User login
Controlling Medicare Spending
Payers, providers, and policymakers are testing several major approaches to reducing US healthcare spending without harming quality. One strategy is to bundle payments for a longitudinal episode of care, as in Medicare's popular Bundled Payments for Care Improvement initiative.[1] A second approach is to decrease rates of inappropriate care, through programs such as Choosing Wisely, that discourage use of low‐value services.[2] Finally, a third approach adopted by the Medicare Shared Savings Program strives to reduce both episode costs and rates of inappropriate care, by incorporating annual per capita Medicare spending into performance benchmarks.[3] Given these ongoing efforts, it would be important to compare the potential impact of reducing episode payments versus rates of care on total costs of care.
METHODS
For 3 common surgical procedures, we compared the relative influence of procedure rates versus episode payments (among those with procedures) on total Medicare expenditures.
We used complete Part A and B Medicare claims data for: coronary artery bypass grafting (CABG), prostatectomy, and hip replacement. We used International Classification of Diseases, Ninth Revision codes to identify the procedures (CABG: 361.0, 361.1, 361.2, 361.3, 361.4, 361.5, 361.6, 361.7, 361.9, 36.2; prostatectomy: 60.4, 60.5, 60.62 with a prostate cancer diagnosis code of 185 or 233.4; and hip replacement: 81.51, 81.52 excluding hip fracture codes 820.0, 820.1, 820.2, 820.3, 820.8, 820.9).
For each procedure, we estimated age‐ and sex‐adjusted episode rates for each hospital referral region (HRR). The numerator was the number of admissions to an acute care hospital for CABG (total n = 118,185), prostatectomy (total n = 18,328), or hip replacement (total n = 178,982) from January 2009 to June 2010. The denominator was fee‐for‐service Medicare beneficiaries age 65 years or older. We excluded those without continuous Part A and B enrollment (total denominator n = 23,403,051). Females were also excluded from the prostatectomy cohort.
For each of the 306 HRRs, we next calculated average HRR‐level episode payments. Using CABG as an example, we aggregated up the risk‐adjusted (age, sex, race, admission type, Elixhauser[4] comorbidities), price‐standardized[5] episode payments for all CABG patients residing in an HRR, and divided this by the number of CABG patients living in that HRR.
Finally, we obtained baseline per capita spending by multiplying the age‐ and sex‐adjusted CABG episode rate by the average CABG episode payment in that HRR. All payments were standardized to 2010 dollars using the Consumer Price Index.
We simulated changes in per capita Medicare spending for CABG across all HRRs under 2 scenarios: (1) reducing HRR‐level rates to the median versus (2) reducing HRR‐level episode payments to the median. We repeated this for prostatectomy and hip replacement.
RESULTS
Age‐ and sex‐adjusted rates of CABG varied more than risk‐adjusted, price‐standardized episode payments (90th:10th percentile of 2.0 for rates vs 1.2 for payments) (see Supporting Information, Appendix, in the online version of this article). Reducing rates of CABG to the 50th percentile decreased per capita episode payments by 11.1%. In contrast, reducing CABG episode payments to the 50th percentile decreased per capita episode payments by 3.6%. The absolute difference between the 2 simulations was 7.5% (95% confidence interval [CI]: 5.6%‐9.4%) (Figure 1). Results were similar for prostatectomy and hip replacement. In sensitivity analyses, reducing hospital‐level episode payments (rather than HRR‐level episode payments) produced similar findings. Employing the 90th percentile as a cutoff (instead of the median) also produced qualitatively similar results.
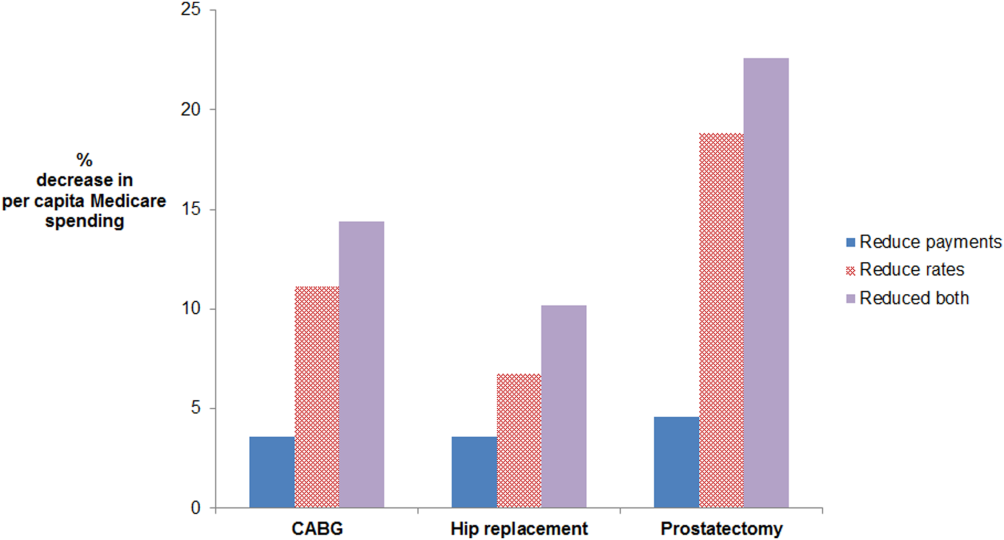
For CABG, the absolute difference between the simulated decrease in per capita Medicare spending due to reducing rates versus reducing payments was 7.5% (95% confidence interval [CI]: 5.6%‐9.4%). For hip replacement, the absolute difference was 3.2% (95% CI: 1.9%‐4.4%). For prostatectomy, the absolute difference was 14.3% (95% CI: 11.0%‐17.6%). The effect of doing both is additive in this simulation, as we did not model possible complex interdependencies between reducing payments and rates. Abbreviations: CABG, coronary artery bypass grafting.
DISCUSSION
For 3 common surgical procedures, reducing procedure rates lowers total Medicare spending substantially more than reducing episode payments. These findings are attributable to a much greater variation in procedure rates compared to episode‐based payments. Prior research has documented wide variation in rates of surgical procedures.[6] This may be due to a number of factors, including physician beliefs about indications for surgery, as well as the degree to which patient preferences are incorporated into decision making.[6]
Our findings suggest that it would be important to incorporate population‐based episode rates into efforts aimed at incentivizing higher value care. Incentives tied to population‐based episode rates are difficult to design well. They may need to be paired with appropriateness criteria to avoid stinting on care. Attribution of a population to a hospital (including those who are not admitted to a hospital) is also complex.[7] Finally, hospitals are not solely responsible for rates of care, because the decision to admit a patient is sometimes made in the emergency department (eg, for chronic medical conditions), but at other times is made in the outpatient arena (eg, for elective surgery). Nevertheless, a narrow focus on per episode spending limits the potential impact of efforts to control Medicare spending.
Acknowledgements
The authors thank Mary Oerline, MS, Yubraj Acharya, MPA, and Haiyin Liu, MA, for the analytic support they provided. They were compensated for their work.
Disclosures: Dr. John Birkmeyer has equity interest in ArborMetrix, a company that profiles hospital quality and episode cost efficiency. The company played no role in the preparation of this article. This work was supported by funding from the National Institute of Aging (grant no. P01AG019783). Dr. Lena Chen is supported by a Career Development Grant Award (K08HS020671) from the Agency for Healthcare Research and Quality. The funders had no role in the design and conduct of the study, in the collection, analysis, and interpretation of the data, and in the preparation, review, or approval of the manuscript.
- Centers for Medicare 370(7):589–592.
- Centers for Medicare 36(1):8–27.
- Prices don't drive regional Medicare spending variations. Health Aff (Millwood). 2010;29(3):537–543. , , , , , .
- Understanding of regional variation in the use of surgery. Lancet. 2013;382(9898):1121–1129. , , , , , .
- Assigning ambulatory patients and their physicians to hospitals: a method for obtaining population‐based provider performance measurements. Health Serv Res. 2007;42(1 pt 1):45–62. , , , .
Payers, providers, and policymakers are testing several major approaches to reducing US healthcare spending without harming quality. One strategy is to bundle payments for a longitudinal episode of care, as in Medicare's popular Bundled Payments for Care Improvement initiative.[1] A second approach is to decrease rates of inappropriate care, through programs such as Choosing Wisely, that discourage use of low‐value services.[2] Finally, a third approach adopted by the Medicare Shared Savings Program strives to reduce both episode costs and rates of inappropriate care, by incorporating annual per capita Medicare spending into performance benchmarks.[3] Given these ongoing efforts, it would be important to compare the potential impact of reducing episode payments versus rates of care on total costs of care.
METHODS
For 3 common surgical procedures, we compared the relative influence of procedure rates versus episode payments (among those with procedures) on total Medicare expenditures.
We used complete Part A and B Medicare claims data for: coronary artery bypass grafting (CABG), prostatectomy, and hip replacement. We used International Classification of Diseases, Ninth Revision codes to identify the procedures (CABG: 361.0, 361.1, 361.2, 361.3, 361.4, 361.5, 361.6, 361.7, 361.9, 36.2; prostatectomy: 60.4, 60.5, 60.62 with a prostate cancer diagnosis code of 185 or 233.4; and hip replacement: 81.51, 81.52 excluding hip fracture codes 820.0, 820.1, 820.2, 820.3, 820.8, 820.9).
For each procedure, we estimated age‐ and sex‐adjusted episode rates for each hospital referral region (HRR). The numerator was the number of admissions to an acute care hospital for CABG (total n = 118,185), prostatectomy (total n = 18,328), or hip replacement (total n = 178,982) from January 2009 to June 2010. The denominator was fee‐for‐service Medicare beneficiaries age 65 years or older. We excluded those without continuous Part A and B enrollment (total denominator n = 23,403,051). Females were also excluded from the prostatectomy cohort.
For each of the 306 HRRs, we next calculated average HRR‐level episode payments. Using CABG as an example, we aggregated up the risk‐adjusted (age, sex, race, admission type, Elixhauser[4] comorbidities), price‐standardized[5] episode payments for all CABG patients residing in an HRR, and divided this by the number of CABG patients living in that HRR.
Finally, we obtained baseline per capita spending by multiplying the age‐ and sex‐adjusted CABG episode rate by the average CABG episode payment in that HRR. All payments were standardized to 2010 dollars using the Consumer Price Index.
We simulated changes in per capita Medicare spending for CABG across all HRRs under 2 scenarios: (1) reducing HRR‐level rates to the median versus (2) reducing HRR‐level episode payments to the median. We repeated this for prostatectomy and hip replacement.
RESULTS
Age‐ and sex‐adjusted rates of CABG varied more than risk‐adjusted, price‐standardized episode payments (90th:10th percentile of 2.0 for rates vs 1.2 for payments) (see Supporting Information, Appendix, in the online version of this article). Reducing rates of CABG to the 50th percentile decreased per capita episode payments by 11.1%. In contrast, reducing CABG episode payments to the 50th percentile decreased per capita episode payments by 3.6%. The absolute difference between the 2 simulations was 7.5% (95% confidence interval [CI]: 5.6%‐9.4%) (Figure 1). Results were similar for prostatectomy and hip replacement. In sensitivity analyses, reducing hospital‐level episode payments (rather than HRR‐level episode payments) produced similar findings. Employing the 90th percentile as a cutoff (instead of the median) also produced qualitatively similar results.
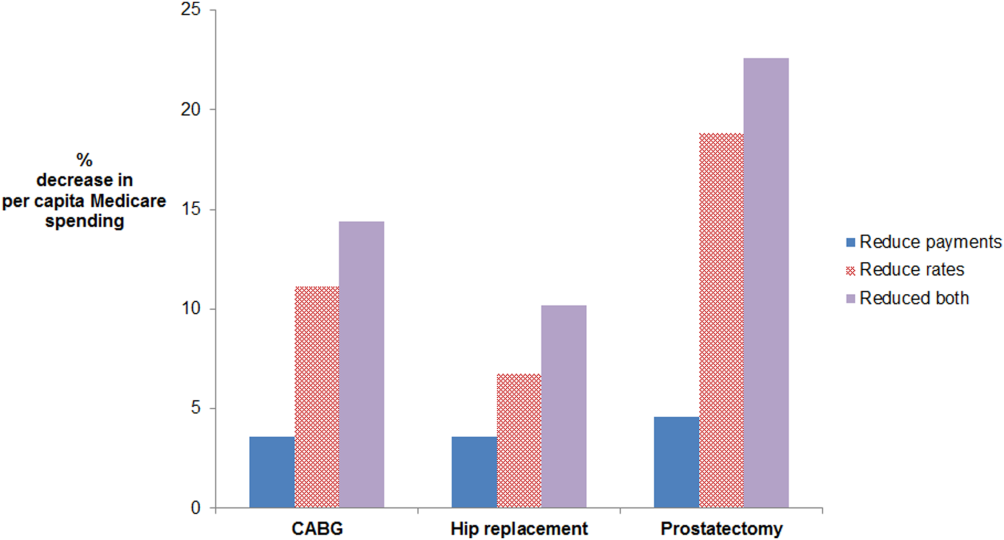
For CABG, the absolute difference between the simulated decrease in per capita Medicare spending due to reducing rates versus reducing payments was 7.5% (95% confidence interval [CI]: 5.6%‐9.4%). For hip replacement, the absolute difference was 3.2% (95% CI: 1.9%‐4.4%). For prostatectomy, the absolute difference was 14.3% (95% CI: 11.0%‐17.6%). The effect of doing both is additive in this simulation, as we did not model possible complex interdependencies between reducing payments and rates. Abbreviations: CABG, coronary artery bypass grafting.
DISCUSSION
For 3 common surgical procedures, reducing procedure rates lowers total Medicare spending substantially more than reducing episode payments. These findings are attributable to a much greater variation in procedure rates compared to episode‐based payments. Prior research has documented wide variation in rates of surgical procedures.[6] This may be due to a number of factors, including physician beliefs about indications for surgery, as well as the degree to which patient preferences are incorporated into decision making.[6]
Our findings suggest that it would be important to incorporate population‐based episode rates into efforts aimed at incentivizing higher value care. Incentives tied to population‐based episode rates are difficult to design well. They may need to be paired with appropriateness criteria to avoid stinting on care. Attribution of a population to a hospital (including those who are not admitted to a hospital) is also complex.[7] Finally, hospitals are not solely responsible for rates of care, because the decision to admit a patient is sometimes made in the emergency department (eg, for chronic medical conditions), but at other times is made in the outpatient arena (eg, for elective surgery). Nevertheless, a narrow focus on per episode spending limits the potential impact of efforts to control Medicare spending.
