0.100), so it was dropped from subsequent analyses. After screening all variables in Table 1, we found 4 independent predictors of readmission: previous hospitalizations, previous emergency department visits, heart failure, and physical function. Generalized estimating equations for readmission that adjusted for predictor variables confirmed a negligible parameter estimate for the discharge intervention variable coefficient (Table 2).
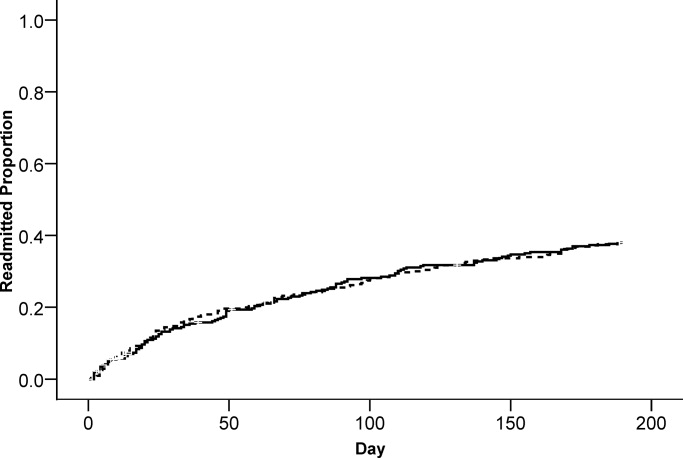
Outcome | Discharge Software, n (%) | Usual Care, n (%) | Parameter Estimate Without Cluster Correction Intervention Coefficient (95% CI) | P Value | Parameter Estimate With Cluster Correction Intervention Coefficient (95% CI) | P Value |
---|---|---|---|---|---|---|
| ||||||
Readmitted within 6 months | 117 (37.0%) | 119 (37.8%) | 0.005* (0.076, 0.067) | 0.897 | 0.005* (0.074, 0.065) | 0.894 |
Emergency department visit within 6 months | 112 (35.4%) | 128 (40.6%) | 0.052 (0.128, 0.024) | 0.179 | 0.052 (0.115, 0.011) | 0.108 |
Adverse event within 1 month | 23 (7.3%) | 23 (7.3%) | 0.003 (0.037, 0.043) | 0.886 | 0.003 (0.037, 0.043) | 0.884 |
We evaluated emergency department visits that were unrelated to readmission as secondary, prespecified, outcomes. The results were similar to readmission results. While the proportion of patients with at least 1 emergency department visit was lower for the discharge software intervention, the difference with usual care was not significant (Table 2). There was no significant difference between interventions for time to first emergency department visit (Figure 3).
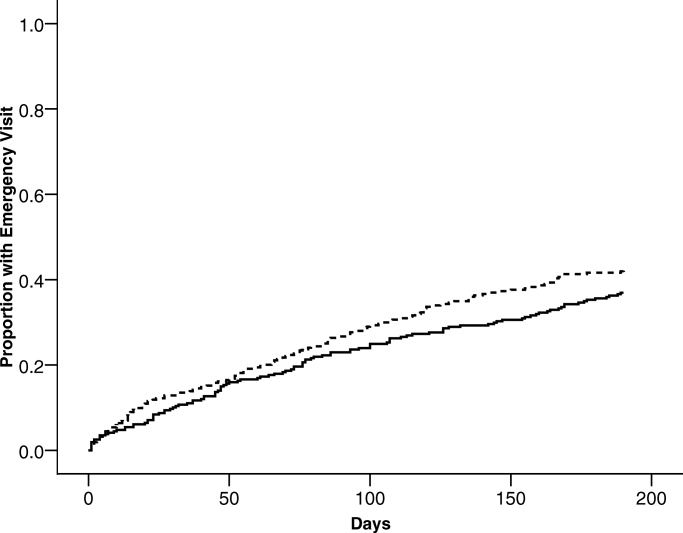
Postdischarge adverse events were secondary, prespecified, outcomes. Data for adverse event adjudication were available for 98% (309/316) of discharge software patients and 97% (307/315) of usual care patients. Within 1 month after discharge, 46 patients had adverse events probably or definitely related to medical management. Two patients had 2 events and 1 patient had 3 events. For analysis, we randomly selected 1 event per patient. When comparing patients assigned to discharge software versus usual care, there were no differences in adverse events related to medical management (Table 2). Most of the events were possible adverse drug events (74%; 34/46). The adverse event severity was several days of symptoms or nonpermanent disability for 76% (35/46) of the adverse events. Adjudicators rated 26% (12/46) of the adverse events as preventable and 46% (21/46) as ameliorable. The absolute numbers of events were small. There were no differences between discharge software and usual care patients within adverse event strata defined by type, severity, preventable, or ameliorable (Table 3). For most of the patients with adverse events, the adjudicators could not identify a system problem or preventability category (Table 3). When a deficiency was evident, there was no pattern to suggest a significant difference between discharge software patients versus usual care patients.
Discharge Software (n) | Usual Care Discharge (n) | |
---|---|---|
| ||
At least 1 adverse event | 23 | 23 |
Preventable adverse event | 7 | 5 |
Ameliorable adverse event | 9 | 12 |
Adverse event severity | ||
Serious laboratory abnormality only or 1 day of symptoms | 5 | 5 |
Several days of symptoms or nonpermanent disability | 18 | 17 |
Permanent disability or death | 0 | 1 |
Adverse event by type | ||
Possible adverse drug event | 17 | 17 |
Procedure‐related injury | 2 | 1 |
Therapeutic error | 4 | 4 |
Diagnostic error | 0 | 1 |
System problems associated with preventable or ameliorable adverse events | ||
Inadequate patient education regarding the medical condition or its treatment | 0 | 1 |
Poor communication between patient and physician | 2 | 1 |
Poor communication between hospital and community physicians | 0 | 0 |
Inadequate monitoring of the patient's illness after discharge | 0 | 6 |
Inadequate monitoring of the patient's treatment after discharge | 2 | 6 |
No emergency contact number given to patient to call about problems | 0 | 0 |
Patient with problems getting prescribed medications immediately | 1 | 0 |
Inadequate home services | 0 | 0 |
Delayed follow‐up care | 0 | 3 |
Premature hospital discharge | 1 | 2 |
Adverse drug event (ADE) preventability categories | ||
Drug involved in the ADE inappropriate for the clinical condition | 2 | 4 |
Dose, route, or frequency inappropriate for age, weight, creatinine clearance, or disease | 1 | 2 |
Failure to obtain required lab tests and/or drug levels | 1 | 2 |
Prior history of an adverse event or allergy to the drug | 1 | 2 |
Drug‐drug interaction involved in the ADE | 2 | 0 |
Toxic serum drug level documented | 0 | 0 |
Noncompliance involved in the ADE | 0 | 1 |
When we designed the trial, we assumed variance in outcomes measured at the patient level. We predicted some variance attributable to clustering by hospital physician. After the trial, we calculated the intracluster correlation coefficients for readmissions, emergency department visits, and adverse events. For all of these outcomes, the intracluster correlation coefficients were negligible. We also evaluated generalized estimating equations with and without correction for hospital physician cluster. We confirmed the negligible cluster effect on confidence intervals for intervention coefficients (Table 2).
We performed an exploratory stratified analysis. We evaluated the intervention effect on readmission within subgroups defined by covariates that predicted readmission (Table 4). When the intervention groups were compared within baseline categories of previous hospitalizations, previous emergency department visits, heart failure, and physical functioning, there was a consistent pattern with no differential effect by intervention assignment. None of the intervention coefficients were statistically significant (Table 4).
Subgroup | Discharge Software Readmitted n/n (%) | Usual Care Readmitted n/n (%) | Adjusted Parameter Estimate Intervention Coefficient (95% CI) |
---|---|---|---|
| |||
Hospital admissions during year prior to index admission | |||
0 or 1 | 77/247 (31.2) | 73/224 (32.6) | 0.025 (0.095, 0.045)* |
2 or more | 40/69 (58.0) | 46/91 (50.5) | 0.059 (0.090, 0.208)* |
Emergency department visits during 6 months before index admission | |||
0 or 1 | 64/194 (33.0) | 45/168 (26.8) | 0.033 (0.047, 0.113) |
2 or more | 53/122 (43.4) | 74/147 (50.3) | 0.071 (0.188, 0.046) |
Heart failure | |||
Present | 40/80 (50.0) | 36/67 (53.7) | 0.024 (0.224, 0.177) |
Absent | 77/236 (32.6) | 83/248 (33.5) | 0.000 (0.076, 0.075) |
Physical functioning from SF‐36 | |||
Lowest third | 55/128 (43.0) | 59/121 (48.8) | 0.032 (0.161, 0.096) |
Upper two‐thirds | 62/188 (33.0) | 60/194 (30.9) | 0.012 (0.071, 0.095) |
Assessment of the Success of the Blind
We evaluated the adequacy of the blind for outcome assessors who interviewed patients or adjudicated adverse events. The guesses of outcomes assessors were unrelated to true intervention assignment (all P values >0.097). We interpreted the blind as adequate for outcome assessors who recorded readmissions, emergency department visits, and adverse events.
Discussion
We performed a cluster‐randomized clinical trial to measure the effects of discharge software versus usual care handwritten discharge. The discharge software with CPOE implemented elements of high‐quality discharge planning and communication endorsed by the National Quality Forum and systematic reviews.6, 38 Despite theoretical benefits, our discharge software intervention did not reduce readmissions or emergency department visits. What were potential explanations for our results? We assumed an association between postdischarge adverse events and readmissions or emergency department visits.1 Our failure to reduce adverse events might explain the failure to reduce readmissions or emergency department visits. Another potential explanation was related to adverse drug events. Other investigators showed most postdischarge adverse events were adverse drug events and our data confirmed previous studies.1, 2 Medication reconciliation at discharge was a potential mechanism for adverse drug event reduction.14 Medication reconciliation was the standard at the study hospital, so it was unethical to deny reconciliation to patients assigned to either intervention.39 Required medication reconciliation in both groups, by its known effect on preventable adverse drug events, might have reduced the event rates in both groups.14 This possibility is supported by the low rate of adverse events observed in our study compared with other studies.1 We speculate that the low background rate of adverse events at the study hospital may have minimized events in both the discharge software and usual care groups and prevented detection of software benefits, if present.39
One limitation of our study may have been the discharge software. The automated decision support in our software lacked features that might have improved outcomes. For example, the software did not generate a list of diagnostic test results that were pending at the time of discharge. Our software relied on prompts to the physician user that did not specify which tests were pending. The software did not perform error checks on the discharge orders to warn physicians about drug‐drug interactions, therapeutic duplications, or missing items (eg, immunizations, drugs, education). The absence of these software enhancements made our discharge process vulnerable to the lapses and slips of the physician user. Whether or not such enhancements affect clinically relevant outcomes remains a testable hypothesis for future studies.
Another limitation of our study was the outpatient physician response. Discharge software did not increase the proportion of outpatient physicians who said they received communication within 7 days after hospital discharge. Our intervention addressed the sending partner but not the receiving partner in the communication dyad. Our discharge software was not designed to change information flow within the outpatient physician office. We do not know if discharge communication arrived and remained unnoticed until the patient called or visited the outpatient clinic. Future studies of discharge communication should consider a closed loop design to assure receipt and comprehension.
When we designed our study, we expected at least some variance between patient clusters attributable to the physician who performed the discharge. Our analysis of intracluster correlation revealed negligible variance. We speculate the highly‐standardized discharge process implemented by discharge software and usual care at our hospital resulted in minimal variance. Future studies of discharge interventions may consider designs that avoid cluster randomization.