Acknowledgements
The authors thank Mary Oerline, MS, Yubraj Acharya, MPA, and Haiyin Liu, MA, for the analytic support they provided. They were compensated for their work.
Disclosures: Dr. John Birkmeyer has equity interest in ArborMetrix, a company that profiles hospital quality and episode cost efficiency. The company played no role in the preparation of this article. This work was supported by funding from the National Institute of Aging (grant no. P01AG019783). Dr. Lena Chen is supported by a Career Development Grant Award (K08HS020671) from the Agency for Healthcare Research and Quality. The funders had no role in the design and conduct of the study, in the collection, analysis, and interpretation of the data, and in the preparation, review, or approval of the manuscript.
Payers, providers, and policymakers are testing several major approaches to reducing US healthcare spending without harming quality. One strategy is to bundle payments for a longitudinal episode of care, as in Medicare's popular Bundled Payments for Care Improvement initiative.[1] A second approach is to decrease rates of inappropriate care, through programs such as Choosing Wisely, that discourage use of low‐value services.[2] Finally, a third approach adopted by the Medicare Shared Savings Program strives to reduce both episode costs and rates of inappropriate care, by incorporating annual per capita Medicare spending into performance benchmarks.[3] Given these ongoing efforts, it would be important to compare the potential impact of reducing episode payments versus rates of care on total costs of care.
METHODS
For 3 common surgical procedures, we compared the relative influence of procedure rates versus episode payments (among those with procedures) on total Medicare expenditures.
We used complete Part A and B Medicare claims data for: coronary artery bypass grafting (CABG), prostatectomy, and hip replacement. We used International Classification of Diseases, Ninth Revision codes to identify the procedures (CABG: 361.0, 361.1, 361.2, 361.3, 361.4, 361.5, 361.6, 361.7, 361.9, 36.2; prostatectomy: 60.4, 60.5, 60.62 with a prostate cancer diagnosis code of 185 or 233.4; and hip replacement: 81.51, 81.52 excluding hip fracture codes 820.0, 820.1, 820.2, 820.3, 820.8, 820.9).
For each procedure, we estimated age‐ and sex‐adjusted episode rates for each hospital referral region (HRR). The numerator was the number of admissions to an acute care hospital for CABG (total n = 118,185), prostatectomy (total n = 18,328), or hip replacement (total n = 178,982) from January 2009 to June 2010. The denominator was fee‐for‐service Medicare beneficiaries age 65 years or older. We excluded those without continuous Part A and B enrollment (total denominator n = 23,403,051). Females were also excluded from the prostatectomy cohort.
For each of the 306 HRRs, we next calculated average HRR‐level episode payments. Using CABG as an example, we aggregated up the risk‐adjusted (age, sex, race, admission type, Elixhauser[4] comorbidities), price‐standardized[5] episode payments for all CABG patients residing in an HRR, and divided this by the number of CABG patients living in that HRR.
Finally, we obtained baseline per capita spending by multiplying the age‐ and sex‐adjusted CABG episode rate by the average CABG episode payment in that HRR. All payments were standardized to 2010 dollars using the Consumer Price Index.
We simulated changes in per capita Medicare spending for CABG across all HRRs under 2 scenarios: (1) reducing HRR‐level rates to the median versus (2) reducing HRR‐level episode payments to the median. We repeated this for prostatectomy and hip replacement.
RESULTS
Age‐ and sex‐adjusted rates of CABG varied more than risk‐adjusted, price‐standardized episode payments (90th:10th percentile of 2.0 for rates vs 1.2 for payments) (see Supporting Information, Appendix, in the online version of this article). Reducing rates of CABG to the 50th percentile decreased per capita episode payments by 11.1%. In contrast, reducing CABG episode payments to the 50th percentile decreased per capita episode payments by 3.6%. The absolute difference between the 2 simulations was 7.5% (95% confidence interval [CI]: 5.6%‐9.4%) (Figure 1). Results were similar for prostatectomy and hip replacement. In sensitivity analyses, reducing hospital‐level episode payments (rather than HRR‐level episode payments) produced similar findings. Employing the 90th percentile as a cutoff (instead of the median) also produced qualitatively similar results.
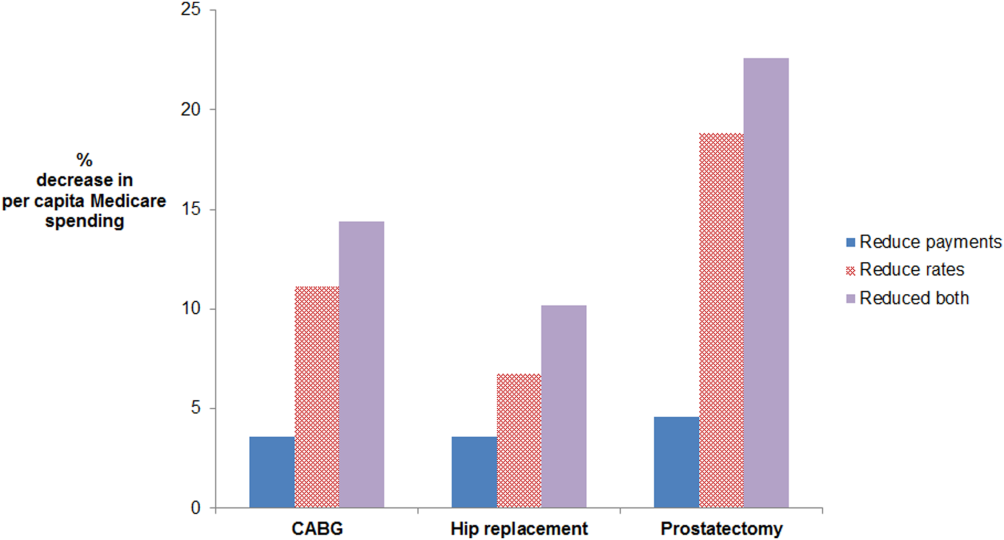
For CABG, the absolute difference between the simulated decrease in per capita Medicare spending due to reducing rates versus reducing payments was 7.5% (95% confidence interval [CI]: 5.6%‐9.4%). For hip replacement, the absolute difference was 3.2% (95% CI: 1.9%‐4.4%). For prostatectomy, the absolute difference was 14.3% (95% CI: 11.0%‐17.6%). The effect of doing both is additive in this simulation, as we did not model possible complex interdependencies between reducing payments and rates. Abbreviations: CABG, coronary artery bypass grafting.
DISCUSSION
For 3 common surgical procedures, reducing procedure rates lowers total Medicare spending substantially more than reducing episode payments. These findings are attributable to a much greater variation in procedure rates compared to episode‐based payments. Prior research has documented wide variation in rates of surgical procedures.[6] This may be due to a number of factors, including physician beliefs about indications for surgery, as well as the degree to which patient preferences are incorporated into decision making.[6]
Our findings suggest that it would be important to incorporate population‐based episode rates into efforts aimed at incentivizing higher value care. Incentives tied to population‐based episode rates are difficult to design well. They may need to be paired with appropriateness criteria to avoid stinting on care. Attribution of a population to a hospital (including those who are not admitted to a hospital) is also complex.[7] Finally, hospitals are not solely responsible for rates of care, because the decision to admit a patient is sometimes made in the emergency department (eg, for chronic medical conditions), but at other times is made in the outpatient arena (eg, for elective surgery). Nevertheless, a narrow focus on per episode spending limits the potential impact of efforts to control Medicare spending.
Acknowledgements
The authors thank Mary Oerline, MS, Yubraj Acharya, MPA, and Haiyin Liu, MA, for the analytic support they provided. They were compensated for their work.
Disclosures: Dr. John Birkmeyer has equity interest in ArborMetrix, a company that profiles hospital quality and episode cost efficiency. The company played no role in the preparation of this article. This work was supported by funding from the National Institute of Aging (grant no. P01AG019783). Dr. Lena Chen is supported by a Career Development Grant Award (K08HS020671) from the Agency for Healthcare Research and Quality. The funders had no role in the design and conduct of the study, in the collection, analysis, and interpretation of the data, and in the preparation, review, or approval of the manuscript.
- Centers for Medicare 370(7):589–592.
- Centers for Medicare 36(1):8–27.
- Prices don't drive regional Medicare spending variations. Health Aff (Millwood). 2010;29(3):537–543. , , , , , .
- Understanding of regional variation in the use of surgery. Lancet. 2013;382(9898):1121–1129. , , , , , .
- Assigning ambulatory patients and their physicians to hospitals: a method for obtaining population‐based provider performance measurements. Health Serv Res. 2007;42(1 pt 1):45–62. , , , .
- Centers for Medicare 370(7):589–592.
- Centers for Medicare 36(1):8–27.
- Prices don't drive regional Medicare spending variations. Health Aff (Millwood). 2010;29(3):537–543. , , , , , .
- Understanding of regional variation in the use of surgery. Lancet. 2013;382(9898):1121–1129. , , , , , .
- Assigning ambulatory patients and their physicians to hospitals: a method for obtaining population‐based provider performance measurements. Health Serv Res. 2007;42(1 pt 1):45–62. , , , .
IVC Filters in Bariatric Surgery
The use of inferior vena cava (IVC) filters has increased substantially in recent years. These medical devices, which are used to prevent pulmonary embolism in patients considered to be at high risk of venous thromboembolism, were placed in 167,000 patients in 2007.1 In 2012, it is estimated that 259,000 patients will undergo placement of an IVC filter, an increase of 55%.[1] Increasing use of IVC filters is attributable to the development of retrievable versions of the devices, which have expanded indications for use such as in bariatric surgery.
Unfortunately, the increase in the use of IVC filters has been accompanied by an increase in reports of adverse events in patients receiving them. The United States Food and Drug Administration (FDA) has received more than 900 adverse event reports involving IVC filters, prompting the agency to issue a warning about their use.[2] A prior study by our group demonstrated a lack of benefit of IVC filter insertion for the prevention of pulmonary embolism among bariatric surgery patients but lacked statistical power to prove harms associated with this practice.[3]
In the current study, we analyzed data from the prospective, statewide, clinical registry of the Michigan Bariatric Surgery Collaborative. Our study population now includes 35,477 bariatric surgery patients from 32 hospitals whose procedures were performed between 2006 and 2012. Since the publication of our prior study, the use of IVC filters in bariatric surgery has decreased significantly in Michigan. For this reason, our study population now includes many more high‐risk patients who did not undergo IVC filter placement, allowing us to match IVC filter patients to similarly high‐risk patients who did not receive IVC filters. We used these data to compare outcomes within 30 days of surgery, including rates of venous thromboembolism, overall serious complications, and death among patients who did and not receive IVC filters.
METHODS
Study Setting
The Michigan Bariatric Surgery Collaborative (MBSC) is a regional voluntary consortium of hospitals and surgeons that perform bariatric surgery in Michigan. The goal of the project is to improve the quality of care for patients undergoing bariatric surgery. To do this, the participating hospitals submit data to the MBSC clinical outcomes registry, patient survey, and surgeon survey databases. Three times per year the group meets to examine these data and to design and implement changes in care to improve the outcomes of care for bariatric patients. The project is funded by Blue Cross and Blue Shield of Michigan/Blue Care Network and coordinated by faculty and staff members from the Center for Healthcare Outcomes and Policy at the University of Michigan.
The MBSC held its first collaborative meeting in June 2005 and enrolled its first patient in June 2006. The MBSC now has the participation of all of the 32 bariatric programs in Michigan, enrolling approximately 6000 patients per year in its clinical registry. Participating hospitals submit data from a review of the medical records for all of their bariatric surgery patients. This review is conducted for each patient at 30 days after surgery. The information collected includes preoperative clinical characteristics and conditions as well as perioperative clinical care and outcomes. The medical record reviews are performed by centrally trained, nurse data abstractors using a standardized and validated instrument. Each participating hospital is site visited annually to verify the accuracy and completeness of their MBSC clinical registry data.
Study Population
This study includes data for 35,477 patients undergoing bariatric surgery, including: 9829 laparoscopic adjustable gastric band, 6068 sleeve gastrectomy, 19,141 gastric bypass, and 439 biliopancreatic diversion with duodenal switch procedures between June 2006 and September 2012. Patients undergoing revisional bariatric surgery were excluded from these analyses. Prior to surgery, 1077 (3.0%) of these patients had a prophylactic IVC filter placed for prevention of pulmonary embolism. Of the IVC filters placed, 39% were temporary IVC filters, 45% were permanent IVC filters, and the type of IVC filter was not known in 15%.
Baseline Clinical Characteristics
Data collected included patient demographic characteristics (age, gender, race, type of insurance), clinical characteristics (height, weight, history of cigarette smoking, mobility limitations), and obesity‐related and other comorbid conditions (lung disease, cardiovascular disease, hyperlipidemia, gastroesophageal reflux disease, peptic ulcer disease, cholelithiasis, urinary incontinence, renal disease, diabetes, liver disease, prior history of venous thromboembolism, sleep apnea, and psychological disorders).
Risk factors for VTE were empirically derived from our data base using multivariate statistical models. Risk factors for VTE included: age, body mass index, male sex, current or past smoking, mobility limitations, asthma, home oxygen use, peripheral vascular disease, prior history of VTE, bariatric procedure time, and procedure type. The baseline predicted risk for VTE was calculated for each patient based on these risk factors and was used to divide patients into low‐ (predicted risk <1%), medium‐ (predicted risk 1%2.5%), and high‐ (predicted risk 2.5%) risk groups. Among the 35,477 patients in the registry overall, 95% are in the low‐risk group, 4% are in the medium‐risk group, and 1% are in the high‐risk group. In the matched study cohorts, 69%, 22%, and 9% were in the high‐, medium‐, and low‐risk groups, respectively.
Medical Venous Thromboembolism Prophylaxis
Data were also collected regarding the type of medical venous thromboembolism prophylaxis (unfractionated vs low molecular weight heparin) used preoperatively, postoperatively, and whether the patient was discharged to home on low molecular weight heparin.