In conclusion, a discharge software application of CPOE did not affect readmissions, emergency department visits, or adverse events after discharge.
Acknowledgements
The authors thank Howard S. Cohen, MD, for his review of the trial protocol and the manuscript.
- The incidence and severity of adverse events affecting patients after discharge from the hospital.Ann Intern Med.2003;138(3):161–167. , , , , .
- Adverse events among medical patients after discharge from hospital.CMAJ.2004;170(3):345–349. , , , et al.
- Frequency of new or worsening symptoms in the posthospitalization period.J Hosp Med.2007;2(2):58–68. , , , , .
- Written and verbal information versus verbal information only for patients being discharged from acute hospital settings to home.Cochrane Database Syst Rev.2003;(4):CD003716. , , .
- Discharge planning from hospital to home.Cochrane Database Syst Rev.2004;(1):CD000313. , , , .
- Deficits in communication and information transfer between hospital‐based and primary care physicians: implications for patient safety and continuity of care.JAMA.2007;297(8):831–841. , , , , , .
- Software design to facilitate information transfer at hospital discharge.Inform Prim Care.2006;14(2):109–119. , , .
- Effects of computerized physician order entry and clinical decision support systems on medication safety: a systematic review.Arch Intern Med.2003;163(12):1409–1416. , , .
- Computer physician order entry: benefits, costs, and issues.Ann Intern Med.2003;139(1):31–39. , .
- Systematic review: impact of health information technology on quality, efficiency, and costs of medical care.Ann Intern Med.2006;144(10):742–752. , , , et al.
- Compliance with post‐hospitalization follow‐up visits: rationing by inconvenience?Ethn Dis.1999;9(3):387–395. , , , , , .
- A computerized reminder system to increase the use of preventive care for hospitalized patients.N Engl J Med.2001;345(13):965–970. , , , , , .
- Evaluation of a new integrated discharge prescription form.Ann Pharmacother.2001;35(7‐8):953–958. , , , .
- Role of pharmacist counseling in preventing adverse drug events after hospitalization.Arch Intern Med.2006;166(5):565–571. , , , et al.
- Factors associated with unplanned hospital readmission among patients 65 years of age and older in a Medicare managed care plan.Am J Med.1999;107(1):13–17. , , , , , .
- Closing the loop of patient care—a clinical trial of a computerized discharge medication program.Proc Annu Symp Comput Appl Med Care.1994:841–845. , .
- A comprehensive inpatient discharge system.Proc AMIA Annu Fall Symp.1996:699–703. , , , .
- Agency for Healthcare Research and Quality. Making health care safer: a critical analysis of patient safety practices, subchapter 42.3. Discharge summaries and follow‐up. Available at: http://www.ahrq.gov/clinic/ptsafety/chap42b. htm#42.3. Accessed January 2009.
- Predictive validity of a questionnaire that identifies older persons at risk for hospital admission.J Am Geriatr Soc.1995;43(4):374–377. , , .
- Predictive validity of the Pra instrument among older recipients of managed care.J Am Geriatr Soc.1997;45(5):614–617. , , , .
- Brief scale measuring patient preparedness for hospital discharge to home: psychometric properties.J Hosp Med.2008;3(6):446–454. , , .
- SF‐36 health survey update.Spine.2000;25(24):3130–3139. .
- Development of a method to identify seniors at high risk for high hospital utilization.Med Care.2002;40(9):782–793. , , , , , .
- Comprehensive discharge planning and home follow‐up of hospitalized elders: arandomized clinical trial.JAMA.1999;281(7):613–620. , , , et al.
- Predicting non‐elective hospital readmissions: a multi‐site study. Department of Veterans Affairs Cooperative Study Group on Primary Care and Readmissions.J Clin Epidemiol.2000;53:1113–1118. , , , et al.
- Identification of factors associated with hospital readmission and development of a predictive model.Health Serv Res.1992;27(1):81–101. , .
- Risk‐adjusting acute myocardial infarction mortality: are APR‐DRGs the right tool?Health Serv Res.2000;34(7):1469–1489. , .
- Adapting a clinical comorbidity index for use with ICD‐9‐CM administrative databases.J Clin Epidemiol.1992;45(6):613–619. , , .
- The community assessment risk screen (CARS): identifying elderly persons at risk for hospitalization or emergency department visit.Am J Manag Care.2000;6(8):925–933. , , .
- Clinical Epidemiology: A Basic Science for Clinical Medicine.2nd ed.Boston:Little, Brown;1991. , , , .
- Identifying clinically significant preventable adverse drug events through a hospital's database of adverse drug reaction reports.Am J Health Syst Pharm.2002;59(18):1742–1749. , , , , .
- A pharmacy discharge plan for hospitalized elderly patients—a randomized controlled trial.Age Ageing.2001;30(1):33–40. , , , , , .
- Can GP input into discharge planning result in better outcomes for the frail aged: results from a randomized controlled trial.Fam Pract.1999;16(3):289–293. , , , , .
- Comprehensive discharge planning with postdischarge support for older patients with congestive heart failure: a meta‐analysis.JAMA.2004;291(11):1358–1367. , , , , , .
- Can readmission after stroke be prevented? Results of a randomized clinical study: a postdischarge follow‐up service for stroke survivors.Stroke.2000;31(5):1038–1045. , , , , , .
- Does increased access to primary care reduce hospital readmissions? Veterans Affairs Cooperative Study Group on Primary Care and Hospital Readmission.N Engl J Med.1996;334(22):1441–147. , , .
- Indexes and boundaries for “quantitative significance” in statistical decisions.J Clin Epidemiol.1990;43(12):1273–1284. , , .
- National Quality Forum. Safe Practices for Better Healthcare 2006 Update, A Consensus Report, Safe Practice 11: Discharge Systems. Available at: http://qualityforum.org/pdf/reports/safe_practices/txsppublic.pdf. Accessed January 2009.
- OSF Healthcare's journey in patient safety.Qual Manag Health Care.2004;13(1):53–59. , .
Adverse events occur to patients after their discharge from acute care hospitals.1, 2 Most of these injuries are adverse drug events, procedure‐related events, nosocomial infections, or falls.1 Postdischarge adverse events are associated with several days of symptoms, nonpermanent disability, emergency department visits, or hospital readmission.1, 3 When adverse events are preventable or ameliorable, the most common root cause is poor communication between hospital personnel and either the patient or the outpatient primary care physician.1 In addition, there may be deficits in discharge processes related to assessment and communication of unresolved problems.1 Systematic reviews have shown that discharge communication is an inefficient and error‐prone process.46
One potential solution to poor discharge communication is health information technology.7 An example of technology is discharge software with a computerized physician order entry (CPOE) system. By definition, a CPOE system is a computer‐based system that automates direct entry of orders by physicians and ensures standardized, legible, and complete orders.8 The benefits of CPOE have been tested in other inpatient settings.8, 9 It is logical to consider software applications with CPOE for discharge interventions.7
Several mechanisms explain the potential benefit of discharge software with CPOE.7 Applications with CPOE decrease medication errors.8, 10 Software with decision support could prompt physicians to enter posthospitalization appointment dates and orders for preventive services.11, 12 Discharge software could facilitate medication reconciliation and generate patient instructions and information.4, 1315 The potential benefits of discharge software with CPOE provide a rationale for clinical trials to measure benefits.
Previous studies addressed discharge applications of health information technology. Observational studies recorded outcomes such as physician satisfaction.16, 17 Prior randomized clinical trials measured quality and timeliness of discharge summaries.18 However, these previous trials did not assess clinically relevant outcomes like readmissions, emergency department visits, or adverse events. We performed a cluster‐randomized trial to assess the value of a discharge software application of CPOE. The rationale for our clustered design complied with recommendations from a systematic review of discharge interventions.5 Our objective was to assess the benefit of discharge software with CPOE when used to discharge patients at high risk for repeat admission. After the intervention, we compared the rates of hospital readmission, emergency department visits, and postdischarge adverse events due to medical management.
Methods
The trial design was a cluster randomized, controlled trial with blinded outcome assessment. Follow‐up occurred until 6 months after discharge from index hospitalization at a 730‐bed, tertiary care, teaching hospital in central Illinois. The Peoria Institutional Review Board approved the protocol for human research.
Participants
The cluster definition was the hospital physician. Patients discharged by the physician comprised the cluster. Hospital physicians and patients were enrolled between November 2004 and January 2007. Internal medicine resident or attending physicians were eligible. We excluded hospital physicians if their assignments to inpatient duties were less than 2 months during the 27‐month enrollment period. The rationale for the physician exclusion was a consequence of the patient enrollment rate of 3 to 5 patients per physician per month. Physicians with brief assignments could not achieve the goal of 9 or more patients per cluster. After physicians gave informed consent to screen their patients, trained research coordinators applied inclusion and exclusion criteria and obtained informed consent from patients. Research personnel identified all consecutive, unique, adult inpatients who were discharged to home. Patient inclusion required a probability of repeat admission (Pra) score 0.40.19, 20 The Pra score came from a logistic model of age, gender, prior hospitalizations, prior doctor visits, self‐rated health status, presence of informal caregiver in the home, and comorbid coronary heart disease and diabetes mellitus. Research coordinators calculated the Pra within 2 days before discharge from the index hospitalization. Other details about exclusion criteria have been published.21 If a patient's outpatient primary care physician treated the patient during the index hospitalization, then there was no perceived barrier in physician‐to‐physician communication and we excluded the patient.
Intervention
The research intervention was a CPOE software application that facilitated communication at the time of hospital discharge to patients, retail pharmacists, and community physicians. Details about the discharge software appeared in a previous publication.7 Software features included required fields, pick lists, standard drug doses, alerts, reminders, and online reference information. The software prompted the discharging physician to enter pending tests and order tests after discharge. Hospital physicians used the software on the day of discharge and automatically generated 4 discharge documents. The first document was a personalized letter to the outpatient physician with discharge diagnoses, reconciled medication list, diet and activity instructions, patient education materials provided, and follow‐up appointments and studies. Second, the software printed legible prescriptions along with specific information for the dispensing pharmacist about changes and deletions in the patient's previous regimen. Third, the software created patient instructions with addresses and telephone numbers for follow‐up appointments and tests. Fourth, the software printed a legible discharge order including all of the aforementioned information.
The control intervention was the usual care discharge process as described previously.7 Hospital physicians and ward nurses completed handwritten discharge forms on the day of discharge. The forms contained blanks for discharge diagnoses, discharge medications, medication instructions, postdischarge activities and restrictions, postdischarge diet, postdischarge diagnostic and therapeutic interventions, and appointments. Patients received handwritten copies of the forms, 1 page of which also included medication instructions and prescriptions. A previous publication gave details about the standard care available to all patients regardless of intervention.7
Randomization
The unit of randomization was the hospital physician who performed the discharge process. Random allocation was to discharge software or usual care discharge process. The randomization ratio was 1:1, the block size was 2, and there was no stratification or matching. There was concealed allocation and details are available from the investigators. Hospital physicians subsequently used their randomly assigned process when discharging their patients who enrolled in the study. After random allocation, it was not possible to conceal the test or control intervention from physicians or their patients. Likewise, it was not possible to conceal the outcome ascertainment, including readmission, from the hospital physicians.
All hospital physicians received training on the usual care discharge process. Physicians assigned to discharge software completed additional training via multimedia demonstration with 1‐on‐1 coaching as needed. Physicians assigned to usual care did not receive training on the discharge software and were blocked from using the software. After informed consent, patients were passive recipients of the research intervention performed by their discharging physician. Patients received the research intervention on the day of discharge from the index hospitalization.