Outcomes
Our primary outcome measures included postoperative venous thromboembolism (deep vein thrombosis or pulmonary embolism requiring treatment). We also assessed overall rate of complications and complications according to severity as follows: non‐life threatening complications (surgical site infection including wound and port site infections treated with antibiotics and/or wound opening, anastomotic stricture requiring dilatation, bleeding requiring blood transfusion of <4 units, and pneumonia requiring treatment with antibiotics only); potentially life‐threatening complications (abdominal abscess requiring percutaneous drainage or reoperation, bowel obstruction requiring reoperation, leak requiring percutaneous drainage or reoperation, bleeding requiring transfusion >4 units, reoperation, or splenectomy, band‐related problems requiring reoperation, respiratory failure requiring 2 to 7 days intubation, renal failure requiring in‐hospital dialysis, wound infection/dehiscence requiring reoperation, and venous thromboembolism); and life‐threatening complications associated with residual and lasting disability or death (myocardial infarction or cardiac arrest, renal failure requiring long‐term dialysis, respiratory failure requiring >7 days intubation or tracheostomy, and death). Other complications that are not included in these categories (eg, IVC filter related) were assessed by an end points committee to determine their severity (non‐life threatening, potentially life threatening, or life threatening associated with residual and lasting disability or death).
Statistical Analyses
We used propensity score matching to assemble cohorts in which patients with and without IVC filters were balanced on baseline characteristics. The probability of IVC filter placement was estimated for each patient using a nonparsimonious multivariate logistic regression model, in which IVC filter was the dependent variable and all of the demographic, weight, medical history, weight‐related comorbidity, and procedure‐related variables (type, length, and year of procedure; and medical venous thromboembolism prophylaxis used) in our dataset were included as covariates. IVC filter patients were matched to control patients using a greedy, 1‐ to ‐1 matching without replacement protocol resulting in cohorts that were well balanced on all baseline characteristics.
Baseline characteristics and outcomes were then compared among the cohorts using [2] and t tests as appropriate. We used mixed effects logistic regression to compare outcomes between the 2 treatment groups while controlling for clustering at the hospital and surgeon level as random effects. Odds ratios (OR) and 95% confidence intervals (CI) were calculated to compare outcomes among patients with and without IVC filters.
RESULTS
Matching resulted in cohorts of IVC filter and control patients who were well balanced on all baseline characteristics (Table 1). In contrast, there were large and significant differences between IVC filter patients and unmatched control patients. For example, mean body mass index was 58 and 57 in the matched cohorts and 47 in the unmatched control patients. Prior history of venous thromboembolism was present in 39%, 39%, and 2% of the IVC filter, matched control, and unmatched control patients, respectively. With regard to procedure mix, unmatched control patients were less likely to have open gastric bypass and more likely to have adjustable gastric band procedures than IVC filter or matched control patients.
Variable | IVC Filter | Matched Controls | P Value | Unmatched Controls | P Value |
---|---|---|---|---|---|
| |||||
No. | 1077 | 1077 | 33,323 | ||
Age (mean, y) | 48 | 49 | 0.295 | 46 | <0.0001 |
Body mass index (mean, kg/m2) | 58 | 57 | 0.061 | 47 | <0.0001 |
Male gender (%) | 32 | 31 | 0.546 | 21 | <0.0001 |
Black race (%) | 27 | 25 | 0.667 | 15 | <0.0001 |
Private Insurance (%) | 62 | 64 | 0.305 | 74 | <0.0001 |
Smoking in past year (%) | 2 | 2 | 0.883 | 2 | 0.440 |
Mobility limitations (%) | 18 | 18 | 0.780 | 5 | <0.0001 |
Lung dsease (%) | 43 | 43 | 1.000 | 25 | <0.0001 |
Cardiovascular disease (%) | 21 | 21 | 0.874 | 10 | <0.0001 |
Hypertension (%) | 72 | 72 | 0.737 | 53 | <0.0001 |
Hyperlipidemia (%) | 59 | 59 | 0.930 | 50 | <0.0001 |
GERD (%) | 50 | 52 | 0.490 | 49 | 0.417 |
Peptic ulcer disease (%) | 5 | 4 | 0.228 | 3 | <0.0001 |
Cholelithiasis (%) | 30 | 30 | 0.963 | 27 | 0.018 |
Urinary incontinence (%) | 25 | 25 | 0.960 | 22 | 0.029 |
Renal failure (%) | 0.4 | 0.6 | 0.526 | 0.2 | 0.298 |
Diabetes (%) | 46 | 48 | 0.546 | 33 | <0.0001 |
Liver disorder (%) | 4 | 4 | 0.584 | 5 | 0.184 |
Prior history of VTE (%) | 39 | 39 | 0.965 | 2 | <0.0001 |
Sleep apnea (%) | 70 | 68 | 0.209 | 43 | <0.0001 |
Musculoskeletal disorder (%) | 78 | 80 | 0.221 | 77 | 0.189 |
History of hernia repair (%) | 5 | 6 | 0.924 | 3 | <0.0001 |
Psychological disorder (%) | 49 | 49 | 0.796 | 47 | 0.267 |
Total comorbidities (mean, no.) | 6 | 6 | 0.922 | 4 | <0.0001 |
Procedure | |||||
Adjustable gastric banding (%) | 15 | 17 | 0.099 | 29 | <0.0001 |
Sleeve gastrectomy (%) | 12 | 13 | 0.515 | 17 | <0.0001 |
Gastric bypass (%) | 73 | 69 | 0.058 | 53 | <0.0001 |
Duodenal switch (%) | 0.7 | 0.8 | 0.616 | 1.3 | <0.0001 |
Procedure Length (mean, minutes) | 114 | 116 | 0.427 | 95 | <0.0001 |
Medical VTE prophylaxis | |||||
Preoperative heparin: | |||||
Unfractionated (%) | 36 | 38 | 0.246 | 34 | 0.306 |
Low molecular weight (%) | 60 | 54 | 0.017 | 53 | <0.0001 |
Postoperative heparin: | |||||
Unfractionated (%) | 7 | 10 | 0.023 | 19 | <0.0001 |
Low molecular weight (%) | 70 | 68 | 0.326 | 64 | <0.0001 |
Postdischarge heparin: | |||||
Low molecular weight (%) | 72 | 66 | 0.003 | 16 | <0.0001 |
With regard to outcomes (Table 2, Figures 1 and 2), IVC filter patients had significantly higher rates of venous thromboembolism (1.9% vs 0.74%; OR, 2.7; 95% CI, 1.1‐6.3; P=0.027) and deep vein thrombosis (1.2% vs 0.37%, OR, 3.3; 95% CI, 1.1‐10.1; P=0.039) than matched control patients. Rates of pulmonary embolism were higher among IVC filter patients, but the difference was not statistically significant (0.84% vs 0.46%; OR, 2.0; 95% CI, 0.6‐6.5; P=0.232). Rates of pulmonary embolism were similar for patients with a low baseline risk of venous thromboembolism (0.27% vs 0.27%; OR, 1.0; 95% CI, 0.1‐7.7; P=0.965) but were higher for medium‐risk patients (2.1% vs 0.87%; OR, 2.5; 95% CI, 0.5‐12.7; P=0.288) and high‐risk patients (2.1% vs 0.97%; OR, 2.2; 95% CI, 0.2‐24.3; P=0.530).
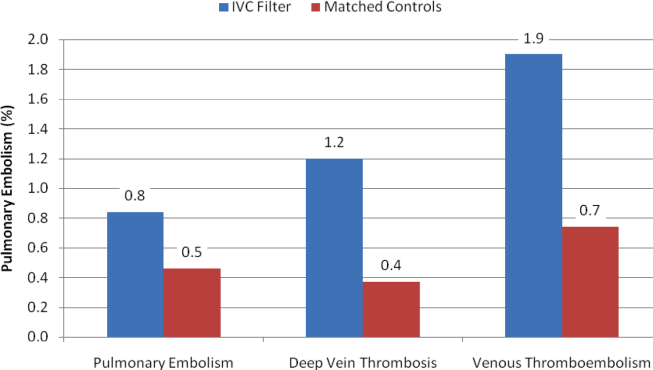
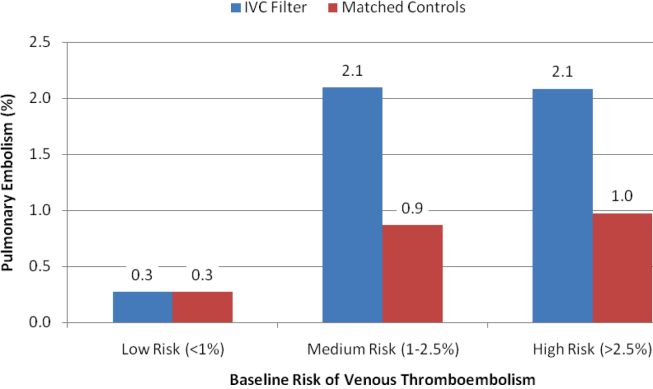
Outcomes | Odds Ratio | 95% Confidence Interval | P Value |
---|---|---|---|
Venous thromboembolism | 2.7 | 1.16.3 | 0.027 |
Deep vein thrombosis | 3.3 | 1.110.1 | 0.039 |
Pulmonary embolism | 2.0 | 0.66.5 | 0.232 |
Low‐risk subgroup | 1.0 | 0.17.7 | 0.965 |
Medium‐risk subgroup | 2.5 | 0.512.7 | 0.288 |
High‐risk subgroup | 2.2 | 0.224.3 | 0.530 |
Any complication | 1.3 | 1.01.7 | 0.048 |
Serious complication | 1.6 | 1.02.4 | 0.031 |
Permanently disabling complication | 4.3 | 1.215.6 | 0.028 |
Death | 7.0 | 0.957.3 | 0.068 |
Rates of other complications were also higher among IVC filter than matched control patients (Table 2 and Figure 3). There were significantly higher rates of complications (15.2% vs 11.6%; OR, 1.3; 95% CI, 1.0‐1.7; P=0.048), serious complications (5.8% vs 3.8%; OR, 1.6; 95% CI, 1.0‐2.4; P=0.031), and permanently disabling complications (1.2% vs 0.4%; OR, 4.3; 95% CI, 1.2‐15.6; P=0.028) among IVC filter patients. Rates of death (0.7% vs 0.1%; OR, 7.0; 95% CI, 0.9‐57.3; P=0.068) were also higher among IVC filter patients than matched control patients, but this difference was not statistically significant.
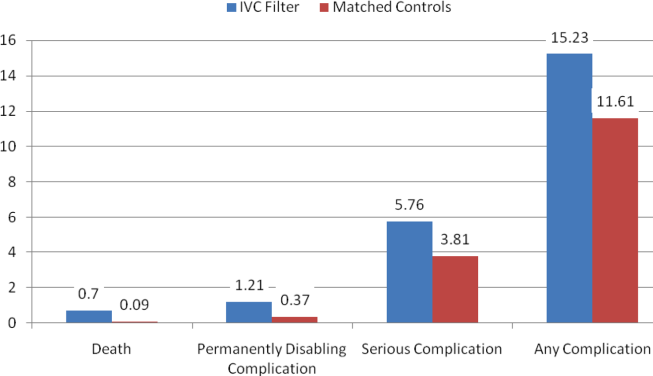
Among the 7 IVC filter patients who died, 4 had fatal pulmonary embolism, and 2 had IVC filter thrombosis/occlusion. Other IVC filter‐specific complications included IVC filter migration requiring heart valve replacement surgery in 1 patient, contrast‐induced nephropathy in 1 patient, IVC filter incision site infection in 1 patient, and technical difficulties removing a temporary IVC filter requiring that the device stay in place in 1 patient.
DISCUSSION
In this propensity matched, observational cohort study, we assessed the safety and effectiveness of prophylactic IVC filters among bariatric surgery patients. We found that patients with IVC filters had significantly worse outcomes than comparably high‐risk patients without IVC filters. Rates of venous thromboembolism were higher in the IVC filter patients, and a large proportion of the other complications among IVC filter patients were device related.
Our current study of IVC filters was prompted by an FDA advisory report regarding complications in patients receiving IVC filters.[2] The FDA's report was in turn prompted by a study indicating a high prevalence of strut fracture and embolization among 80 patients who received a certain type of retrievable IVC filter.[4] The FDA conveyed receiving 921 adverse events reports involving IVC filters between 2005 and 2009. Thirty‐six percent of these reports involved migration of the device, 16% were related to breakage and embolization of parts of the device, and 8% involved perforation of the IVC.
Research on the safety and efficacy of IVC filters in bariatric surgery patients has largely been limited to small, single‐center, case series or cohort studies.[5, 6, 7, 8, 9, 10, 11, 12, 13, 14, 15] A systematic review of this literature concluded that the evidence was insufficient to recommend IVC filters for patients undergoing bariatric surgery.[16] A 2010 study by our group was the largest and only multicenter study of IVC filters in the bariatric surgery population. We found no benefit of IVC filters in a comparison of 542 gastric bypass patients with prophylactic IVC filters to 5,834 gastric bypass patients without prophylactic IVC filters.[3]
When interpreting the results of this study, a number of limitations should be considered. Our study was observational, so there is the potential for unmeasured confounding variables to have influenced our results. To minimize the risk of confounding, we used propensity scores to match IVC filter patients to comparably high‐risk control patients, resulting in study cohorts that were well balanced on all baseline variables. Although this method accounts for confounding on the variables for which there are data, there is still the possibility that an unknown confounder could affect our findings. For example, our clinical registry lacks data on hypercoagulable states, so it is possible that a higher proportion of IVC filter patients could have had this risk factor and therefore a higher baseline risk of venous thromboembolism. However, most patients with a hypercoagulable state would have had a prior history of venous thromboembolism, which is a variable included in our database that patients were matched on.
The effects of changes in clinical care occurring during the time frame of this study should be considered in interpreting our findings. For example, bariatric surgery has been getting safer in general over time. Rates of death have fallen both in Michigan and in the rest of the country as bariatric surgeons have gained experience with this procedure. In Michigan during this time period, our group has developed and implemented a risk‐stratified, standardized approach to venous thromboembolism prophylaxis for patients undergoing bariatric surgery. For these reasons, we included the year of the procedure and the type of medical venous thromboembolism prophylaxis (unfractionated or low molecular weight heparin) used perioperatively as a matching variable in our analysis.
Another limitation that should be considered in interpreting our findings is statistical power. Although our study is the largest in this study population to date, many of the outcomes of interest are relatively rare. Considering the entire bariatric surgery population, rates of venous thromboembolism and death within 30 days are each less than 1%. Even in the high‐risk patients included in this analysis, there were a total of just 28 (1.3%) venous thromboembolism events and 8 (0.37) deaths. Nonetheless, our study did find significantly greater risks of multiple types of complications among patients receiving IVC filters.
Finally, our study captures events occurring within 30 days of bariatric surgery. Complications, including venous thromboembolism and other complications directly related to IVC filters, frequently occur after 30 days of bariatric surgery. Therefore, our study may be a conservative estimate of the risks associated with the use of IVC filters in bariatric surgery patients. Furthermore, certain brands of filters have been shown to be associated with higher risks of complications. Our study lacks data on the brand of IVC filter used and so cannot assess the extent to which this would affect our results.
CONCLUSIONS
In conclusion, our study indicates that IVC filters do not reduce the risk of pulmonary embolism in high‐risk bariatric surgery patients. They are also associated with other complications attributable to malfunctions of the device itself. We believe that the use of IVC filters among bariatric surgery patients should be discouraged.
Disclosure
This study was supported by a grant from the Agency for Healthcare Research and Quality (HS018050) and was presented at the Annual Meeting of the American Society for Metabolic and Bariatric Surgery (ASMBS), San Diego, California, June 20, 2012.
- Vena cava filters: a call to action. Chest Physician. 2011;16:18a. , , .
- U.S. Food and Drug Administration. Removing retrievable inferior vena cava filters: initial communication. August 9, 2010. Available at: http://www.fda.gov/MedicalDevices/Safety/AlertsandNotices/ucm221 676.htm. Accessed December 2, 2012.
- Preoperative placement of inferior vena cava filters and outcomes after gastric bypass surgery. Ann Surg. 2010;252:131–318. , , , et al.
- Prevalence of fracture and fragment embolization of Bard retrievable vena cava filters and clinical implications including cardiac perforation and tamponade. Arch Intern Med. 2010;170:1827–1831. , , , et al.
- Experience with inferior vena cava filter placement in patients undergoing open gastric bypass procedures. Ann Vasc Surg. 2006;44: 1301–1305. , , , , , .
- Preoperative placement of retrievable inferior vena cava filters in bariatric surgery. Surg Obes Relat Dis. 2007;3: 602–605. , .
- Safety and efficacy of intravascular ultrasound‐guided inferior vena cava filter in super obese bariatric patients. Surg Obes Relat Dis. 2008;4:50–54. , , , et al.
- Current indications for preoperative inferior vena cava filter insertion in patients undergoing surgery for morbid obesity. Obes Surg. 2005;15:1009–1012. , , , , , .
- Efficacy of prophylactic inferior vena cava filter placement in bariatric surgery. Surg Obes Relat Dis. 2007;3:606–610. , , , .
- Safety, feasibility, and outcome of retrievable vena cava filters in high‐risk surgical patients. J Vasc Surg. 2007;45:784–788. , , , et al.
- Retrievable inferior vena cava filters may be safely applied in gastric bypass surgery. Surg Endosc. 2007;21:2277–2279. , , , .
- Inferior vena cava filter placement for pulmonary embolism risk reduction in super morbidly obese undergoing bariatric surgery. Surg Obes Relat Dis. 2007;3:461–464. , , , , .
- A simple venous thromboembolism prophylaxis protocol for patients undergoing bariatric surgery. Obesity (Silver Spring). 2006;14:1961–1965. , .
- Risk‐group targeted inferior vena cava filter placemetn in gastric bypass patients. Obes Surg. 2009;19:451–455. , , , et al.
- Retreivable inferior vena cava filters in high‐risk patients undergoing bariatric surgery. Surg Endosc. 2009;23:2203–2207. , , , , , .
- Inferior vena caval filter insertion prior to bariatric surgery: A systematic review of the literature. J Thromb Haemost. 2010;8:1266–1270. , .
The use of inferior vena cava (IVC) filters has increased substantially in recent years. These medical devices, which are used to prevent pulmonary embolism in patients considered to be at high risk of venous thromboembolism, were placed in 167,000 patients in 2007.1 In 2012, it is estimated that 259,000 patients will undergo placement of an IVC filter, an increase of 55%.[1] Increasing use of IVC filters is attributable to the development of retrievable versions of the devices, which have expanded indications for use such as in bariatric surgery.
Unfortunately, the increase in the use of IVC filters has been accompanied by an increase in reports of adverse events in patients receiving them. The United States Food and Drug Administration (FDA) has received more than 900 adverse event reports involving IVC filters, prompting the agency to issue a warning about their use.[2] A prior study by our group demonstrated a lack of benefit of IVC filter insertion for the prevention of pulmonary embolism among bariatric surgery patients but lacked statistical power to prove harms associated with this practice.[3]
In the current study, we analyzed data from the prospective, statewide, clinical registry of the Michigan Bariatric Surgery Collaborative. Our study population now includes 35,477 bariatric surgery patients from 32 hospitals whose procedures were performed between 2006 and 2012. Since the publication of our prior study, the use of IVC filters in bariatric surgery has decreased significantly in Michigan. For this reason, our study population now includes many more high‐risk patients who did not undergo IVC filter placement, allowing us to match IVC filter patients to similarly high‐risk patients who did not receive IVC filters. We used these data to compare outcomes within 30 days of surgery, including rates of venous thromboembolism, overall serious complications, and death among patients who did and not receive IVC filters.
METHODS
Study Setting
The Michigan Bariatric Surgery Collaborative (MBSC) is a regional voluntary consortium of hospitals and surgeons that perform bariatric surgery in Michigan. The goal of the project is to improve the quality of care for patients undergoing bariatric surgery. To do this, the participating hospitals submit data to the MBSC clinical outcomes registry, patient survey, and surgeon survey databases. Three times per year the group meets to examine these data and to design and implement changes in care to improve the outcomes of care for bariatric patients. The project is funded by Blue Cross and Blue Shield of Michigan/Blue Care Network and coordinated by faculty and staff members from the Center for Healthcare Outcomes and Policy at the University of Michigan.
The MBSC held its first collaborative meeting in June 2005 and enrolled its first patient in June 2006. The MBSC now has the participation of all of the 32 bariatric programs in Michigan, enrolling approximately 6000 patients per year in its clinical registry. Participating hospitals submit data from a review of the medical records for all of their bariatric surgery patients. This review is conducted for each patient at 30 days after surgery. The information collected includes preoperative clinical characteristics and conditions as well as perioperative clinical care and outcomes. The medical record reviews are performed by centrally trained, nurse data abstractors using a standardized and validated instrument. Each participating hospital is site visited annually to verify the accuracy and completeness of their MBSC clinical registry data.
Study Population
This study includes data for 35,477 patients undergoing bariatric surgery, including: 9829 laparoscopic adjustable gastric band, 6068 sleeve gastrectomy, 19,141 gastric bypass, and 439 biliopancreatic diversion with duodenal switch procedures between June 2006 and September 2012. Patients undergoing revisional bariatric surgery were excluded from these analyses. Prior to surgery, 1077 (3.0%) of these patients had a prophylactic IVC filter placed for prevention of pulmonary embolism. Of the IVC filters placed, 39% were temporary IVC filters, 45% were permanent IVC filters, and the type of IVC filter was not known in 15%.
Baseline Clinical Characteristics
Data collected included patient demographic characteristics (age, gender, race, type of insurance), clinical characteristics (height, weight, history of cigarette smoking, mobility limitations), and obesity‐related and other comorbid conditions (lung disease, cardiovascular disease, hyperlipidemia, gastroesophageal reflux disease, peptic ulcer disease, cholelithiasis, urinary incontinence, renal disease, diabetes, liver disease, prior history of venous thromboembolism, sleep apnea, and psychological disorders).
Risk factors for VTE were empirically derived from our data base using multivariate statistical models. Risk factors for VTE included: age, body mass index, male sex, current or past smoking, mobility limitations, asthma, home oxygen use, peripheral vascular disease, prior history of VTE, bariatric procedure time, and procedure type. The baseline predicted risk for VTE was calculated for each patient based on these risk factors and was used to divide patients into low‐ (predicted risk <1%), medium‐ (predicted risk 1%2.5%), and high‐ (predicted risk 2.5%) risk groups. Among the 35,477 patients in the registry overall, 95% are in the low‐risk group, 4% are in the medium‐risk group, and 1% are in the high‐risk group. In the matched study cohorts, 69%, 22%, and 9% were in the high‐, medium‐, and low‐risk groups, respectively.
Medical Venous Thromboembolism Prophylaxis
Data were also collected regarding the type of medical venous thromboembolism prophylaxis (unfractionated vs low molecular weight heparin) used preoperatively, postoperatively, and whether the patient was discharged to home on low molecular weight heparin.
Outcomes
Our primary outcome measures included postoperative venous thromboembolism (deep vein thrombosis or pulmonary embolism requiring treatment). We also assessed overall rate of complications and complications according to severity as follows: non‐life threatening complications (surgical site infection including wound and port site infections treated with antibiotics and/or wound opening, anastomotic stricture requiring dilatation, bleeding requiring blood transfusion of <4 units, and pneumonia requiring treatment with antibiotics only); potentially life‐threatening complications (abdominal abscess requiring percutaneous drainage or reoperation, bowel obstruction requiring reoperation, leak requiring percutaneous drainage or reoperation, bleeding requiring transfusion >4 units, reoperation, or splenectomy, band‐related problems requiring reoperation, respiratory failure requiring 2 to 7 days intubation, renal failure requiring in‐hospital dialysis, wound infection/dehiscence requiring reoperation, and venous thromboembolism); and life‐threatening complications associated with residual and lasting disability or death (myocardial infarction or cardiac arrest, renal failure requiring long‐term dialysis, respiratory failure requiring >7 days intubation or tracheostomy, and death). Other complications that are not included in these categories (eg, IVC filter related) were assessed by an end points committee to determine their severity (non‐life threatening, potentially life threatening, or life threatening associated with residual and lasting disability or death).
Statistical Analyses
We used propensity score matching to assemble cohorts in which patients with and without IVC filters were balanced on baseline characteristics. The probability of IVC filter placement was estimated for each patient using a nonparsimonious multivariate logistic regression model, in which IVC filter was the dependent variable and all of the demographic, weight, medical history, weight‐related comorbidity, and procedure‐related variables (type, length, and year of procedure; and medical venous thromboembolism prophylaxis used) in our dataset were included as covariates. IVC filter patients were matched to control patients using a greedy, 1‐ to ‐1 matching without replacement protocol resulting in cohorts that were well balanced on all baseline characteristics.
Baseline characteristics and outcomes were then compared among the cohorts using [2] and t tests as appropriate. We used mixed effects logistic regression to compare outcomes between the 2 treatment groups while controlling for clustering at the hospital and surgeon level as random effects. Odds ratios (OR) and 95% confidence intervals (CI) were calculated to compare outcomes among patients with and without IVC filters.
RESULTS
Matching resulted in cohorts of IVC filter and control patients who were well balanced on all baseline characteristics (Table 1). In contrast, there were large and significant differences between IVC filter patients and unmatched control patients. For example, mean body mass index was 58 and 57 in the matched cohorts and 47 in the unmatched control patients. Prior history of venous thromboembolism was present in 39%, 39%, and 2% of the IVC filter, matched control, and unmatched control patients, respectively. With regard to procedure mix, unmatched control patients were less likely to have open gastric bypass and more likely to have adjustable gastric band procedures than IVC filter or matched control patients.
Variable | IVC Filter | Matched Controls | P Value | Unmatched Controls | P Value |
---|---|---|---|---|---|
| |||||
No. | 1077 | 1077 | 33,323 | ||
Age (mean, y) | 48 | 49 | 0.295 | 46 | <0.0001 |
Body mass index (mean, kg/m2) | 58 | 57 | 0.061 | 47 | <0.0001 |
Male gender (%) | 32 | 31 | 0.546 | 21 | <0.0001 |
Black race (%) | 27 | 25 | 0.667 | 15 | <0.0001 |
Private Insurance (%) | 62 | 64 | 0.305 | 74 | <0.0001 |
Smoking in past year (%) | 2 | 2 | 0.883 | 2 | 0.440 |
Mobility limitations (%) | 18 | 18 | 0.780 | 5 | <0.0001 |
Lung dsease (%) | 43 | 43 | 1.000 | 25 | <0.0001 |
Cardiovascular disease (%) | 21 | 21 | 0.874 | 10 | <0.0001 |
Hypertension (%) | 72 | 72 | 0.737 | 53 | <0.0001 |
Hyperlipidemia (%) | 59 | 59 | 0.930 | 50 | <0.0001 |
GERD (%) | 50 | 52 | 0.490 | 49 | 0.417 |
Peptic ulcer disease (%) | 5 | 4 | 0.228 | 3 | <0.0001 |
Cholelithiasis (%) | 30 | 30 | 0.963 | 27 | 0.018 |
Urinary incontinence (%) | 25 | 25 | 0.960 | 22 | 0.029 |
Renal failure (%) | 0.4 | 0.6 | 0.526 | 0.2 | 0.298 |
Diabetes (%) | 46 | 48 | 0.546 | 33 | <0.0001 |
Liver disorder (%) | 4 | 4 | 0.584 | 5 | 0.184 |
Prior history of VTE (%) | 39 | 39 | 0.965 | 2 | <0.0001 |
Sleep apnea (%) | 70 | 68 | 0.209 | 43 | <0.0001 |
Musculoskeletal disorder (%) | 78 | 80 | 0.221 | 77 | 0.189 |
History of hernia repair (%) | 5 | 6 | 0.924 | 3 | <0.0001 |
Psychological disorder (%) | 49 | 49 | 0.796 | 47 | 0.267 |
Total comorbidities (mean, no.) | 6 | 6 | 0.922 | 4 | <0.0001 |
Procedure | |||||
Adjustable gastric banding (%) | 15 | 17 | 0.099 | 29 | <0.0001 |
Sleeve gastrectomy (%) | 12 | 13 | 0.515 | 17 | <0.0001 |
Gastric bypass (%) | 73 | 69 | 0.058 | 53 | <0.0001 |
Duodenal switch (%) | 0.7 | 0.8 | 0.616 | 1.3 | <0.0001 |
Procedure Length (mean, minutes) | 114 | 116 | 0.427 | 95 | <0.0001 |
Medical VTE prophylaxis | |||||
Preoperative heparin: | |||||
Unfractionated (%) | 36 | 38 | 0.246 | 34 | 0.306 |
Low molecular weight (%) | 60 | 54 | 0.017 | 53 | <0.0001 |
Postoperative heparin: | |||||
Unfractionated (%) | 7 | 10 | 0.023 | 19 | <0.0001 |
Low molecular weight (%) | 70 | 68 | 0.326 | 64 | <0.0001 |
Postdischarge heparin: | |||||
Low molecular weight (%) | 72 | 66 | 0.003 | 16 | <0.0001 |
With regard to outcomes (Table 2, Figures 1 and 2), IVC filter patients had significantly higher rates of venous thromboembolism (1.9% vs 0.74%; OR, 2.7; 95% CI, 1.1‐6.3; P=0.027) and deep vein thrombosis (1.2% vs 0.37%, OR, 3.3; 95% CI, 1.1‐10.1; P=0.039) than matched control patients. Rates of pulmonary embolism were higher among IVC filter patients, but the difference was not statistically significant (0.84% vs 0.46%; OR, 2.0; 95% CI, 0.6‐6.5; P=0.232). Rates of pulmonary embolism were similar for patients with a low baseline risk of venous thromboembolism (0.27% vs 0.27%; OR, 1.0; 95% CI, 0.1‐7.7; P=0.965) but were higher for medium‐risk patients (2.1% vs 0.87%; OR, 2.5; 95% CI, 0.5‐12.7; P=0.288) and high‐risk patients (2.1% vs 0.97%; OR, 2.2; 95% CI, 0.2‐24.3; P=0.530).