The baseline assessment of patient characteristics occurred during the index hospitalization. Trained data abstractors recorded patient demographic data plus variables to calculate the Pra score for probability for repeat admission. We recorded additional variables because of their possible association with readmission.15, 2229 Data came from the patient or proxy for physical functioning and mental health (SF‐36, Version 2; Medical Outcomes Trust, Boston, MA). Other data for predictor variables came from interviews or hospital records.
Outcome Assessment
The primary study outcome was the proportion of patients readmitted at least once within 6 months after the index hospitalization. Readmission was for any reason and included observation and full admission status. Secondary outcomes were emergency department visits that did not result in hospital admission. Outcome assessment occurred at the patient level. We obtained data for readmissions and emergency department visits from 6 hospitals in central Illinois where study patients were likely to seek care. We validated readmissions and emergency department visits via patient/proxy telephone interviews that occurred 6 months after index hospital discharge. Interviewers were blind to intervention assignment. We evaluated the adequacy of the blind and asked interviewers to guess the patient's intervention assignment.
Another secondary outcome was the proportion of patients who experienced an adverse event related to medical management within 1 month after discharge. For adverse event ascertainment, we employed the process of Forster et al.1, 2 Within 20 to 40 days after discharge, an internal medicine physician performed telephone interviews with the patient or proxy. The interviewer recorded symptoms, drug information, other treatment, hospital readmissions, and emergency department visits. Another physician compiled case summaries from interview data and information abstracted from the electronic medical record, including dictated discharge summaries from the index hospitalization and postdischarge emergency department visits, diagnostic test results, and readmission reports. Two additional internal medicine physicians adjudicated each case summary separately. We counted adverse events only when adjudicators agreed that medical management probably or definitely caused the event. The initial rating by each adjudicator revealed moderate‐to‐good agreement (Kappa = 0.52).30 When initial adjudications were discordant, then adjudicators met and resolved all discrepancies. The adjudicators also scored the severity of the adverse event. The severity scale options were serious laboratory abnormality only, 1 day of symptoms, several days of symptoms, nonpermanent disability, permanent disability, or death. The adjudicators also scored the adverse event as preventable (yes/no), ameliorable (yes/no), and recorded system problems associated with preventable and ameliorable adverse events.1 For adverse drug events, the adjudicators recorded preventability categories defined by previous investigators.31 We designed the adverse event outcome ascertainment as a blinded process. We evaluated the success of the blind and asked adjudicators to guess the patient's intervention assignment.
Sample Size
The sample size analysis employed several assumptions regarding the proportion of readmitted patients. The estimated readmission rate after usual care was 37%.24, 3236 The minimum clinically relevant difference in readmission rates was 13%, an empirical boundary for quantitative significance.37 Estimates for intracluster correlation were not available when we designed the trial. We projected intracluster correlations with low, medium, and high values. The cluster number and size were selected to maintain test significance level, 1‐sided alpha, <0.05 and power >80%. The sample size assumed no interim analysis. The initial sample size estimates were 11 physician clusters per intervention with 25 patients per cluster. During the first 2 months of patient recruitment, we observed that we could not consistently achieve clusters with 25 patients. We recalculated the sample size. Using the same assumptions, we found we could achieve similar test significance and power with 35 physician clusters per intervention and 9 patients per cluster. The sample size calculator was nQuery (Statistical Solutions, Saugus, MA).
Statistical Methods
Analyses were performed with SPSS PC (Version 15.0.1; SPSS Inc, Chicago, IL). Using descriptive statistics, we reported baseline variables as means and standard deviations (SD) for interval variables, and percentages for categorical variables. For outcome variables, we utilized the principle of intention‐to‐treat and assumed patient exposure to the intervention randomly assigned to their discharging physician. We inspected scatter plots and correlations for all variables to test assumptions regarding normal distribution, homogeneity of variance, and linearity of relationships between independent and dependent variables. When assumptions failed, we stratified variables (median or thirds) or performed transformations to satisfy assumptions. For patient‐level outcome variables, we calculated intracluster correlation coefficients. The assessment of the blind was unaffected by the cluster assumption so we used the chi‐square procedure. For analysis of time to event, we used Kaplan‐Meier plots.
The primary hypothesis was a significant decrease in the primary readmission outcome for patients assigned to discharge software. We tested the primary hypothesis with generalized estimating equations that corrected for clustering by hospital physician and adjusted for covariates that predicted readmission. The intervention variable was discharge software versus usual care handwritten discharge. We reported parameter estimates of the intervention variable coefficient and Wald 95% confidence interval (95% CI) with and without correction for cluster. For the secondary, patient‐level outcomes, we performed similar analyses with generalized estimating equations that corrected for clustering by hospital physician.
During covariate analysis, we screened all baseline variables for their correlation with readmission. The variable with the highest correlation and P value <0.05 entered initially in the general estimating equation. After initial variable entry, we evaluated subsequent variables with partial correlations that controlled for variables entered previously. At each iterative step, we entered into the model the variable with the highest partial correlation and P value <0.05.
In exploratory analyses, we examined intervention group differences within strata defined by covariates that predicted readmission. We used generalized estimating equations and adjusted for the other covariates that predicted readmission.
Results
We screened 127 physicians who were general internal medicine hospital physicians. Seventy physicians consented and received random allocation to discharge software or usual care. The physician characteristics appear in Table 1. Most of the hospital physicians were interns in the first year of postgraduate training (58.6%; 41/70). We excluded 57 physicians for reasons shown in the trial flow diagram (Figure 1). The most common reason for hospital physician exclusion applied to resident physicians in their last months of training before graduation or emergency department residents temporarily assigned to internal medicine training. We approached 6,884 patients during their index hospitalization. After excluding 6,253 ineligible patients, we enrolled and followed 631 patients who received the discharge intervention (Figure 1). During 6 months of follow‐up, a small proportion of patients died (3%; 20/631). Hospital records were available for deceased patients and they were included in the analysis. A small proportion (6%; 41/631) of patients withdrew consent or left the trial for other reasons during 6 months. There was no differential dropout between the interventions. Protocol deviations were rare (0.5%; 3/631). Three patients erroneously received usual care discharge from physicians assigned to discharge software. All 631 patients were included in the intention‐to‐treat analysis. The baseline characteristics of the randomly‐assigned hospital physicians and their patients are in Table 1.
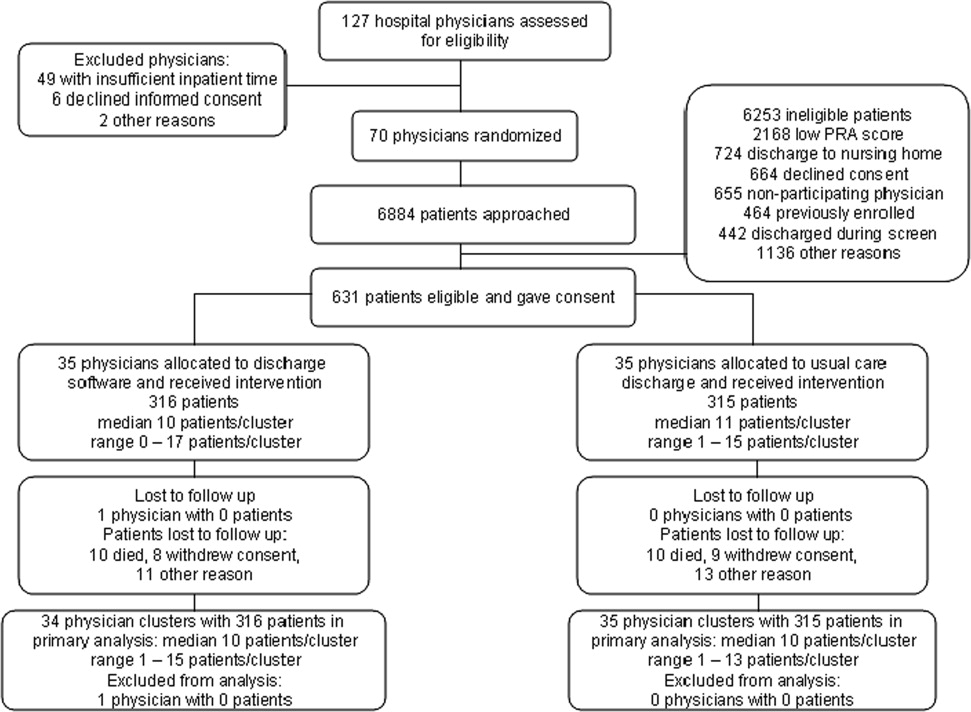
Discharge Software | Usual Care | |
---|---|---|
| ||
Hospital physician characteristics, n (%) | (n = 35) | (n = 35) |
Postgraduate year 1 | 18 (51.4) | 23 (65.7) |
Postgraduate years 2‐4 | 10 (28.6) | 7 (20.0) |
Attending physician | 7 (20.0) | 5 (14.3) |
Patient characteristics | (n = 316) | (n = 315) |
Gender, female, n (%) | 180 (57.0) | 168 (53.3) |
Age, years, n (%) | ||
18‐44 | 68 (21.5) | 95 (30.2) |
45‐54 | 79 (25.0) | 76 (24.1) |
55‐64 | 86 (27.2) | 74 (23.5) |
65‐98 | 83 (26.3) | 70 (22.2) |
Race, n (%) | ||
Caucasian | 239 (75.6) | 229 (72.7) |
Black | 72 (22.8) | 85 (27.0) |
Other | 5 (1.6) | 1 (0.3) |
Self‐rated health status, n (%) | ||
Poor | 82 (25.9) | 108 (34.3) |
Fair | 169 (53.5) | 147 (46.7) |
Good | 54 (17.1) | 46 (14.6) |
Very good | 10 (3.2) | 11 (3.5) |
Excellent | 1 (0.3) | 3 (1.0) |
Diabetes mellitus, n (%) | 172 (54.4) | 177 (56.2) |
Chronic obstructive pulmonary disease, n (%) | ||
None | 259 (82.0) | 257 (81.6) |
Without oral steroid or home oxygen | 28 (8.9) | 26 (8.3) |
With chronic oral steroid | 10 (3.2) | 8 (2.5) |
With home oxygen oral steroid | 19 (6.0) | 24 (7.6) |
Coronary heart disease, n (%) | 133 (42.1) | 120 (38.1) |
Heart failure, n (%) | 80 (25.3) | 67 (21.3) |
Informal caregiver available, yes, n (%) | 313 (99.1) | 313 (99.4) |
Taking loop diuretic, n (%) | 110 (34.8) | 88 (27.9) |
Physical functioning from SF‐36, n (%) | ||
Lowest third | 128 (40.5) | 121 (38.4) |
Upper two‐thirds | 188 (59.5) | 194 (61.6) |
Mental health from SF‐36, n (%) | ||
Lowest third | 113 (35.8) | 117 (37.1)* |
Upper two‐thirds | 203 (64.2) | 197 (62.5)* |
Hospital admissions during year prior to index admission, n (%) | ||
0 or 1 | 247 (78.2) | 224 (71.1) |
2 or more | 69 (21.8) | 91 (28.9) |
Emergency department visits during 6 months before index admission, n (%) | ||
0 or 1 | 194 (61.4) | 168 (53.3) |
2 or more | 122 (38.6) | 147 (46.7) |
Outpatient doctor or clinic visits during year prior to index admission | ||
0 to 4 | 97 (30.7%) | 77 (24.4%) |
5 to 8 | 68 (21.5%) | 81 (25.7%) |
9 to 12 | 82 (25.9%) | 84 (26.7%) |
13 or more | 69 (21.8%) | 73 (23.2%) |
Insurance or payor | ||
Medicare, age less than 65 years | 18 (5.7%) | 13 (4.1%) |
Medicare, age 65 years and older | 56 (17.7%) | 40 (12.7%) |
Medicaid, age less than 65 years | 98 (31.0%) | 130 (41.3%) |
Medicaid, age 65 years and older | 17 (5.4%) | 20 (6.3%) |
Commercial or veteran | 85 (26.9%) | 61 (19.4%) |
Self‐pay | 42 (13.3%) | 51 (16.2%) |
Religious participation | ||
Never | 159 (50.3%) | 164 (52.1%) |
1‐24 times per year | 55 (17.4%) | 51 (16.2%) |
1‐7 times per week | 102 (32.3%) | 100 (31.7%) |
Volunteer activity, 1 or more hour/month | 31 (9.8%) | 39 (12.4%) |
Employment status | ||
Not working | 229 (72.5%) | 233 (74.4%)* |
Part‐time (<37.5 hours/week) | 30 (9.5%) | 25 (8.0%)* |
Full‐time (at least 37.5 hour/week) | 57 (18.0%) | 55 (17.6%)* |
Number of discharge medications, mean (SD) | 10.5 (4.8) | 9.9 (5.1) |
Severity of illness, mean (SD) | 1.8 (1.2) | 1.6 (1.3) |
Charlson‐Deyo comorbidity, mean (SD) | 1.7 (1.4) | 1.6 (1.9) |
Index hospital length of stay, days, mean (SD) | 3.9 (3.5) | 3.5 (3.5) |
Blood urea nitrogen, mean (SD) | 17.9 (12.9) | 19.1 (12.9) |
Probability of repeat admission, Pra, mean (SD) | 0.486 (0.072) | 0.495 (0.076) |
We asked outpatient physicians about their receipt of discharge communication from hospital physicians. The text of the question was, How soon after discharge did you receive any information (in any form) relating to this patient's hospital admission and discharge plans? We mailed the question 10 days after discharge to outpatient physicians designated by patients enrolled in the study. Among patients in the discharge software group, 75.0% (237/316) of their outpatient physicians responded to the question. The response rate was 80.6% (254/315) from physicians who followed patients in the usual care group. Respondents from the discharge software group said within 1‐2 days or within a week for 56.0% (177/316) of patients. Respondents from the usual care group said within 1‐2 days or within a week for 57.1% (180/315) of patients. The difference between the intervention groups, 1.1% (95% CI, 9.2% to 6.9%), was not significant.
The primary, prespecified, outcome of the study was the proportion of patients with at least 1 readmission to the hospital. After intervention with discharge software versus usual care, there was no significant difference in readmission rates (Table 2) or time to first readmission (Figure 2). We screened all baseline variables in Table 1 and sought predictors of readmission to employ in adjusted models. For example, we evaluated physician level of training because we wondered if experience or seniority affected readmission when hospital physicians used the discharge software or usual care discharge. The candidate variable, physician level of training, did not correlate with readmission (rho = 0.066; P = 0.100), so it was dropped from subsequent analyses. After screening all variables in Table 1, we found 4 independent predictors of readmission: previous hospitalizations, previous emergency department visits, heart failure, and physical function. Generalized estimating equations for readmission that adjusted for predictor variables confirmed a negligible parameter estimate for the discharge intervention variable coefficient (Table 2).
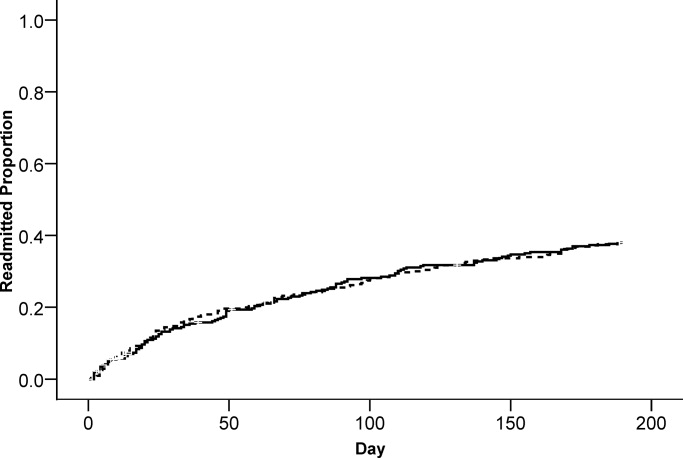
Outcome | Discharge Software, n (%) | Usual Care, n (%) | Parameter Estimate Without Cluster Correction Intervention Coefficient (95% CI) | P Value | Parameter Estimate With Cluster Correction Intervention Coefficient (95% CI) | P Value |
---|---|---|---|---|---|---|
| ||||||
Readmitted within 6 months | 117 (37.0%) | 119 (37.8%) | 0.005* (0.076, 0.067) | 0.897 | 0.005* (0.074, 0.065) | 0.894 |
Emergency department visit within 6 months | 112 (35.4%) | 128 (40.6%) | 0.052 (0.128, 0.024) | 0.179 | 0.052 (0.115, 0.011) | 0.108 |
Adverse event within 1 month | 23 (7.3%) | 23 (7.3%) | 0.003 (0.037, 0.043) | 0.886 | 0.003 (0.037, 0.043) | 0.884 |
We evaluated emergency department visits that were unrelated to readmission as secondary, prespecified, outcomes. The results were similar to readmission results. While the proportion of patients with at least 1 emergency department visit was lower for the discharge software intervention, the difference with usual care was not significant (Table 2). There was no significant difference between interventions for time to first emergency department visit (Figure 3).
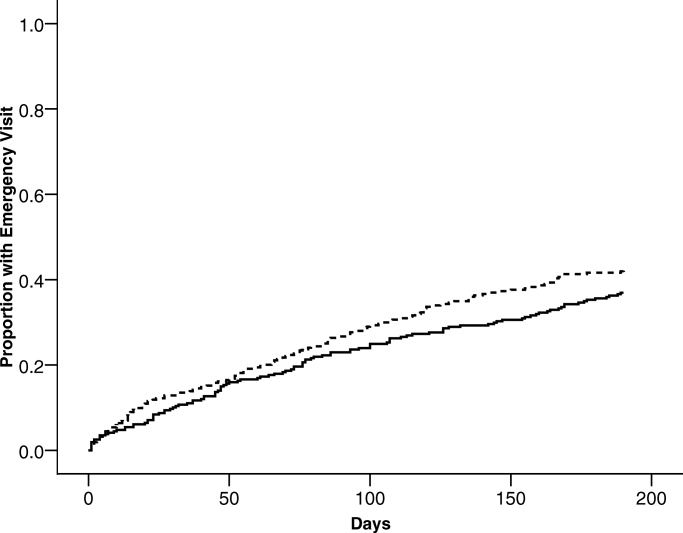
Postdischarge adverse events were secondary, prespecified, outcomes. Data for adverse event adjudication were available for 98% (309/316) of discharge software patients and 97% (307/315) of usual care patients. Within 1 month after discharge, 46 patients had adverse events probably or definitely related to medical management. Two patients had 2 events and 1 patient had 3 events. For analysis, we randomly selected 1 event per patient. When comparing patients assigned to discharge software versus usual care, there were no differences in adverse events related to medical management (Table 2). Most of the events were possible adverse drug events (74%; 34/46). The adverse event severity was several days of symptoms or nonpermanent disability for 76% (35/46) of the adverse events. Adjudicators rated 26% (12/46) of the adverse events as preventable and 46% (21/46) as ameliorable. The absolute numbers of events were small. There were no differences between discharge software and usual care patients within adverse event strata defined by type, severity, preventable, or ameliorable (Table 3). For most of the patients with adverse events, the adjudicators could not identify a system problem or preventability category (Table 3). When a deficiency was evident, there was no pattern to suggest a significant difference between discharge software patients versus usual care patients.
Discharge Software (n) | Usual Care Discharge (n) | |
---|---|---|
| ||
At least 1 adverse event | 23 | 23 |
Preventable adverse event | 7 | 5 |
Ameliorable adverse event | 9 | 12 |
Adverse event severity | ||
Serious laboratory abnormality only or 1 day of symptoms | 5 | 5 |
Several days of symptoms or nonpermanent disability | 18 | 17 |
Permanent disability or death | 0 | 1 |
Adverse event by type | ||
Possible adverse drug event | 17 | 17 |
Procedure‐related injury | 2 | 1 |
Therapeutic error | 4 | 4 |
Diagnostic error | 0 | 1 |
System problems associated with preventable or ameliorable adverse events | ||
Inadequate patient education regarding the medical condition or its treatment | 0 | 1 |
Poor communication between patient and physician | 2 | 1 |
Poor communication between hospital and community physicians | 0 | 0 |
Inadequate monitoring of the patient's illness after discharge | 0 | 6 |
Inadequate monitoring of the patient's treatment after discharge | 2 | 6 |
No emergency contact number given to patient to call about problems | 0 | 0 |
Patient with problems getting prescribed medications immediately | 1 | 0 |
Inadequate home services | 0 | 0 |
Delayed follow‐up care | 0 | 3 |
Premature hospital discharge | 1 | 2 |
Adverse drug event (ADE) preventability categories | ||
Drug involved in the ADE inappropriate for the clinical condition | 2 | 4 |
Dose, route, or frequency inappropriate for age, weight, creatinine clearance, or disease | 1 | 2 |
Failure to obtain required lab tests and/or drug levels | 1 | 2 |
Prior history of an adverse event or allergy to the drug | 1 | 2 |
Drug‐drug interaction involved in the ADE | 2 | 0 |
Toxic serum drug level documented | 0 | 0 |
Noncompliance involved in the ADE | 0 | 1 |
When we designed the trial, we assumed variance in outcomes measured at the patient level. We predicted some variance attributable to clustering by hospital physician. After the trial, we calculated the intracluster correlation coefficients for readmissions, emergency department visits, and adverse events. For all of these outcomes, the intracluster correlation coefficients were negligible. We also evaluated generalized estimating equations with and without correction for hospital physician cluster. We confirmed the negligible cluster effect on confidence intervals for intervention coefficients (Table 2).
We performed an exploratory stratified analysis. We evaluated the intervention effect on readmission within subgroups defined by covariates that predicted readmission (Table 4). When the intervention groups were compared within baseline categories of previous hospitalizations, previous emergency department visits, heart failure, and physical functioning, there was a consistent pattern with no differential effect by intervention assignment. None of the intervention coefficients were statistically significant (Table 4).
Subgroup | Discharge Software Readmitted n/n (%) | Usual Care Readmitted n/n (%) | Adjusted Parameter Estimate Intervention Coefficient (95% CI) |
---|---|---|---|
| |||
Hospital admissions during year prior to index admission | |||
0 or 1 | 77/247 (31.2) | 73/224 (32.6) | 0.025 (0.095, 0.045)* |
2 or more | 40/69 (58.0) | 46/91 (50.5) | 0.059 (0.090, 0.208)* |
Emergency department visits during 6 months before index admission | |||
0 or 1 | 64/194 (33.0) | 45/168 (26.8) | 0.033 (0.047, 0.113) |
2 or more | 53/122 (43.4) | 74/147 (50.3) | 0.071 (0.188, 0.046) |
Heart failure | |||
Present | 40/80 (50.0) | 36/67 (53.7) | 0.024 (0.224, 0.177) |
Absent | 77/236 (32.6) | 83/248 (33.5) | 0.000 (0.076, 0.075) |
Physical functioning from SF‐36 | |||
Lowest third | 55/128 (43.0) | 59/121 (48.8) | 0.032 (0.161, 0.096) |
Upper two‐thirds | 62/188 (33.0) | 60/194 (30.9) | 0.012 (0.071, 0.095) |
Assessment of the Success of the Blind
We evaluated the adequacy of the blind for outcome assessors who interviewed patients or adjudicated adverse events. The guesses of outcomes assessors were unrelated to true intervention assignment (all P values >0.097). We interpreted the blind as adequate for outcome assessors who recorded readmissions, emergency department visits, and adverse events.