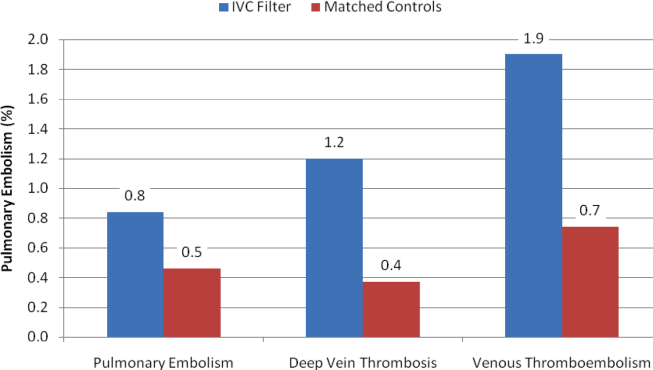
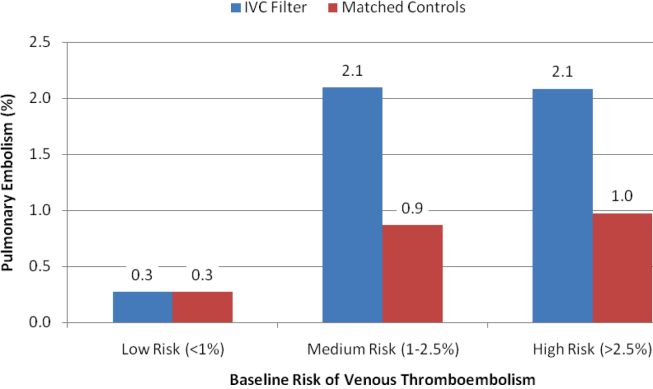
Outcomes | Odds Ratio | 95% Confidence Interval | P Value |
---|---|---|---|
Venous thromboembolism | 2.7 | 1.16.3 | 0.027 |
Deep vein thrombosis | 3.3 | 1.110.1 | 0.039 |
Pulmonary embolism | 2.0 | 0.66.5 | 0.232 |
Low‐risk subgroup | 1.0 | 0.17.7 | 0.965 |
Medium‐risk subgroup | 2.5 | 0.512.7 | 0.288 |
High‐risk subgroup | 2.2 | 0.224.3 | 0.530 |
Any complication | 1.3 | 1.01.7 | 0.048 |
Serious complication | 1.6 | 1.02.4 | 0.031 |
Permanently disabling complication | 4.3 | 1.215.6 | 0.028 |
Death | 7.0 | 0.957.3 | 0.068 |
Rates of other complications were also higher among IVC filter than matched control patients (Table 2 and Figure 3). There were significantly higher rates of complications (15.2% vs 11.6%; OR, 1.3; 95% CI, 1.0‐1.7; P=0.048), serious complications (5.8% vs 3.8%; OR, 1.6; 95% CI, 1.0‐2.4; P=0.031), and permanently disabling complications (1.2% vs 0.4%; OR, 4.3; 95% CI, 1.2‐15.6; P=0.028) among IVC filter patients. Rates of death (0.7% vs 0.1%; OR, 7.0; 95% CI, 0.9‐57.3; P=0.068) were also higher among IVC filter patients than matched control patients, but this difference was not statistically significant.
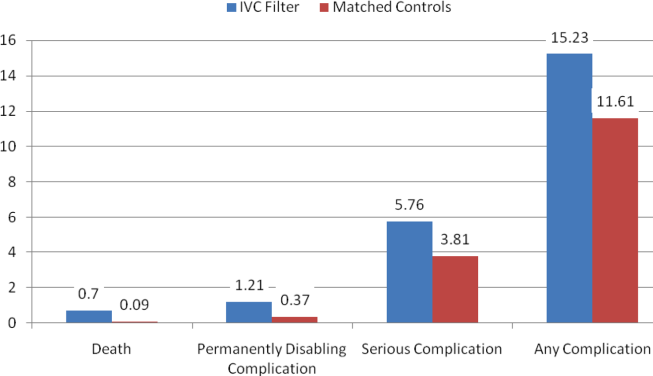
Among the 7 IVC filter patients who died, 4 had fatal pulmonary embolism, and 2 had IVC filter thrombosis/occlusion. Other IVC filter‐specific complications included IVC filter migration requiring heart valve replacement surgery in 1 patient, contrast‐induced nephropathy in 1 patient, IVC filter incision site infection in 1 patient, and technical difficulties removing a temporary IVC filter requiring that the device stay in place in 1 patient.
DISCUSSION
In this propensity matched, observational cohort study, we assessed the safety and effectiveness of prophylactic IVC filters among bariatric surgery patients. We found that patients with IVC filters had significantly worse outcomes than comparably high‐risk patients without IVC filters. Rates of venous thromboembolism were higher in the IVC filter patients, and a large proportion of the other complications among IVC filter patients were device related.
Our current study of IVC filters was prompted by an FDA advisory report regarding complications in patients receiving IVC filters.[2] The FDA's report was in turn prompted by a study indicating a high prevalence of strut fracture and embolization among 80 patients who received a certain type of retrievable IVC filter.[4] The FDA conveyed receiving 921 adverse events reports involving IVC filters between 2005 and 2009. Thirty‐six percent of these reports involved migration of the device, 16% were related to breakage and embolization of parts of the device, and 8% involved perforation of the IVC.
Research on the safety and efficacy of IVC filters in bariatric surgery patients has largely been limited to small, single‐center, case series or cohort studies.[5, 6, 7, 8, 9, 10, 11, 12, 13, 14, 15] A systematic review of this literature concluded that the evidence was insufficient to recommend IVC filters for patients undergoing bariatric surgery.[16] A 2010 study by our group was the largest and only multicenter study of IVC filters in the bariatric surgery population. We found no benefit of IVC filters in a comparison of 542 gastric bypass patients with prophylactic IVC filters to 5,834 gastric bypass patients without prophylactic IVC filters.[3]
When interpreting the results of this study, a number of limitations should be considered. Our study was observational, so there is the potential for unmeasured confounding variables to have influenced our results. To minimize the risk of confounding, we used propensity scores to match IVC filter patients to comparably high‐risk control patients, resulting in study cohorts that were well balanced on all baseline variables. Although this method accounts for confounding on the variables for which there are data, there is still the possibility that an unknown confounder could affect our findings. For example, our clinical registry lacks data on hypercoagulable states, so it is possible that a higher proportion of IVC filter patients could have had this risk factor and therefore a higher baseline risk of venous thromboembolism. However, most patients with a hypercoagulable state would have had a prior history of venous thromboembolism, which is a variable included in our database that patients were matched on.
The effects of changes in clinical care occurring during the time frame of this study should be considered in interpreting our findings. For example, bariatric surgery has been getting safer in general over time. Rates of death have fallen both in Michigan and in the rest of the country as bariatric surgeons have gained experience with this procedure. In Michigan during this time period, our group has developed and implemented a risk‐stratified, standardized approach to venous thromboembolism prophylaxis for patients undergoing bariatric surgery. For these reasons, we included the year of the procedure and the type of medical venous thromboembolism prophylaxis (unfractionated or low molecular weight heparin) used perioperatively as a matching variable in our analysis.
Another limitation that should be considered in interpreting our findings is statistical power. Although our study is the largest in this study population to date, many of the outcomes of interest are relatively rare. Considering the entire bariatric surgery population, rates of venous thromboembolism and death within 30 days are each less than 1%. Even in the high‐risk patients included in this analysis, there were a total of just 28 (1.3%) venous thromboembolism events and 8 (0.37) deaths. Nonetheless, our study did find significantly greater risks of multiple types of complications among patients receiving IVC filters.
Finally, our study captures events occurring within 30 days of bariatric surgery. Complications, including venous thromboembolism and other complications directly related to IVC filters, frequently occur after 30 days of bariatric surgery. Therefore, our study may be a conservative estimate of the risks associated with the use of IVC filters in bariatric surgery patients. Furthermore, certain brands of filters have been shown to be associated with higher risks of complications. Our study lacks data on the brand of IVC filter used and so cannot assess the extent to which this would affect our results.
CONCLUSIONS
In conclusion, our study indicates that IVC filters do not reduce the risk of pulmonary embolism in high‐risk bariatric surgery patients. They are also associated with other complications attributable to malfunctions of the device itself. We believe that the use of IVC filters among bariatric surgery patients should be discouraged.
Disclosure
This study was supported by a grant from the Agency for Healthcare Research and Quality (HS018050) and was presented at the Annual Meeting of the American Society for Metabolic and Bariatric Surgery (ASMBS), San Diego, California, June 20, 2012.
The use of inferior vena cava (IVC) filters has increased substantially in recent years. These medical devices, which are used to prevent pulmonary embolism in patients considered to be at high risk of venous thromboembolism, were placed in 167,000 patients in 2007.1 In 2012, it is estimated that 259,000 patients will undergo placement of an IVC filter, an increase of 55%.[1] Increasing use of IVC filters is attributable to the development of retrievable versions of the devices, which have expanded indications for use such as in bariatric surgery.
Unfortunately, the increase in the use of IVC filters has been accompanied by an increase in reports of adverse events in patients receiving them. The United States Food and Drug Administration (FDA) has received more than 900 adverse event reports involving IVC filters, prompting the agency to issue a warning about their use.[2] A prior study by our group demonstrated a lack of benefit of IVC filter insertion for the prevention of pulmonary embolism among bariatric surgery patients but lacked statistical power to prove harms associated with this practice.[3]
In the current study, we analyzed data from the prospective, statewide, clinical registry of the Michigan Bariatric Surgery Collaborative. Our study population now includes 35,477 bariatric surgery patients from 32 hospitals whose procedures were performed between 2006 and 2012. Since the publication of our prior study, the use of IVC filters in bariatric surgery has decreased significantly in Michigan. For this reason, our study population now includes many more high‐risk patients who did not undergo IVC filter placement, allowing us to match IVC filter patients to similarly high‐risk patients who did not receive IVC filters. We used these data to compare outcomes within 30 days of surgery, including rates of venous thromboembolism, overall serious complications, and death among patients who did and not receive IVC filters.
METHODS
Study Setting
The Michigan Bariatric Surgery Collaborative (MBSC) is a regional voluntary consortium of hospitals and surgeons that perform bariatric surgery in Michigan. The goal of the project is to improve the quality of care for patients undergoing bariatric surgery. To do this, the participating hospitals submit data to the MBSC clinical outcomes registry, patient survey, and surgeon survey databases. Three times per year the group meets to examine these data and to design and implement changes in care to improve the outcomes of care for bariatric patients. The project is funded by Blue Cross and Blue Shield of Michigan/Blue Care Network and coordinated by faculty and staff members from the Center for Healthcare Outcomes and Policy at the University of Michigan.
The MBSC held its first collaborative meeting in June 2005 and enrolled its first patient in June 2006. The MBSC now has the participation of all of the 32 bariatric programs in Michigan, enrolling approximately 6000 patients per year in its clinical registry. Participating hospitals submit data from a review of the medical records for all of their bariatric surgery patients. This review is conducted for each patient at 30 days after surgery. The information collected includes preoperative clinical characteristics and conditions as well as perioperative clinical care and outcomes. The medical record reviews are performed by centrally trained, nurse data abstractors using a standardized and validated instrument. Each participating hospital is site visited annually to verify the accuracy and completeness of their MBSC clinical registry data.
Study Population
This study includes data for 35,477 patients undergoing bariatric surgery, including: 9829 laparoscopic adjustable gastric band, 6068 sleeve gastrectomy, 19,141 gastric bypass, and 439 biliopancreatic diversion with duodenal switch procedures between June 2006 and September 2012. Patients undergoing revisional bariatric surgery were excluded from these analyses. Prior to surgery, 1077 (3.0%) of these patients had a prophylactic IVC filter placed for prevention of pulmonary embolism. Of the IVC filters placed, 39% were temporary IVC filters, 45% were permanent IVC filters, and the type of IVC filter was not known in 15%.