Discussion
We performed a cluster‐randomized clinical trial to measure the effects of discharge software versus usual care handwritten discharge. The discharge software with CPOE implemented elements of high‐quality discharge planning and communication endorsed by the National Quality Forum and systematic reviews.6, 38 Despite theoretical benefits, our discharge software intervention did not reduce readmissions or emergency department visits. What were potential explanations for our results? We assumed an association between postdischarge adverse events and readmissions or emergency department visits.1 Our failure to reduce adverse events might explain the failure to reduce readmissions or emergency department visits. Another potential explanation was related to adverse drug events. Other investigators showed most postdischarge adverse events were adverse drug events and our data confirmed previous studies.1, 2 Medication reconciliation at discharge was a potential mechanism for adverse drug event reduction.14 Medication reconciliation was the standard at the study hospital, so it was unethical to deny reconciliation to patients assigned to either intervention.39 Required medication reconciliation in both groups, by its known effect on preventable adverse drug events, might have reduced the event rates in both groups.14 This possibility is supported by the low rate of adverse events observed in our study compared with other studies.1 We speculate that the low background rate of adverse events at the study hospital may have minimized events in both the discharge software and usual care groups and prevented detection of software benefits, if present.39
One limitation of our study may have been the discharge software. The automated decision support in our software lacked features that might have improved outcomes. For example, the software did not generate a list of diagnostic test results that were pending at the time of discharge. Our software relied on prompts to the physician user that did not specify which tests were pending. The software did not perform error checks on the discharge orders to warn physicians about drug‐drug interactions, therapeutic duplications, or missing items (eg, immunizations, drugs, education). The absence of these software enhancements made our discharge process vulnerable to the lapses and slips of the physician user. Whether or not such enhancements affect clinically relevant outcomes remains a testable hypothesis for future studies.
Another limitation of our study was the outpatient physician response. Discharge software did not increase the proportion of outpatient physicians who said they received communication within 7 days after hospital discharge. Our intervention addressed the sending partner but not the receiving partner in the communication dyad. Our discharge software was not designed to change information flow within the outpatient physician office. We do not know if discharge communication arrived and remained unnoticed until the patient called or visited the outpatient clinic. Future studies of discharge communication should consider a closed loop design to assure receipt and comprehension.
When we designed our study, we expected at least some variance between patient clusters attributable to the physician who performed the discharge. Our analysis of intracluster correlation revealed negligible variance. We speculate the highly‐standardized discharge process implemented by discharge software and usual care at our hospital resulted in minimal variance. Future studies of discharge interventions may consider designs that avoid cluster randomization.
In conclusion, a discharge software application of CPOE did not affect readmissions, emergency department visits, or adverse events after discharge.
Acknowledgements
The authors thank Howard S. Cohen, MD, for his review of the trial protocol and the manuscript.
Adverse events occur to patients after their discharge from acute care hospitals.1, 2 Most of these injuries are adverse drug events, procedure‐related events, nosocomial infections, or falls.1 Postdischarge adverse events are associated with several days of symptoms, nonpermanent disability, emergency department visits, or hospital readmission.1, 3 When adverse events are preventable or ameliorable, the most common root cause is poor communication between hospital personnel and either the patient or the outpatient primary care physician.1 In addition, there may be deficits in discharge processes related to assessment and communication of unresolved problems.1 Systematic reviews have shown that discharge communication is an inefficient and error‐prone process.46
One potential solution to poor discharge communication is health information technology.7 An example of technology is discharge software with a computerized physician order entry (CPOE) system. By definition, a CPOE system is a computer‐based system that automates direct entry of orders by physicians and ensures standardized, legible, and complete orders.8 The benefits of CPOE have been tested in other inpatient settings.8, 9 It is logical to consider software applications with CPOE for discharge interventions.7
Several mechanisms explain the potential benefit of discharge software with CPOE.7 Applications with CPOE decrease medication errors.8, 10 Software with decision support could prompt physicians to enter posthospitalization appointment dates and orders for preventive services.11, 12 Discharge software could facilitate medication reconciliation and generate patient instructions and information.4, 1315 The potential benefits of discharge software with CPOE provide a rationale for clinical trials to measure benefits.
Previous studies addressed discharge applications of health information technology. Observational studies recorded outcomes such as physician satisfaction.16, 17 Prior randomized clinical trials measured quality and timeliness of discharge summaries.18 However, these previous trials did not assess clinically relevant outcomes like readmissions, emergency department visits, or adverse events. We performed a cluster‐randomized trial to assess the value of a discharge software application of CPOE. The rationale for our clustered design complied with recommendations from a systematic review of discharge interventions.5 Our objective was to assess the benefit of discharge software with CPOE when used to discharge patients at high risk for repeat admission. After the intervention, we compared the rates of hospital readmission, emergency department visits, and postdischarge adverse events due to medical management.
Methods
The trial design was a cluster randomized, controlled trial with blinded outcome assessment. Follow‐up occurred until 6 months after discharge from index hospitalization at a 730‐bed, tertiary care, teaching hospital in central Illinois. The Peoria Institutional Review Board approved the protocol for human research.
Participants
The cluster definition was the hospital physician. Patients discharged by the physician comprised the cluster. Hospital physicians and patients were enrolled between November 2004 and January 2007. Internal medicine resident or attending physicians were eligible. We excluded hospital physicians if their assignments to inpatient duties were less than 2 months during the 27‐month enrollment period. The rationale for the physician exclusion was a consequence of the patient enrollment rate of 3 to 5 patients per physician per month. Physicians with brief assignments could not achieve the goal of 9 or more patients per cluster. After physicians gave informed consent to screen their patients, trained research coordinators applied inclusion and exclusion criteria and obtained informed consent from patients. Research personnel identified all consecutive, unique, adult inpatients who were discharged to home. Patient inclusion required a probability of repeat admission (Pra) score 0.40.19, 20 The Pra score came from a logistic model of age, gender, prior hospitalizations, prior doctor visits, self‐rated health status, presence of informal caregiver in the home, and comorbid coronary heart disease and diabetes mellitus. Research coordinators calculated the Pra within 2 days before discharge from the index hospitalization. Other details about exclusion criteria have been published.21 If a patient's outpatient primary care physician treated the patient during the index hospitalization, then there was no perceived barrier in physician‐to‐physician communication and we excluded the patient.
Intervention
The research intervention was a CPOE software application that facilitated communication at the time of hospital discharge to patients, retail pharmacists, and community physicians. Details about the discharge software appeared in a previous publication.7 Software features included required fields, pick lists, standard drug doses, alerts, reminders, and online reference information. The software prompted the discharging physician to enter pending tests and order tests after discharge. Hospital physicians used the software on the day of discharge and automatically generated 4 discharge documents. The first document was a personalized letter to the outpatient physician with discharge diagnoses, reconciled medication list, diet and activity instructions, patient education materials provided, and follow‐up appointments and studies. Second, the software printed legible prescriptions along with specific information for the dispensing pharmacist about changes and deletions in the patient's previous regimen. Third, the software created patient instructions with addresses and telephone numbers for follow‐up appointments and tests. Fourth, the software printed a legible discharge order including all of the aforementioned information.
The control intervention was the usual care discharge process as described previously.7 Hospital physicians and ward nurses completed handwritten discharge forms on the day of discharge. The forms contained blanks for discharge diagnoses, discharge medications, medication instructions, postdischarge activities and restrictions, postdischarge diet, postdischarge diagnostic and therapeutic interventions, and appointments. Patients received handwritten copies of the forms, 1 page of which also included medication instructions and prescriptions. A previous publication gave details about the standard care available to all patients regardless of intervention.7
Randomization
The unit of randomization was the hospital physician who performed the discharge process. Random allocation was to discharge software or usual care discharge process. The randomization ratio was 1:1, the block size was 2, and there was no stratification or matching. There was concealed allocation and details are available from the investigators. Hospital physicians subsequently used their randomly assigned process when discharging their patients who enrolled in the study. After random allocation, it was not possible to conceal the test or control intervention from physicians or their patients. Likewise, it was not possible to conceal the outcome ascertainment, including readmission, from the hospital physicians.
All hospital physicians received training on the usual care discharge process. Physicians assigned to discharge software completed additional training via multimedia demonstration with 1‐on‐1 coaching as needed. Physicians assigned to usual care did not receive training on the discharge software and were blocked from using the software. After informed consent, patients were passive recipients of the research intervention performed by their discharging physician. Patients received the research intervention on the day of discharge from the index hospitalization.
The baseline assessment of patient characteristics occurred during the index hospitalization. Trained data abstractors recorded patient demographic data plus variables to calculate the Pra score for probability for repeat admission. We recorded additional variables because of their possible association with readmission.15, 2229 Data came from the patient or proxy for physical functioning and mental health (SF‐36, Version 2; Medical Outcomes Trust, Boston, MA). Other data for predictor variables came from interviews or hospital records.
Outcome Assessment
The primary study outcome was the proportion of patients readmitted at least once within 6 months after the index hospitalization. Readmission was for any reason and included observation and full admission status. Secondary outcomes were emergency department visits that did not result in hospital admission. Outcome assessment occurred at the patient level. We obtained data for readmissions and emergency department visits from 6 hospitals in central Illinois where study patients were likely to seek care. We validated readmissions and emergency department visits via patient/proxy telephone interviews that occurred 6 months after index hospital discharge. Interviewers were blind to intervention assignment. We evaluated the adequacy of the blind and asked interviewers to guess the patient's intervention assignment.
Another secondary outcome was the proportion of patients who experienced an adverse event related to medical management within 1 month after discharge. For adverse event ascertainment, we employed the process of Forster et al.1, 2 Within 20 to 40 days after discharge, an internal medicine physician performed telephone interviews with the patient or proxy. The interviewer recorded symptoms, drug information, other treatment, hospital readmissions, and emergency department visits. Another physician compiled case summaries from interview data and information abstracted from the electronic medical record, including dictated discharge summaries from the index hospitalization and postdischarge emergency department visits, diagnostic test results, and readmission reports. Two additional internal medicine physicians adjudicated each case summary separately. We counted adverse events only when adjudicators agreed that medical management probably or definitely caused the event. The initial rating by each adjudicator revealed moderate‐to‐good agreement (Kappa = 0.52).30 When initial adjudications were discordant, then adjudicators met and resolved all discrepancies. The adjudicators also scored the severity of the adverse event. The severity scale options were serious laboratory abnormality only, 1 day of symptoms, several days of symptoms, nonpermanent disability, permanent disability, or death. The adjudicators also scored the adverse event as preventable (yes/no), ameliorable (yes/no), and recorded system problems associated with preventable and ameliorable adverse events.1 For adverse drug events, the adjudicators recorded preventability categories defined by previous investigators.31 We designed the adverse event outcome ascertainment as a blinded process. We evaluated the success of the blind and asked adjudicators to guess the patient's intervention assignment.
Sample Size
The sample size analysis employed several assumptions regarding the proportion of readmitted patients. The estimated readmission rate after usual care was 37%.24, 3236 The minimum clinically relevant difference in readmission rates was 13%, an empirical boundary for quantitative significance.37 Estimates for intracluster correlation were not available when we designed the trial. We projected intracluster correlations with low, medium, and high values. The cluster number and size were selected to maintain test significance level, 1‐sided alpha, <0.05 and power >80%. The sample size assumed no interim analysis. The initial sample size estimates were 11 physician clusters per intervention with 25 patients per cluster. During the first 2 months of patient recruitment, we observed that we could not consistently achieve clusters with 25 patients. We recalculated the sample size. Using the same assumptions, we found we could achieve similar test significance and power with 35 physician clusters per intervention and 9 patients per cluster. The sample size calculator was nQuery (Statistical Solutions, Saugus, MA).
Statistical Methods
Analyses were performed with SPSS PC (Version 15.0.1; SPSS Inc, Chicago, IL). Using descriptive statistics, we reported baseline variables as means and standard deviations (SD) for interval variables, and percentages for categorical variables. For outcome variables, we utilized the principle of intention‐to‐treat and assumed patient exposure to the intervention randomly assigned to their discharging physician. We inspected scatter plots and correlations for all variables to test assumptions regarding normal distribution, homogeneity of variance, and linearity of relationships between independent and dependent variables. When assumptions failed, we stratified variables (median or thirds) or performed transformations to satisfy assumptions. For patient‐level outcome variables, we calculated intracluster correlation coefficients. The assessment of the blind was unaffected by the cluster assumption so we used the chi‐square procedure. For analysis of time to event, we used Kaplan‐Meier plots.
The primary hypothesis was a significant decrease in the primary readmission outcome for patients assigned to discharge software. We tested the primary hypothesis with generalized estimating equations that corrected for clustering by hospital physician and adjusted for covariates that predicted readmission. The intervention variable was discharge software versus usual care handwritten discharge. We reported parameter estimates of the intervention variable coefficient and Wald 95% confidence interval (95% CI) with and without correction for cluster. For the secondary, patient‐level outcomes, we performed similar analyses with generalized estimating equations that corrected for clustering by hospital physician.
During covariate analysis, we screened all baseline variables for their correlation with readmission. The variable with the highest correlation and P value <0.05 entered initially in the general estimating equation. After initial variable entry, we evaluated subsequent variables with partial correlations that controlled for variables entered previously. At each iterative step, we entered into the model the variable with the highest partial correlation and P value <0.05.
In exploratory analyses, we examined intervention group differences within strata defined by covariates that predicted readmission. We used generalized estimating equations and adjusted for the other covariates that predicted readmission.
Results
We screened 127 physicians who were general internal medicine hospital physicians. Seventy physicians consented and received random allocation to discharge software or usual care. The physician characteristics appear in Table 1. Most of the hospital physicians were interns in the first year of postgraduate training (58.6%; 41/70). We excluded 57 physicians for reasons shown in the trial flow diagram (Figure 1). The most common reason for hospital physician exclusion applied to resident physicians in their last months of training before graduation or emergency department residents temporarily assigned to internal medicine training. We approached 6,884 patients during their index hospitalization. After excluding 6,253 ineligible patients, we enrolled and followed 631 patients who received the discharge intervention (Figure 1). During 6 months of follow‐up, a small proportion of patients died (3%; 20/631). Hospital records were available for deceased patients and they were included in the analysis. A small proportion (6%; 41/631) of patients withdrew consent or left the trial for other reasons during 6 months. There was no differential dropout between the interventions. Protocol deviations were rare (0.5%; 3/631). Three patients erroneously received usual care discharge from physicians assigned to discharge software. All 631 patients were included in the intention‐to‐treat analysis. The baseline characteristics of the randomly‐assigned hospital physicians and their patients are in Table 1.
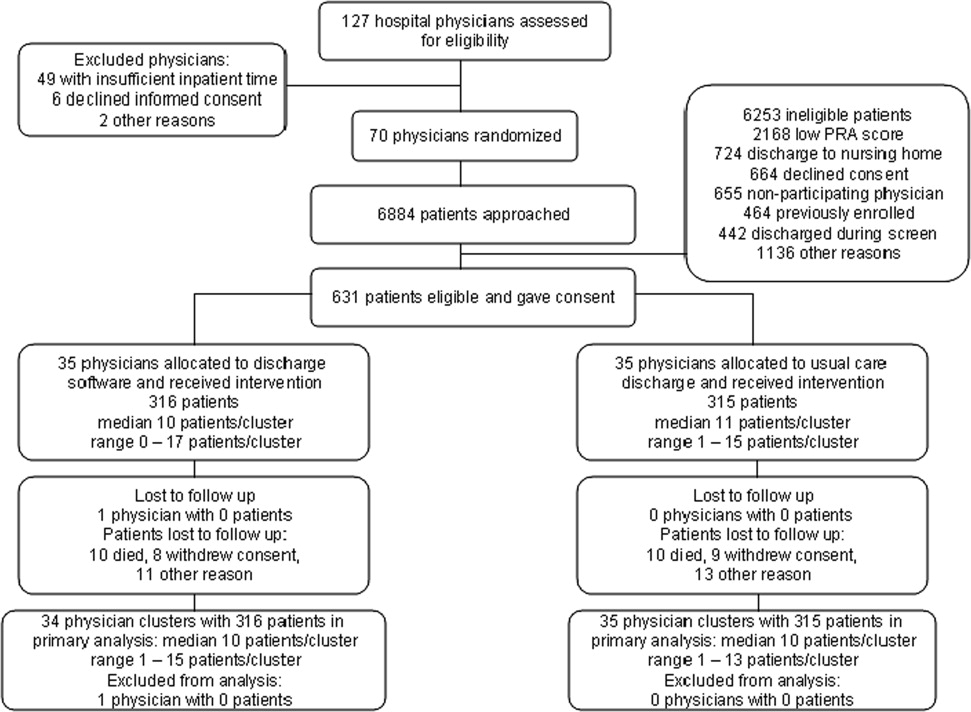
Discharge Software | Usual Care | |
---|---|---|
| ||
Hospital physician characteristics, n (%) | (n = 35) | (n = 35) |
Postgraduate year 1 | 18 (51.4) | 23 (65.7) |
Postgraduate years 2‐4 | 10 (28.6) | 7 (20.0) |
Attending physician | 7 (20.0) | 5 (14.3) |
Patient characteristics | (n = 316) | (n = 315) |
Gender, female, n (%) | 180 (57.0) | 168 (53.3) |
Age, years, n (%) | ||
18‐44 | 68 (21.5) | 95 (30.2) |
45‐54 | 79 (25.0) | 76 (24.1) |
55‐64 | 86 (27.2) | 74 (23.5) |
65‐98 | 83 (26.3) | 70 (22.2) |
Race, n (%) | ||
Caucasian | 239 (75.6) | 229 (72.7) |
Black | 72 (22.8) | 85 (27.0) |
Other | 5 (1.6) | 1 (0.3) |
Self‐rated health status, n (%) | ||
Poor | 82 (25.9) | 108 (34.3) |
Fair | 169 (53.5) | 147 (46.7) |
Good | 54 (17.1) | 46 (14.6) |
Very good | 10 (3.2) | 11 (3.5) |
Excellent | 1 (0.3) | 3 (1.0) |
Diabetes mellitus, n (%) | 172 (54.4) | 177 (56.2) |
Chronic obstructive pulmonary disease, n (%) | ||
None | 259 (82.0) | 257 (81.6) |
Without oral steroid or home oxygen | 28 (8.9) | 26 (8.3) |
With chronic oral steroid | 10 (3.2) | 8 (2.5) |
With home oxygen oral steroid | 19 (6.0) | 24 (7.6) |
Coronary heart disease, n (%) | 133 (42.1) | 120 (38.1) |
Heart failure, n (%) | 80 (25.3) | 67 (21.3) |
Informal caregiver available, yes, n (%) | 313 (99.1) | 313 (99.4) |
Taking loop diuretic, n (%) | 110 (34.8) | 88 (27.9) |
Physical functioning from SF‐36, n (%) | ||
Lowest third | 128 (40.5) | 121 (38.4) |
Upper two‐thirds | 188 (59.5) | 194 (61.6) |
Mental health from SF‐36, n (%) | ||
Lowest third | 113 (35.8) | 117 (37.1)* |
Upper two‐thirds | 203 (64.2) | 197 (62.5)* |
Hospital admissions during year prior to index admission, n (%) | ||
0 or 1 | 247 (78.2) | 224 (71.1) |
2 or more | 69 (21.8) | 91 (28.9) |
Emergency department visits during 6 months before index admission, n (%) | ||
0 or 1 | 194 (61.4) | 168 (53.3) |
2 or more | 122 (38.6) | 147 (46.7) |
Outpatient doctor or clinic visits during year prior to index admission | ||
0 to 4 | 97 (30.7%) | 77 (24.4%) |
5 to 8 | 68 (21.5%) | 81 (25.7%) |
9 to 12 | 82 (25.9%) | 84 (26.7%) |
13 or more | 69 (21.8%) | 73 (23.2%) |
Insurance or payor | ||
Medicare, age less than 65 years | 18 (5.7%) | 13 (4.1%) |
Medicare, age 65 years and older | 56 (17.7%) | 40 (12.7%) |
Medicaid, age less than 65 years | 98 (31.0%) | 130 (41.3%) |
Medicaid, age 65 years and older | 17 (5.4%) | 20 (6.3%) |
Commercial or veteran | 85 (26.9%) | 61 (19.4%) |
Self‐pay | 42 (13.3%) | 51 (16.2%) |
Religious participation | ||
Never | 159 (50.3%) | 164 (52.1%) |
1‐24 times per year | 55 (17.4%) | 51 (16.2%) |
1‐7 times per week | 102 (32.3%) | 100 (31.7%) |
Volunteer activity, 1 or more hour/month | 31 (9.8%) | 39 (12.4%) |
Employment status | ||
Not working | 229 (72.5%) | 233 (74.4%)* |
Part‐time (<37.5 hours/week) | 30 (9.5%) | 25 (8.0%)* |
Full‐time (at least 37.5 hour/week) | 57 (18.0%) | 55 (17.6%)* |
Number of discharge medications, mean (SD) | 10.5 (4.8) | 9.9 (5.1) |
Severity of illness, mean (SD) | 1.8 (1.2) | 1.6 (1.3) |
Charlson‐Deyo comorbidity, mean (SD) | 1.7 (1.4) | 1.6 (1.9) |
Index hospital length of stay, days, mean (SD) | 3.9 (3.5) | 3.5 (3.5) |
Blood urea nitrogen, mean (SD) | 17.9 (12.9) | 19.1 (12.9) |
Probability of repeat admission, Pra, mean (SD) | 0.486 (0.072) | 0.495 (0.076) |
We asked outpatient physicians about their receipt of discharge communication from hospital physicians. The text of the question was, How soon after discharge did you receive any information (in any form) relating to this patient's hospital admission and discharge plans? We mailed the question 10 days after discharge to outpatient physicians designated by patients enrolled in the study. Among patients in the discharge software group, 75.0% (237/316) of their outpatient physicians responded to the question. The response rate was 80.6% (254/315) from physicians who followed patients in the usual care group. Respondents from the discharge software group said within 1‐2 days or within a week for 56.0% (177/316) of patients. Respondents from the usual care group said within 1‐2 days or within a week for 57.1% (180/315) of patients. The difference between the intervention groups, 1.1% (95% CI, 9.2% to 6.9%), was not significant.
The primary, prespecified, outcome of the study was the proportion of patients with at least 1 readmission to the hospital. After intervention with discharge software versus usual care, there was no significant difference in readmission rates (Table 2) or time to first readmission (Figure 2). We screened all baseline variables in Table 1 and sought predictors of readmission to employ in adjusted models. For example, we evaluated physician level of training because we wondered if experience or seniority affected readmission when hospital physicians used the discharge software or usual care discharge. The candidate variable, physician level of training, did not correlate with readmission (rho = 0.066; P = 0.100), so it was dropped from subsequent analyses. After screening all variables in Table 1, we found 4 independent predictors of readmission: previous hospitalizations, previous emergency department visits, heart failure, and physical function. Generalized estimating equations for readmission that adjusted for predictor variables confirmed a negligible parameter estimate for the discharge intervention variable coefficient (Table 2).