Baseline Clinical Characteristics
Data collected included patient demographic characteristics (age, gender, race, type of insurance), clinical characteristics (height, weight, history of cigarette smoking, mobility limitations), and obesity‐related and other comorbid conditions (lung disease, cardiovascular disease, hyperlipidemia, gastroesophageal reflux disease, peptic ulcer disease, cholelithiasis, urinary incontinence, renal disease, diabetes, liver disease, prior history of venous thromboembolism, sleep apnea, and psychological disorders).
Risk factors for VTE were empirically derived from our data base using multivariate statistical models. Risk factors for VTE included: age, body mass index, male sex, current or past smoking, mobility limitations, asthma, home oxygen use, peripheral vascular disease, prior history of VTE, bariatric procedure time, and procedure type. The baseline predicted risk for VTE was calculated for each patient based on these risk factors and was used to divide patients into low‐ (predicted risk <1%), medium‐ (predicted risk 1%2.5%), and high‐ (predicted risk 2.5%) risk groups. Among the 35,477 patients in the registry overall, 95% are in the low‐risk group, 4% are in the medium‐risk group, and 1% are in the high‐risk group. In the matched study cohorts, 69%, 22%, and 9% were in the high‐, medium‐, and low‐risk groups, respectively.
Medical Venous Thromboembolism Prophylaxis
Data were also collected regarding the type of medical venous thromboembolism prophylaxis (unfractionated vs low molecular weight heparin) used preoperatively, postoperatively, and whether the patient was discharged to home on low molecular weight heparin.
Outcomes
Our primary outcome measures included postoperative venous thromboembolism (deep vein thrombosis or pulmonary embolism requiring treatment). We also assessed overall rate of complications and complications according to severity as follows: non‐life threatening complications (surgical site infection including wound and port site infections treated with antibiotics and/or wound opening, anastomotic stricture requiring dilatation, bleeding requiring blood transfusion of <4 units, and pneumonia requiring treatment with antibiotics only); potentially life‐threatening complications (abdominal abscess requiring percutaneous drainage or reoperation, bowel obstruction requiring reoperation, leak requiring percutaneous drainage or reoperation, bleeding requiring transfusion >4 units, reoperation, or splenectomy, band‐related problems requiring reoperation, respiratory failure requiring 2 to 7 days intubation, renal failure requiring in‐hospital dialysis, wound infection/dehiscence requiring reoperation, and venous thromboembolism); and life‐threatening complications associated with residual and lasting disability or death (myocardial infarction or cardiac arrest, renal failure requiring long‐term dialysis, respiratory failure requiring >7 days intubation or tracheostomy, and death). Other complications that are not included in these categories (eg, IVC filter related) were assessed by an end points committee to determine their severity (non‐life threatening, potentially life threatening, or life threatening associated with residual and lasting disability or death).
Statistical Analyses
We used propensity score matching to assemble cohorts in which patients with and without IVC filters were balanced on baseline characteristics. The probability of IVC filter placement was estimated for each patient using a nonparsimonious multivariate logistic regression model, in which IVC filter was the dependent variable and all of the demographic, weight, medical history, weight‐related comorbidity, and procedure‐related variables (type, length, and year of procedure; and medical venous thromboembolism prophylaxis used) in our dataset were included as covariates. IVC filter patients were matched to control patients using a greedy, 1‐ to ‐1 matching without replacement protocol resulting in cohorts that were well balanced on all baseline characteristics.
Baseline characteristics and outcomes were then compared among the cohorts using [2] and t tests as appropriate. We used mixed effects logistic regression to compare outcomes between the 2 treatment groups while controlling for clustering at the hospital and surgeon level as random effects. Odds ratios (OR) and 95% confidence intervals (CI) were calculated to compare outcomes among patients with and without IVC filters.
RESULTS
Matching resulted in cohorts of IVC filter and control patients who were well balanced on all baseline characteristics (Table 1). In contrast, there were large and significant differences between IVC filter patients and unmatched control patients. For example, mean body mass index was 58 and 57 in the matched cohorts and 47 in the unmatched control patients. Prior history of venous thromboembolism was present in 39%, 39%, and 2% of the IVC filter, matched control, and unmatched control patients, respectively. With regard to procedure mix, unmatched control patients were less likely to have open gastric bypass and more likely to have adjustable gastric band procedures than IVC filter or matched control patients.
Variable | IVC Filter | Matched Controls | P Value | Unmatched Controls | P Value |
---|---|---|---|---|---|
| |||||
No. | 1077 | 1077 | 33,323 | ||
Age (mean, y) | 48 | 49 | 0.295 | 46 | <0.0001 |
Body mass index (mean, kg/m2) | 58 | 57 | 0.061 | 47 | <0.0001 |
Male gender (%) | 32 | 31 | 0.546 | 21 | <0.0001 |
Black race (%) | 27 | 25 | 0.667 | 15 | <0.0001 |
Private Insurance (%) | 62 | 64 | 0.305 | 74 | <0.0001 |
Smoking in past year (%) | 2 | 2 | 0.883 | 2 | 0.440 |
Mobility limitations (%) | 18 | 18 | 0.780 | 5 | <0.0001 |
Lung dsease (%) | 43 | 43 | 1.000 | 25 | <0.0001 |
Cardiovascular disease (%) | 21 | 21 | 0.874 | 10 | <0.0001 |
Hypertension (%) | 72 | 72 | 0.737 | 53 | <0.0001 |
Hyperlipidemia (%) | 59 | 59 | 0.930 | 50 | <0.0001 |
GERD (%) | 50 | 52 | 0.490 | 49 | 0.417 |
Peptic ulcer disease (%) | 5 | 4 | 0.228 | 3 | <0.0001 |
Cholelithiasis (%) | 30 | 30 | 0.963 | 27 | 0.018 |
Urinary incontinence (%) | 25 | 25 | 0.960 | 22 | 0.029 |
Renal failure (%) | 0.4 | 0.6 | 0.526 | 0.2 | 0.298 |
Diabetes (%) | 46 | 48 | 0.546 | 33 | <0.0001 |
Liver disorder (%) | 4 | 4 | 0.584 | 5 | 0.184 |
Prior history of VTE (%) | 39 | 39 | 0.965 | 2 | <0.0001 |
Sleep apnea (%) | 70 | 68 | 0.209 | 43 | <0.0001 |
Musculoskeletal disorder (%) | 78 | 80 | 0.221 | 77 | 0.189 |
History of hernia repair (%) | 5 | 6 | 0.924 | 3 | <0.0001 |
Psychological disorder (%) | 49 | 49 | 0.796 | 47 | 0.267 |
Total comorbidities (mean, no.) | 6 | 6 | 0.922 | 4 | <0.0001 |
Procedure | |||||
Adjustable gastric banding (%) | 15 | 17 | 0.099 | 29 | <0.0001 |
Sleeve gastrectomy (%) | 12 | 13 | 0.515 | 17 | <0.0001 |
Gastric bypass (%) | 73 | 69 | 0.058 | 53 | <0.0001 |
Duodenal switch (%) | 0.7 | 0.8 | 0.616 | 1.3 | <0.0001 |
Procedure Length (mean, minutes) | 114 | 116 | 0.427 | 95 | <0.0001 |
Medical VTE prophylaxis | |||||
Preoperative heparin: | |||||
Unfractionated (%) | 36 | 38 | 0.246 | 34 | 0.306 |
Low molecular weight (%) | 60 | 54 | 0.017 | 53 | <0.0001 |
Postoperative heparin: | |||||
Unfractionated (%) | 7 | 10 | 0.023 | 19 | <0.0001 |
Low molecular weight (%) | 70 | 68 | 0.326 | 64 | <0.0001 |
Postdischarge heparin: | |||||
Low molecular weight (%) | 72 | 66 | 0.003 | 16 | <0.0001 |
With regard to outcomes (Table 2, Figures 1 and 2), IVC filter patients had significantly higher rates of venous thromboembolism (1.9% vs 0.74%; OR, 2.7; 95% CI, 1.1‐6.3; P=0.027) and deep vein thrombosis (1.2% vs 0.37%, OR, 3.3; 95% CI, 1.1‐10.1; P=0.039) than matched control patients. Rates of pulmonary embolism were higher among IVC filter patients, but the difference was not statistically significant (0.84% vs 0.46%; OR, 2.0; 95% CI, 0.6‐6.5; P=0.232). Rates of pulmonary embolism were similar for patients with a low baseline risk of venous thromboembolism (0.27% vs 0.27%; OR, 1.0; 95% CI, 0.1‐7.7; P=0.965) but were higher for medium‐risk patients (2.1% vs 0.87%; OR, 2.5; 95% CI, 0.5‐12.7; P=0.288) and high‐risk patients (2.1% vs 0.97%; OR, 2.2; 95% CI, 0.2‐24.3; P=0.530).
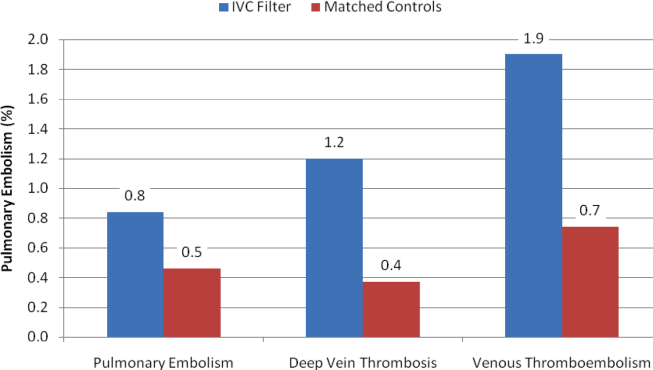
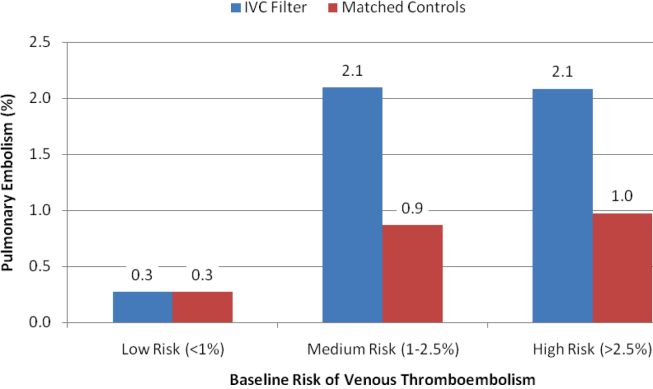
Outcomes | Odds Ratio | 95% Confidence Interval | P Value |
---|---|---|---|
Venous thromboembolism | 2.7 | 1.16.3 | 0.027 |
Deep vein thrombosis | 3.3 | 1.110.1 | 0.039 |
Pulmonary embolism | 2.0 | 0.66.5 | 0.232 |
Low‐risk subgroup | 1.0 | 0.17.7 | 0.965 |
Medium‐risk subgroup | 2.5 | 0.512.7 | 0.288 |
High‐risk subgroup | 2.2 | 0.224.3 | 0.530 |
Any complication | 1.3 | 1.01.7 | 0.048 |
Serious complication | 1.6 | 1.02.4 | 0.031 |
Permanently disabling complication | 4.3 | 1.215.6 | 0.028 |
Death | 7.0 | 0.957.3 | 0.068 |
Rates of other complications were also higher among IVC filter than matched control patients (Table 2 and Figure 3). There were significantly higher rates of complications (15.2% vs 11.6%; OR, 1.3; 95% CI, 1.0‐1.7; P=0.048), serious complications (5.8% vs 3.8%; OR, 1.6; 95% CI, 1.0‐2.4; P=0.031), and permanently disabling complications (1.2% vs 0.4%; OR, 4.3; 95% CI, 1.2‐15.6; P=0.028) among IVC filter patients. Rates of death (0.7% vs 0.1%; OR, 7.0; 95% CI, 0.9‐57.3; P=0.068) were also higher among IVC filter patients than matched control patients, but this difference was not statistically significant.
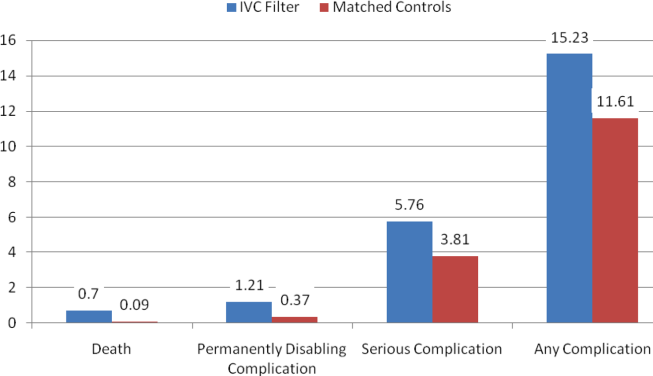
Among the 7 IVC filter patients who died, 4 had fatal pulmonary embolism, and 2 had IVC filter thrombosis/occlusion. Other IVC filter‐specific complications included IVC filter migration requiring heart valve replacement surgery in 1 patient, contrast‐induced nephropathy in 1 patient, IVC filter incision site infection in 1 patient, and technical difficulties removing a temporary IVC filter requiring that the device stay in place in 1 patient.
DISCUSSION
In this propensity matched, observational cohort study, we assessed the safety and effectiveness of prophylactic IVC filters among bariatric surgery patients. We found that patients with IVC filters had significantly worse outcomes than comparably high‐risk patients without IVC filters. Rates of venous thromboembolism were higher in the IVC filter patients, and a large proportion of the other complications among IVC filter patients were device related.
Our current study of IVC filters was prompted by an FDA advisory report regarding complications in patients receiving IVC filters.[2] The FDA's report was in turn prompted by a study indicating a high prevalence of strut fracture and embolization among 80 patients who received a certain type of retrievable IVC filter.[4] The FDA conveyed receiving 921 adverse events reports involving IVC filters between 2005 and 2009. Thirty‐six percent of these reports involved migration of the device, 16% were related to breakage and embolization of parts of the device, and 8% involved perforation of the IVC.
Research on the safety and efficacy of IVC filters in bariatric surgery patients has largely been limited to small, single‐center, case series or cohort studies.[5, 6, 7, 8, 9, 10, 11, 12, 13, 14, 15] A systematic review of this literature concluded that the evidence was insufficient to recommend IVC filters for patients undergoing bariatric surgery.[16] A 2010 study by our group was the largest and only multicenter study of IVC filters in the bariatric surgery population. We found no benefit of IVC filters in a comparison of 542 gastric bypass patients with prophylactic IVC filters to 5,834 gastric bypass patients without prophylactic IVC filters.[3]
When interpreting the results of this study, a number of limitations should be considered. Our study was observational, so there is the potential for unmeasured confounding variables to have influenced our results. To minimize the risk of confounding, we used propensity scores to match IVC filter patients to comparably high‐risk control patients, resulting in study cohorts that were well balanced on all baseline variables. Although this method accounts for confounding on the variables for which there are data, there is still the possibility that an unknown confounder could affect our findings. For example, our clinical registry lacks data on hypercoagulable states, so it is possible that a higher proportion of IVC filter patients could have had this risk factor and therefore a higher baseline risk of venous thromboembolism. However, most patients with a hypercoagulable state would have had a prior history of venous thromboembolism, which is a variable included in our database that patients were matched on.
The effects of changes in clinical care occurring during the time frame of this study should be considered in interpreting our findings. For example, bariatric surgery has been getting safer in general over time. Rates of death have fallen both in Michigan and in the rest of the country as bariatric surgeons have gained experience with this procedure. In Michigan during this time period, our group has developed and implemented a risk‐stratified, standardized approach to venous thromboembolism prophylaxis for patients undergoing bariatric surgery. For these reasons, we included the year of the procedure and the type of medical venous thromboembolism prophylaxis (unfractionated or low molecular weight heparin) used perioperatively as a matching variable in our analysis.
Another limitation that should be considered in interpreting our findings is statistical power. Although our study is the largest in this study population to date, many of the outcomes of interest are relatively rare. Considering the entire bariatric surgery population, rates of venous thromboembolism and death within 30 days are each less than 1%. Even in the high‐risk patients included in this analysis, there were a total of just 28 (1.3%) venous thromboembolism events and 8 (0.37) deaths. Nonetheless, our study did find significantly greater risks of multiple types of complications among patients receiving IVC filters.
Finally, our study captures events occurring within 30 days of bariatric surgery. Complications, including venous thromboembolism and other complications directly related to IVC filters, frequently occur after 30 days of bariatric surgery. Therefore, our study may be a conservative estimate of the risks associated with the use of IVC filters in bariatric surgery patients. Furthermore, certain brands of filters have been shown to be associated with higher risks of complications. Our study lacks data on the brand of IVC filter used and so cannot assess the extent to which this would affect our results.
CONCLUSIONS
In conclusion, our study indicates that IVC filters do not reduce the risk of pulmonary embolism in high‐risk bariatric surgery patients. They are also associated with other complications attributable to malfunctions of the device itself. We believe that the use of IVC filters among bariatric surgery patients should be discouraged.
Disclosure
This study was supported by a grant from the Agency for Healthcare Research and Quality (HS018050) and was presented at the Annual Meeting of the American Society for Metabolic and Bariatric Surgery (ASMBS), San Diego, California, June 20, 2012.
- Vena cava filters: a call to action. Chest Physician. 2011;16:18a. , , .
- U.S. Food and Drug Administration. Removing retrievable inferior vena cava filters: initial communication. August 9, 2010. Available at: http://www.fda.gov/MedicalDevices/Safety/AlertsandNotices/ucm221 676.htm. Accessed December 2, 2012.
- Preoperative placement of inferior vena cava filters and outcomes after gastric bypass surgery. Ann Surg. 2010;252:131–318. , , , et al.
- Prevalence of fracture and fragment embolization of Bard retrievable vena cava filters and clinical implications including cardiac perforation and tamponade. Arch Intern Med. 2010;170:1827–1831. , , , et al.
- Experience with inferior vena cava filter placement in patients undergoing open gastric bypass procedures. Ann Vasc Surg. 2006;44: 1301–1305. , , , , , .
- Preoperative placement of retrievable inferior vena cava filters in bariatric surgery. Surg Obes Relat Dis. 2007;3: 602–605. , .
- Safety and efficacy of intravascular ultrasound‐guided inferior vena cava filter in super obese bariatric patients. Surg Obes Relat Dis. 2008;4:50–54. , , , et al.
- Current indications for preoperative inferior vena cava filter insertion in patients undergoing surgery for morbid obesity. Obes Surg. 2005;15:1009–1012. , , , , , .
- Efficacy of prophylactic inferior vena cava filter placement in bariatric surgery. Surg Obes Relat Dis. 2007;3:606–610. , , , .
- Safety, feasibility, and outcome of retrievable vena cava filters in high‐risk surgical patients. J Vasc Surg. 2007;45:784–788. , , , et al.
- Retrievable inferior vena cava filters may be safely applied in gastric bypass surgery. Surg Endosc. 2007;21:2277–2279. , , , .
- Inferior vena cava filter placement for pulmonary embolism risk reduction in super morbidly obese undergoing bariatric surgery. Surg Obes Relat Dis. 2007;3:461–464. , , , , .
- A simple venous thromboembolism prophylaxis protocol for patients undergoing bariatric surgery. Obesity (Silver Spring). 2006;14:1961–1965. , .
- Risk‐group targeted inferior vena cava filter placemetn in gastric bypass patients. Obes Surg. 2009;19:451–455. , , , et al.
- Retreivable inferior vena cava filters in high‐risk patients undergoing bariatric surgery. Surg Endosc. 2009;23:2203–2207. , , , , , .
- Inferior vena caval filter insertion prior to bariatric surgery: A systematic review of the literature. J Thromb Haemost. 2010;8:1266–1270. , .
- Vena cava filters: a call to action. Chest Physician. 2011;16:18a. , , .
- U.S. Food and Drug Administration. Removing retrievable inferior vena cava filters: initial communication. August 9, 2010. Available at: http://www.fda.gov/MedicalDevices/Safety/AlertsandNotices/ucm221 676.htm. Accessed December 2, 2012.
- Preoperative placement of inferior vena cava filters and outcomes after gastric bypass surgery. Ann Surg. 2010;252:131–318. , , , et al.
- Prevalence of fracture and fragment embolization of Bard retrievable vena cava filters and clinical implications including cardiac perforation and tamponade. Arch Intern Med. 2010;170:1827–1831. , , , et al.
- Experience with inferior vena cava filter placement in patients undergoing open gastric bypass procedures. Ann Vasc Surg. 2006;44: 1301–1305. , , , , , .
- Preoperative placement of retrievable inferior vena cava filters in bariatric surgery. Surg Obes Relat Dis. 2007;3: 602–605. , .
- Safety and efficacy of intravascular ultrasound‐guided inferior vena cava filter in super obese bariatric patients. Surg Obes Relat Dis. 2008;4:50–54. , , , et al.
- Current indications for preoperative inferior vena cava filter insertion in patients undergoing surgery for morbid obesity. Obes Surg. 2005;15:1009–1012. , , , , , .
- Efficacy of prophylactic inferior vena cava filter placement in bariatric surgery. Surg Obes Relat Dis. 2007;3:606–610. , , , .
- Safety, feasibility, and outcome of retrievable vena cava filters in high‐risk surgical patients. J Vasc Surg. 2007;45:784–788. , , , et al.
- Retrievable inferior vena cava filters may be safely applied in gastric bypass surgery. Surg Endosc. 2007;21:2277–2279. , , , .
- Inferior vena cava filter placement for pulmonary embolism risk reduction in super morbidly obese undergoing bariatric surgery. Surg Obes Relat Dis. 2007;3:461–464. , , , , .
- A simple venous thromboembolism prophylaxis protocol for patients undergoing bariatric surgery. Obesity (Silver Spring). 2006;14:1961–1965. , .
- Risk‐group targeted inferior vena cava filter placemetn in gastric bypass patients. Obes Surg. 2009;19:451–455. , , , et al.
- Retreivable inferior vena cava filters in high‐risk patients undergoing bariatric surgery. Surg Endosc. 2009;23:2203–2207. , , , , , .
- Inferior vena caval filter insertion prior to bariatric surgery: A systematic review of the literature. J Thromb Haemost. 2010;8:1266–1270. , .
Copyright © 2013 Society of Hospital Medicine
Hospitalist Care and Patient Satisfaction
Payers and policymakers are increasingly holding hospitals accountable for patients' experiences with their care. Since 2006, the Centers for Medicare and Medicaid Services (CMS) have collected data on patients' experiences with inpatient care using the Hospital Consumer Assessment of Healthcare Providers and Systems (HCAHPS) survey, a well‐validated and widely used tool. In 2008, these data on patient experience began to be publicly reported, and CMS now plans to base part of its payments to hospitals on HCAHPS performance scores. In this context, hospitals are looking for ways to improve patient satisfaction.
The effort to hold hospitals accountable for patient experience may conflict with another major trend in US hospitals: the increasing use of hospitalists.[1] Although hospitalists may have greater expertise in the day‐to‐day care of the hospitalized patient, they generally do not know the patient and cannot cater to patients' preferences in ways that the primary‐care provider might. Therefore, given that patients may prefer to be seen by their primary‐care provider,[2] greater use of hospitalists may actually lead to a decrease in patient satisfaction. Unfortunately, we are unaware of any national examination of the relationship between hospitalist use in an institution and that entity's performance on patient‐experience scores.
To better understand the relationship between greater hospitalist staffing and patient‐centered care, we examined the association between hospitalist staffing and patient satisfaction with both overall care and specific domains of patient‐centered care. We hypothesized that hospitals that used a high proportion of hospitalists would generally have lower patient‐experience scores. Further, we expected that the relationship would be monotonic (greater use of hospitalists associated with lower scores) and particularly pronounced in 2 domains: patient experience with discharge planning and patient experience with physician communication.
METHODS
Data
We sought to identify acute‐care hospitals with elderly medical patients cared for by hospitalists, non‐hospitalists, or some combination of the 2. To construct this cohort, we used 3 2009 Medicare files. The Beneficiary Summary File contains demographic information on Medicare beneficiaries and data on enrollment in managed‐care plans. To identify medical hospitalizations, we used the Medicare Provider Analysis and Review (MedPAR) 100% Files, which contain the clinical diagnoses and payments for all fee‐for‐service Medicare beneficiaries discharged from acute‐care hospitals. To identify hospitalists and non‐hospitalists, we used the 5% Carrier File, which contains physician billing data for a 5% random sample of fee‐for‐service Medicare beneficiaries. We also obtained information on hospital characteristics from the American Hospital Association (AHA) Annual Survey. We supplemented this with hospital‐level data on patient satisfaction from the HCAHPS survey conducted in 2009. The HCAHPS is a standard survey developed by the Agency for Healthcare Research and Quality (AHRQ) and administered by hospitals to a random sample of adult patients 48 hours to 6 weeks after discharge. The HCAHPS results are adjusted for patient mix and have been tested for nonresponse bias.[3] Details about the development and design of HCAHPS have been described previously.[4]
Patient and Hospital Sample
We started with 48,861,000 Medicare beneficiaries in the Beneficiary Summary File and excluded 38% either because their age was <65 years or they were members of an HMO. At the same time, from the 1,850,000 patients in the 5% Carrier File, we excluded 55% who had not been cared for by a general internist. Finally, we used the MedPAR File to identify 17,387,000 hospital admissions by fee‐for‐service Medicare beneficiaries. From MedPAR, we excluded admissions to a facility other than an acute‐care hospital (24%), surgical admissions identified by diagnosis‐related group (DRG) (29%), and admissions to hospitals with <5 medicine admissions in 2009 (<0.1%). After merging these 3 files (Beneficiary Summary, MedPAR, and 5% Carrier), we were left with 229,496 admissions among 180,399 patients at 3365 hospitals. We subsequently excluded readmissions and were left with 156,333 admissions at 3244 hospitals. Finally, we excluded those patients cared for by both hospitalists and non‐hospitalists during the same hospitalization, and those hospitals missing AHA or HCAHPS data, leaving us with 132,814 patients at 2843 hospitals.
Definition of Hospitalist
We used the claims‐based definition developed and validated by Kuo and Goodwin in earlier work.[1] Hospitalists are defined as those general internists (providers in general practice or internal medicine) who had 5 evaluation and management (E&M) billings (in a 5% sample of Medicare beneficiaries) in 2009 and generated >90% of their claims from the care of hospitalized patients in 2009.
Measures of Patient Satisfaction
There are 2 HCAHPS questions about overall satisfaction, one that asks patients to rate their experience on a scale of 0 to 10 and another that asks whether they would recommend the hospital. Not surprisingly, hospitals' performance on these 2 questions is highly correlated.[5] We measured overall patient experience using commonly used approaches: the proportion of patients who gave the hospital a 9 or 10 (on the 10‐point scale) or the proportion of patients who reported that they would definitely recommend the hospital. The HCAHPS also contains 24 questions, which are reported by CMS in 8 domains: communication with nurse, communication with physician, responsiveness of the staff, pain control, communication about medications, adequacy of discharge planning, cleanliness of the room, and quietness of the room. The patient‐satisfaction score for each of these domains represents the proportion of patients who answered always to each of the questions, or who answered yes to the question about discharge.
Potentially Confounding Variables
Because we were worried that hospitals with hospitalists would be different from hospitals without hospitalists, we identified a series of covariates for adjustment in a multivariable model. We extracted data from the AHA on hospitals' structural characteristics that we assumed might be associated both with having a hospitalist and with patient experience. These variables were size (number of beds), teaching status (membership in the Council of Teaching Hospitals vs no membership), location (urban vs rural), region (the 4 census regions), ownership (for profit, private nonprofit, or public), and presence of advanced clinical capabilities (as measured by having a medical, surgical, and/or cardiac intensive care unit [ICU]). We also used information about the patient population (proportion of patients with Medicare or with Medicaid) as well as nurse‐staffing level (ratio of full‐time equivalent registered nurses to total hospital beds).
Statistical Analyses
We first quantified hospital variation in the proportion of general‐medicine patients cared for by hospitalists, using basic descriptive statistics. Based on these analyses, we categorized hospitals into 3 groups: non‐hospitalist, mixed, and hospitalist (corresponding to lowest, middle, and highest tertile of hospitalist use respectively). We used bivariate techniques to describe the patient and hospital characteristics of hospitals in each group. Patient characteristics included number of comorbidities, which were calculated using software from the Healthcare Cost and Utilization Project (HCUP),[6] based on methods developed by Elixhauser et al.[7] We used the ‐square test to assess whether hospital or patient characteristics differed between hospitalist, mixed, and non‐hospitalist hospitals.