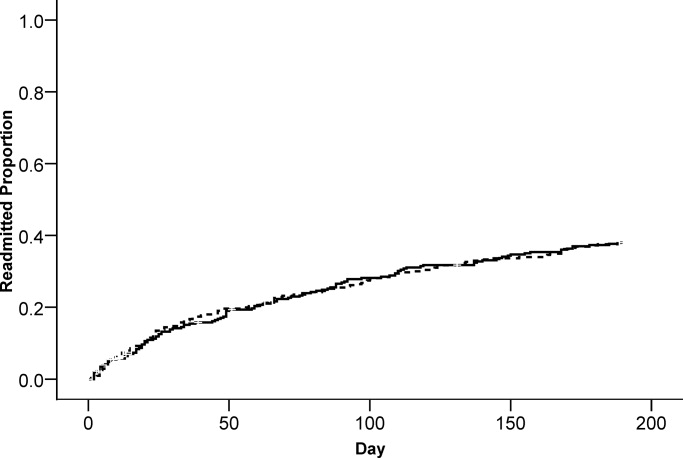
Outcome | Discharge Software, n (%) | Usual Care, n (%) | Parameter Estimate Without Cluster Correction Intervention Coefficient (95% CI) | P Value | Parameter Estimate With Cluster Correction Intervention Coefficient (95% CI) | P Value |
---|---|---|---|---|---|---|
| ||||||
Readmitted within 6 months | 117 (37.0%) | 119 (37.8%) | 0.005* (0.076, 0.067) | 0.897 | 0.005* (0.074, 0.065) | 0.894 |
Emergency department visit within 6 months | 112 (35.4%) | 128 (40.6%) | 0.052 (0.128, 0.024) | 0.179 | 0.052 (0.115, 0.011) | 0.108 |
Adverse event within 1 month | 23 (7.3%) | 23 (7.3%) | 0.003 (0.037, 0.043) | 0.886 | 0.003 (0.037, 0.043) | 0.884 |
We evaluated emergency department visits that were unrelated to readmission as secondary, prespecified, outcomes. The results were similar to readmission results. While the proportion of patients with at least 1 emergency department visit was lower for the discharge software intervention, the difference with usual care was not significant (Table 2). There was no significant difference between interventions for time to first emergency department visit (Figure 3).
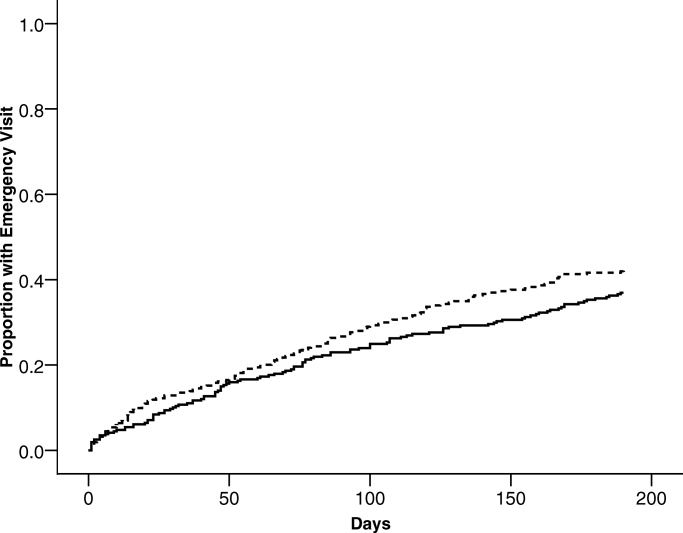
Postdischarge adverse events were secondary, prespecified, outcomes. Data for adverse event adjudication were available for 98% (309/316) of discharge software patients and 97% (307/315) of usual care patients. Within 1 month after discharge, 46 patients had adverse events probably or definitely related to medical management. Two patients had 2 events and 1 patient had 3 events. For analysis, we randomly selected 1 event per patient. When comparing patients assigned to discharge software versus usual care, there were no differences in adverse events related to medical management (Table 2). Most of the events were possible adverse drug events (74%; 34/46). The adverse event severity was several days of symptoms or nonpermanent disability for 76% (35/46) of the adverse events. Adjudicators rated 26% (12/46) of the adverse events as preventable and 46% (21/46) as ameliorable. The absolute numbers of events were small. There were no differences between discharge software and usual care patients within adverse event strata defined by type, severity, preventable, or ameliorable (Table 3). For most of the patients with adverse events, the adjudicators could not identify a system problem or preventability category (Table 3). When a deficiency was evident, there was no pattern to suggest a significant difference between discharge software patients versus usual care patients.
Discharge Software (n) | Usual Care Discharge (n) | |
---|---|---|
| ||
At least 1 adverse event | 23 | 23 |
Preventable adverse event | 7 | 5 |
Ameliorable adverse event | 9 | 12 |
Adverse event severity | ||
Serious laboratory abnormality only or 1 day of symptoms | 5 | 5 |
Several days of symptoms or nonpermanent disability | 18 | 17 |
Permanent disability or death | 0 | 1 |
Adverse event by type | ||
Possible adverse drug event | 17 | 17 |
Procedure‐related injury | 2 | 1 |
Therapeutic error | 4 | 4 |
Diagnostic error | 0 | 1 |
System problems associated with preventable or ameliorable adverse events | ||
Inadequate patient education regarding the medical condition or its treatment | 0 | 1 |
Poor communication between patient and physician | 2 | 1 |
Poor communication between hospital and community physicians | 0 | 0 |
Inadequate monitoring of the patient's illness after discharge | 0 | 6 |
Inadequate monitoring of the patient's treatment after discharge | 2 | 6 |
No emergency contact number given to patient to call about problems | 0 | 0 |
Patient with problems getting prescribed medications immediately | 1 | 0 |
Inadequate home services | 0 | 0 |
Delayed follow‐up care | 0 | 3 |
Premature hospital discharge | 1 | 2 |
Adverse drug event (ADE) preventability categories | ||
Drug involved in the ADE inappropriate for the clinical condition | 2 | 4 |
Dose, route, or frequency inappropriate for age, weight, creatinine clearance, or disease | 1 | 2 |
Failure to obtain required lab tests and/or drug levels | 1 | 2 |
Prior history of an adverse event or allergy to the drug | 1 | 2 |
Drug‐drug interaction involved in the ADE | 2 | 0 |
Toxic serum drug level documented | 0 | 0 |
Noncompliance involved in the ADE | 0 | 1 |
When we designed the trial, we assumed variance in outcomes measured at the patient level. We predicted some variance attributable to clustering by hospital physician. After the trial, we calculated the intracluster correlation coefficients for readmissions, emergency department visits, and adverse events. For all of these outcomes, the intracluster correlation coefficients were negligible. We also evaluated generalized estimating equations with and without correction for hospital physician cluster. We confirmed the negligible cluster effect on confidence intervals for intervention coefficients (Table 2).
We performed an exploratory stratified analysis. We evaluated the intervention effect on readmission within subgroups defined by covariates that predicted readmission (Table 4). When the intervention groups were compared within baseline categories of previous hospitalizations, previous emergency department visits, heart failure, and physical functioning, there was a consistent pattern with no differential effect by intervention assignment. None of the intervention coefficients were statistically significant (Table 4).
Subgroup | Discharge Software Readmitted n/n (%) | Usual Care Readmitted n/n (%) | Adjusted Parameter Estimate Intervention Coefficient (95% CI) |
---|---|---|---|
| |||
Hospital admissions during year prior to index admission | |||
0 or 1 | 77/247 (31.2) | 73/224 (32.6) | 0.025 (0.095, 0.045)* |
2 or more | 40/69 (58.0) | 46/91 (50.5) | 0.059 (0.090, 0.208)* |
Emergency department visits during 6 months before index admission | |||
0 or 1 | 64/194 (33.0) | 45/168 (26.8) | 0.033 (0.047, 0.113) |
2 or more | 53/122 (43.4) | 74/147 (50.3) | 0.071 (0.188, 0.046) |
Heart failure | |||
Present | 40/80 (50.0) | 36/67 (53.7) | 0.024 (0.224, 0.177) |
Absent | 77/236 (32.6) | 83/248 (33.5) | 0.000 (0.076, 0.075) |
Physical functioning from SF‐36 | |||
Lowest third | 55/128 (43.0) | 59/121 (48.8) | 0.032 (0.161, 0.096) |
Upper two‐thirds | 62/188 (33.0) | 60/194 (30.9) | 0.012 (0.071, 0.095) |
Assessment of the Success of the Blind
We evaluated the adequacy of the blind for outcome assessors who interviewed patients or adjudicated adverse events. The guesses of outcomes assessors were unrelated to true intervention assignment (all P values >0.097). We interpreted the blind as adequate for outcome assessors who recorded readmissions, emergency department visits, and adverse events.
Discussion
We performed a cluster‐randomized clinical trial to measure the effects of discharge software versus usual care handwritten discharge. The discharge software with CPOE implemented elements of high‐quality discharge planning and communication endorsed by the National Quality Forum and systematic reviews.6, 38 Despite theoretical benefits, our discharge software intervention did not reduce readmissions or emergency department visits. What were potential explanations for our results? We assumed an association between postdischarge adverse events and readmissions or emergency department visits.1 Our failure to reduce adverse events might explain the failure to reduce readmissions or emergency department visits. Another potential explanation was related to adverse drug events. Other investigators showed most postdischarge adverse events were adverse drug events and our data confirmed previous studies.1, 2 Medication reconciliation at discharge was a potential mechanism for adverse drug event reduction.14 Medication reconciliation was the standard at the study hospital, so it was unethical to deny reconciliation to patients assigned to either intervention.39 Required medication reconciliation in both groups, by its known effect on preventable adverse drug events, might have reduced the event rates in both groups.14 This possibility is supported by the low rate of adverse events observed in our study compared with other studies.1 We speculate that the low background rate of adverse events at the study hospital may have minimized events in both the discharge software and usual care groups and prevented detection of software benefits, if present.39
One limitation of our study may have been the discharge software. The automated decision support in our software lacked features that might have improved outcomes. For example, the software did not generate a list of diagnostic test results that were pending at the time of discharge. Our software relied on prompts to the physician user that did not specify which tests were pending. The software did not perform error checks on the discharge orders to warn physicians about drug‐drug interactions, therapeutic duplications, or missing items (eg, immunizations, drugs, education). The absence of these software enhancements made our discharge process vulnerable to the lapses and slips of the physician user. Whether or not such enhancements affect clinically relevant outcomes remains a testable hypothesis for future studies.
Another limitation of our study was the outpatient physician response. Discharge software did not increase the proportion of outpatient physicians who said they received communication within 7 days after hospital discharge. Our intervention addressed the sending partner but not the receiving partner in the communication dyad. Our discharge software was not designed to change information flow within the outpatient physician office. We do not know if discharge communication arrived and remained unnoticed until the patient called or visited the outpatient clinic. Future studies of discharge communication should consider a closed loop design to assure receipt and comprehension.
When we designed our study, we expected at least some variance between patient clusters attributable to the physician who performed the discharge. Our analysis of intracluster correlation revealed negligible variance. We speculate the highly‐standardized discharge process implemented by discharge software and usual care at our hospital resulted in minimal variance. Future studies of discharge interventions may consider designs that avoid cluster randomization.
In conclusion, a discharge software application of CPOE did not affect readmissions, emergency department visits, or adverse events after discharge.
Acknowledgements
The authors thank Howard S. Cohen, MD, for his review of the trial protocol and the manuscript.
- The incidence and severity of adverse events affecting patients after discharge from the hospital.Ann Intern Med.2003;138(3):161–167. , , , , .
- Adverse events among medical patients after discharge from hospital.CMAJ.2004;170(3):345–349. , , , et al.
- Frequency of new or worsening symptoms in the posthospitalization period.J Hosp Med.2007;2(2):58–68. , , , , .
- Written and verbal information versus verbal information only for patients being discharged from acute hospital settings to home.Cochrane Database Syst Rev.2003;(4):CD003716. , , .
- Discharge planning from hospital to home.Cochrane Database Syst Rev.2004;(1):CD000313. , , , .
- Deficits in communication and information transfer between hospital‐based and primary care physicians: implications for patient safety and continuity of care.JAMA.2007;297(8):831–841. , , , , , .
- Software design to facilitate information transfer at hospital discharge.Inform Prim Care.2006;14(2):109–119. , , .
- Effects of computerized physician order entry and clinical decision support systems on medication safety: a systematic review.Arch Intern Med.2003;163(12):1409–1416. , , .
- Computer physician order entry: benefits, costs, and issues.Ann Intern Med.2003;139(1):31–39. , .
- Systematic review: impact of health information technology on quality, efficiency, and costs of medical care.Ann Intern Med.2006;144(10):742–752. , , , et al.
- Compliance with post‐hospitalization follow‐up visits: rationing by inconvenience?Ethn Dis.1999;9(3):387–395. , , , , , .
- A computerized reminder system to increase the use of preventive care for hospitalized patients.N Engl J Med.2001;345(13):965–970. , , , , , .
- Evaluation of a new integrated discharge prescription form.Ann Pharmacother.2001;35(7‐8):953–958. , , , .
- Role of pharmacist counseling in preventing adverse drug events after hospitalization.Arch Intern Med.2006;166(5):565–571. , , , et al.
- Factors associated with unplanned hospital readmission among patients 65 years of age and older in a Medicare managed care plan.Am J Med.1999;107(1):13–17. , , , , , .
- Closing the loop of patient care—a clinical trial of a computerized discharge medication program.Proc Annu Symp Comput Appl Med Care.1994:841–845. , .
- A comprehensive inpatient discharge system.Proc AMIA Annu Fall Symp.1996:699–703. , , , .
- Agency for Healthcare Research and Quality. Making health care safer: a critical analysis of patient safety practices, subchapter 42.3. Discharge summaries and follow‐up. Available at: http://www.ahrq.gov/clinic/ptsafety/chap42b. htm#42.3. Accessed January 2009.
- Predictive validity of a questionnaire that identifies older persons at risk for hospital admission.J Am Geriatr Soc.1995;43(4):374–377. , , .
- Predictive validity of the Pra instrument among older recipients of managed care.J Am Geriatr Soc.1997;45(5):614–617. , , , .
- Brief scale measuring patient preparedness for hospital discharge to home: psychometric properties.J Hosp Med.2008;3(6):446–454. , , .
- SF‐36 health survey update.Spine.2000;25(24):3130–3139. .
- Development of a method to identify seniors at high risk for high hospital utilization.Med Care.2002;40(9):782–793. , , , , , .
- Comprehensive discharge planning and home follow‐up of hospitalized elders: arandomized clinical trial.JAMA.1999;281(7):613–620. , , , et al.
- Predicting non‐elective hospital readmissions: a multi‐site study. Department of Veterans Affairs Cooperative Study Group on Primary Care and Readmissions.J Clin Epidemiol.2000;53:1113–1118. , , , et al.
- Identification of factors associated with hospital readmission and development of a predictive model.Health Serv Res.1992;27(1):81–101. , .
- Risk‐adjusting acute myocardial infarction mortality: are APR‐DRGs the right tool?Health Serv Res.2000;34(7):1469–1489. , .
- Adapting a clinical comorbidity index for use with ICD‐9‐CM administrative databases.J Clin Epidemiol.1992;45(6):613–619. , , .
- The community assessment risk screen (CARS): identifying elderly persons at risk for hospitalization or emergency department visit.Am J Manag Care.2000;6(8):925–933. , , .
- Clinical Epidemiology: A Basic Science for Clinical Medicine.2nd ed.Boston:Little, Brown;1991. , , , .
- Identifying clinically significant preventable adverse drug events through a hospital's database of adverse drug reaction reports.Am J Health Syst Pharm.2002;59(18):1742–1749. , , , , .
- A pharmacy discharge plan for hospitalized elderly patients—a randomized controlled trial.Age Ageing.2001;30(1):33–40. , , , , , .
- Can GP input into discharge planning result in better outcomes for the frail aged: results from a randomized controlled trial.Fam Pract.1999;16(3):289–293. , , , , .
- Comprehensive discharge planning with postdischarge support for older patients with congestive heart failure: a meta‐analysis.JAMA.2004;291(11):1358–1367. , , , , , .
- Can readmission after stroke be prevented? Results of a randomized clinical study: a postdischarge follow‐up service for stroke survivors.Stroke.2000;31(5):1038–1045. , , , , , .
- Does increased access to primary care reduce hospital readmissions? Veterans Affairs Cooperative Study Group on Primary Care and Hospital Readmission.N Engl J Med.1996;334(22):1441–147. , , .
- Indexes and boundaries for “quantitative significance” in statistical decisions.J Clin Epidemiol.1990;43(12):1273–1284. , , .
- National Quality Forum. Safe Practices for Better Healthcare 2006 Update, A Consensus Report, Safe Practice 11: Discharge Systems. Available at: http://qualityforum.org/pdf/reports/safe_practices/txsppublic.pdf. Accessed January 2009.
- OSF Healthcare's journey in patient safety.Qual Manag Health Care.2004;13(1):53–59. , .
- The incidence and severity of adverse events affecting patients after discharge from the hospital.Ann Intern Med.2003;138(3):161–167. , , , , .
- Adverse events among medical patients after discharge from hospital.CMAJ.2004;170(3):345–349. , , , et al.
- Frequency of new or worsening symptoms in the posthospitalization period.J Hosp Med.2007;2(2):58–68. , , , , .
- Written and verbal information versus verbal information only for patients being discharged from acute hospital settings to home.Cochrane Database Syst Rev.2003;(4):CD003716. , , .
- Discharge planning from hospital to home.Cochrane Database Syst Rev.2004;(1):CD000313. , , , .
- Deficits in communication and information transfer between hospital‐based and primary care physicians: implications for patient safety and continuity of care.JAMA.2007;297(8):831–841. , , , , , .
- Software design to facilitate information transfer at hospital discharge.Inform Prim Care.2006;14(2):109–119. , , .
- Effects of computerized physician order entry and clinical decision support systems on medication safety: a systematic review.Arch Intern Med.2003;163(12):1409–1416. , , .
- Computer physician order entry: benefits, costs, and issues.Ann Intern Med.2003;139(1):31–39. , .
- Systematic review: impact of health information technology on quality, efficiency, and costs of medical care.Ann Intern Med.2006;144(10):742–752. , , , et al.
- Compliance with post‐hospitalization follow‐up visits: rationing by inconvenience?Ethn Dis.1999;9(3):387–395. , , , , , .
- A computerized reminder system to increase the use of preventive care for hospitalized patients.N Engl J Med.2001;345(13):965–970. , , , , , .
- Evaluation of a new integrated discharge prescription form.Ann Pharmacother.2001;35(7‐8):953–958. , , , .
- Role of pharmacist counseling in preventing adverse drug events after hospitalization.Arch Intern Med.2006;166(5):565–571. , , , et al.
- Factors associated with unplanned hospital readmission among patients 65 years of age and older in a Medicare managed care plan.Am J Med.1999;107(1):13–17. , , , , , .
- Closing the loop of patient care—a clinical trial of a computerized discharge medication program.Proc Annu Symp Comput Appl Med Care.1994:841–845. , .
- A comprehensive inpatient discharge system.Proc AMIA Annu Fall Symp.1996:699–703. , , , .
- Agency for Healthcare Research and Quality. Making health care safer: a critical analysis of patient safety practices, subchapter 42.3. Discharge summaries and follow‐up. Available at: http://www.ahrq.gov/clinic/ptsafety/chap42b. htm#42.3. Accessed January 2009.
- Predictive validity of a questionnaire that identifies older persons at risk for hospital admission.J Am Geriatr Soc.1995;43(4):374–377. , , .
- Predictive validity of the Pra instrument among older recipients of managed care.J Am Geriatr Soc.1997;45(5):614–617. , , , .
- Brief scale measuring patient preparedness for hospital discharge to home: psychometric properties.J Hosp Med.2008;3(6):446–454. , , .
- SF‐36 health survey update.Spine.2000;25(24):3130–3139. .
- Development of a method to identify seniors at high risk for high hospital utilization.Med Care.2002;40(9):782–793. , , , , , .
- Comprehensive discharge planning and home follow‐up of hospitalized elders: arandomized clinical trial.JAMA.1999;281(7):613–620. , , , et al.
- Predicting non‐elective hospital readmissions: a multi‐site study. Department of Veterans Affairs Cooperative Study Group on Primary Care and Readmissions.J Clin Epidemiol.2000;53:1113–1118. , , , et al.
- Identification of factors associated with hospital readmission and development of a predictive model.Health Serv Res.1992;27(1):81–101. , .
- Risk‐adjusting acute myocardial infarction mortality: are APR‐DRGs the right tool?Health Serv Res.2000;34(7):1469–1489. , .
- Adapting a clinical comorbidity index for use with ICD‐9‐CM administrative databases.J Clin Epidemiol.1992;45(6):613–619. , , .
- The community assessment risk screen (CARS): identifying elderly persons at risk for hospitalization or emergency department visit.Am J Manag Care.2000;6(8):925–933. , , .
- Clinical Epidemiology: A Basic Science for Clinical Medicine.2nd ed.Boston:Little, Brown;1991. , , , .
- Identifying clinically significant preventable adverse drug events through a hospital's database of adverse drug reaction reports.Am J Health Syst Pharm.2002;59(18):1742–1749. , , , , .
- A pharmacy discharge plan for hospitalized elderly patients—a randomized controlled trial.Age Ageing.2001;30(1):33–40. , , , , , .
- Can GP input into discharge planning result in better outcomes for the frail aged: results from a randomized controlled trial.Fam Pract.1999;16(3):289–293. , , , , .
- Comprehensive discharge planning with postdischarge support for older patients with congestive heart failure: a meta‐analysis.JAMA.2004;291(11):1358–1367. , , , , , .
- Can readmission after stroke be prevented? Results of a randomized clinical study: a postdischarge follow‐up service for stroke survivors.Stroke.2000;31(5):1038–1045. , , , , , .
- Does increased access to primary care reduce hospital readmissions? Veterans Affairs Cooperative Study Group on Primary Care and Hospital Readmission.N Engl J Med.1996;334(22):1441–147. , , .
- Indexes and boundaries for “quantitative significance” in statistical decisions.J Clin Epidemiol.1990;43(12):1273–1284. , , .
- National Quality Forum. Safe Practices for Better Healthcare 2006 Update, A Consensus Report, Safe Practice 11: Discharge Systems. Available at: http://qualityforum.org/pdf/reports/safe_practices/txsppublic.pdf. Accessed January 2009.
- OSF Healthcare's journey in patient safety.Qual Manag Health Care.2004;13(1):53–59. , .
Copyright © 2009 Society of Hospital Medicine