To examine the association between hospitalist care and patient satisfaction, we first constructed bivariate models for each measure of patient satisfaction. In these models, hospital type (hospitalist, mixed, and non‐hospitalist) was our predictor. We next constructed multivariable models, which adjusted for each of the hospital characteristics described above in order to assess the independent relationship between hospitalist care and HCAHPS performance.
In sensitivity analyses, we first examined hospitalist use as a continuous variable and had qualitatively very similar results. Those data are not presented. Additionally, we conducted a propensity score analysis, with results presented in the Appendix (see Supporting Information, Appendix 1, in the online version of this article). In our first‐stage logistic regression model, being a hospitalist hospital (defined as being in the top tertile of hospitalist use vs bottom 2 tertiles) was the outcome. Hospital structural factors were covariates. Based on this first‐stage model, each hospital was assigned a propensity of being a hospitalist hospital. We divided the hospitals into 3 groups (highest propensity tertile, middle propensity tertile, and lowest propensity tertile). In a second‐stage linear regression model, patient satisfaction score was the outcome. The predictors were hospital type (dichotomized, and defined as being in the top tertile of hospitalist use vs bottom 2 tertiles), and propensity of being a hospitalist hospital (3 categories, with low propensity as the reference).
All analyses were performed using SAS version 9.2. The project was reviewed by the Institutional Review Board at the University of Michigan and determined to be not regulated given our use of publicly available datasets.
RESULTS
Among all hospitals, the median proportion of general‐medicine admissions cared for by hospitalists was 41.2% (interquartile range [IQR], 11.5%67.4%). However, US hospitals varied widely in the proportion of general‐medicine patients cared for by hospitalists (Figure 1). Whereas 3.5% of hospitals had all of their general‐medicine patients cared for by hospitalists, 16.6% had none of their general‐medicine patients seen by hospitalists. For hospitals with at least some hospitalist care, the proportion of patients cared for by hospitalists was distributed fairly evenly across the range of possibilities (Figure 1).
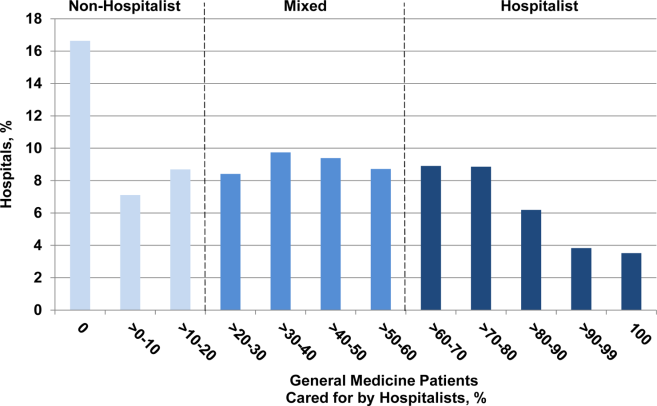
Because hospitalist care varied widely among hospitals, we categorized hospitals into 3 groups (non‐hospitalist, mixed, and hospitalist). The median proportion of patients cared for by hospitalists in the 3 groups was 0%, 39.5%, and 76.5%, respectively (Table 1). The non‐hospitalist hospitals, when compared with mixed and hospitalist hospitals, were more likely to be small, nonteaching, for‐profit institutions located in the Midwestern United States. They also were less likely to have an ICU and had lower nurse‐to‐bed ratios.
Hospital Characteristics | Hospital Type | P Value | ||
---|---|---|---|---|
Non‐Hospitalist (N = 943) | Mixed (N = 948) | Hospitalist (N = 952) | ||
| ||||
GM admissions cared for by hospitalists, median (range), % | 0 (021) | 40 (2158) | 77 (58100) | <0.001 |
Nurse‐to‐bed ratio | 1 | 1 | 2 | <0.001 |
Presence of MICU, % | 79 | 84 | 85 | 0.001 |
Medicaid patients, % | 19 | 18 | 18 | 0.06 |
Hospital beds, % | <0.001 | |||
Small (99) | 36 | 16 | 24 | |
Medium (100399) | 59 | 64 | 58 | |
Large (400) | 6 | 21 | 18 | |
COTH membership, % | <0.001 | |||
Yes | 3 | 13 | 11 | |
No | 97 | 87 | 89 | |
Urban, % | 0.10 | |||
Yes | 88 | 89 | 91 | |
No | 12 | 11 | 9 | |
Profit status, % | <0.001 | |||
For profit | 21 | 17 | 18 | |
Not for profit, private | 62 | 71 | 67 | |
Other | 18 | 12 | 15 | |
Region, % | <0.001 | |||
South | 41 | 42 | 42 | |
Northeast | 14 | 21 | 16 | |
Midwest | 30 | 22 | 18 | |
West | 15 | 15 | 24 |
The types of patients cared for at all 3 hospital types (non‐hospitalist, mixed, and hospitalist) were similar in age and day of admission (Table 2). Patients cared for at non‐hospitalist hospitals were slightly more likely to be female and non‐White, and less likely to be admitted from the emergency department or another hospital or healthcare facility.
Patient Characteristics | Hospital Type | P Value | ||
---|---|---|---|---|
Non‐Hospitalist (N = 33,265) | Mixed (N = 52,844) | Hospitalist (N = 46,705) | ||
| ||||
Age, y | 0.51 | |||
6574 | 27 | 27 | 27 | |
7584 | 39 | 39 | 39 | |
85 | 34 | 34 | 34 | |
Sex | <0.001 | |||
M | 35 | 35 | 36 | |
F | 65 | 65 | 64 | |
Race/ethnicity | <0.001 | |||
White | 85 | 85 | 87 | |
Black | 10 | 11 | 9 | |
Other | 5 | 4 | 4 | |
Unknown | 0 | 0 | 0 | |
Comorbidities, % | <0.001 | |||
0 | 8 | 8 | 7 | |
1 | 23 | 23 | 22 | |
2+ | 69 | 69 | 71 | |
Day of admission | 0.08 | |||
Weekday | 73 | 73 | 73 | |
Weekend | 27 | 27 | 27 | |
Admission source | <0.001 | |||
ED | 75 | 78 | 80 | |
Another ACH | 1 | 2 | 3 | |
Other healthcare facility | 4 | 4 | 4 | |
Other | 20 | 17 | 13 | |
ICU stay | <0.001 | |||
Yes | 13 | 12 | 12 | |
No | 87 | 88 | 88 | |
Length of stay, d | <0.001 | |||
Median (Q1, Q3) | 4 (3, 6) | 4 (2, 6) | 3 (2, 5) | |
DRG | <0.001 | |||
Septicemia or severe sepsis | 3 | 4 | 4 | |
Esophagitis, gastroenteritis | 3 | 3 | 3 | |
Kidney and urinary tract infections | 3 | 3 | 3 | |
Syncope | 3 | 3 | 3 | |
Pneumonia | 3 | 3 | 3 |
When we examined unadjusted relationships between type of hospital and patient experience, we found that patients at hospitalist vs non‐hospitalist hospitals were more likely to recommend the hospital (69.4% vs 65.1%: P < 0.001), and report higher overall satisfaction (65.9% vs 63.6%: P < 0.001) ((see Supporting Information, Appendix, Table A1, in the online version of this article)). Care at hospitalist hospitals was associated with higher satisfaction with discharge, but lower satisfaction with room cleanliness and communication with doctors. These differences were statistically significant at the P < 0.05 level.
When we examined the relationship between having more hospitalists and patient experience using multivariable models that accounted for differences in hospital characteristics, we found largely similar results: The proportion of patients who were satisfied with their overall care was still higher at hospitalist compared with non‐hospitalist hospitals (65.6% vs 63.9%: P < 0.001) (Figure 2). Similarly, patients were more likely to definitely recommend their hospital if they had been cared for at a hospitalist vs non‐hospitalist hospital (66.0% vs 63.4%: P < 0.001).
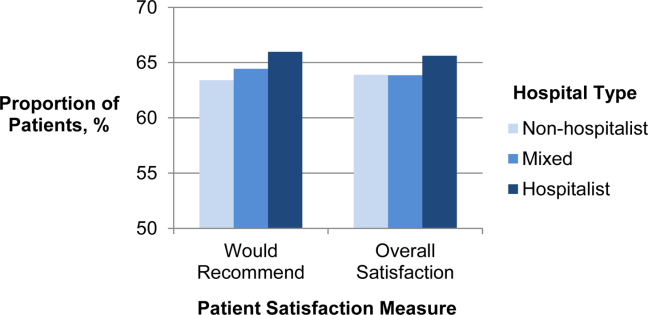
To better understand which domains of care might be contributing to greater overall satisfaction, we also examined patient satisfaction with specific domains of care at hospitalist vs non‐hospitalist hospitals (Table 3) in our adjusted analyses. Among 8 domains, the largest difference in satisfaction between patients cared for at hospitalist vs non‐hospitalist hospitals occurred with discharge. At hospitalist hospitals, 80.3% of patients said they were satisfied with the quality of the discharge planning compared with 78.1% at non‐hospitalist hospitals (P < 0.001). Patients at hospitalist hospitals were more satisfied with most other domains of care as well. Patients cared for at hospitalist hospitals were slightly less likely to be satisfied with communication with doctors, but this difference was not statistically significant (P = 0.45). Results were qualitatively similar in propensity‐score analyses (see Supporting Information, Appendix, Table A2, in the online version of this article).
Specific Domains of Care | Hospital Type, % Satisfied | Hospitalist vs Non‐Hospitalist | |||
---|---|---|---|---|---|
Non‐Hospitalist | Mixed | Hospitalist | Difference in % Satisfied | P Value | |
| |||||
Discharge | 78.1 | 79.1 | 80.3 | 2.1 | <0.001 |
Nursing services | 66.0 | 65.8 | 67.1 | 1.1 | <0.001 |
Quiet | 63.3 | 63.1 | 64.4 | 1.1 | 0.001 |
Communication, nurse | 76.7 | 76.7 | 77.7 | 1.0 | <0.001 |
Pain control | 69.7 | 69.7 | 70.4 | 0.7 | 0.001 |
Medications | 60.5 | 60.5 | 61.2 | 0.7 | 0.002 |
Cleanliness | 72.7 | 72.1 | 72.9 | 0.2 | 0.56 |
Communication, physician | 83.6 | 83.1 | 83.5 | 0.2 | 0.45 |
DISCUSSION
We found that in 2009, US hospitals varied widely in the proportion of general medicine patients cared for by hospitalists. Hospitals with higher levels of hospitalist care did better on most measures of patient satisfaction. Differences were largest in overall satisfaction and for discharge planning. In 5 other domains of care, differences were smaller, but hospitals with more hospitalist care consistently performed better than non‐hospitalist hospitals. Hospitalist care was not associated with patient satisfaction in 2 domains: communication with doctors and cleanliness of room.
Our findings of modestly higher patient satisfaction at hospitalist hospitals along most dimensions of care are surprising and reassuring. Indeed, when hospitalists first began caring for inpatients, some expressed concerns that hospitalist care would decrease patient satisfaction.[8, 9] Though this has been an ongoing concern, we found no evidence to support this contention. It may be that as a response to the concern, hospitals with hospitalists have paid particular attention to issues such as effective handoffs to primary‐care providers.[10, 11, 12, 13] Whether due to these efforts or other factors such as the 24/7 inpatient presence of hospitalists, we found that patients at hospitalist hospitals were more likely to be satisfied with their inpatient care, including their experience at discharge. In contrast, one area that may offer room for improvement for hospitalist hospitals is communication with physicians. It may be that patients cared for by hospitalists do not know their physicians as well as patients whose care is being orchestrated by their primary‐care provider, and thus the benefits of having an ever‐present hospitalist are diminished.
The magnitude of the associations that we found should also be placed in the context of existing research on patient satisfaction. Prior work has described baseline hospital performance, changes over time, and factors associated with greater inpatient satisfaction.[5, 14, 15] The associations that we found between hospitalist care and satisfaction with care at discharge were larger than those found for teaching (vs non‐teaching) hospitals.[5] However, compared with other hospital characteristics such as nurse staffing or profit status, hospitalist care was associated with smaller differences in patient satisfaction. In one study, hospitals in the highest quartile of nurse staffing had HCAHPS scores (ie, willingness to recommend measure) that were 6.7 points higher than those in the lowest quartile of nurse staffing, and similar differences existed between not‐for‐profit, public hospitals vs for‐profit hospitals.[5]
Taken together, our findings address an important gap in knowledge about hospitalist care. Prior research has documented growth in the use of hospitalist care[1] and described the association of hospitalist care with outcomes such as mortality and resource use, and receipt of recommended care.[16, 17, 18, 19] However, we are unaware of any national study that has examined the association of hospitalist care with patient satisfaction. One study surveyed patients in a single health system and found that patients were similarly satisfied with care provided by hospitalists and primary‐care physicians.[20] Our findings should be reassuring to clinical leaders and policymakers who have advocated greater use of hospitalists: the results suggest that there need be no tradeoff between greater use of hospitalist services and patient satisfaction. Indeed, patients appear to be even more satisfied in hospitals that have greater use of hospitalist physicians.
Our study has several limitations. First, it was a cross‐sectional study, and thus we cannot make any conclusions about causality. Although we adjusted for several potential confounders (eg, teaching status, advanced care capabilities, nurse staffing), it is possible that hospitalist care is a surrogate marker for features of hospitals that we could not measure but that directly influence patient experience. In addition, it is possible that patients cared for at hospitalist hospitals differ in unmeasured ways from patients cared for at other types of hospitals. Second, we constructed our primary predictor and outcome from different cohorts. Our primary predictor was derived from the proportion of general‐medicine patients cared for by hospitalists in Medicare claims data. In contrast, our primary outcome was based on HCAHPS responses from a random sampling of all hospital admissions. This misclassification likely would have biased us towards finding small or no associations. Therefore, we are likely underestimating the true association between hospitalist use and patient experience. Third, our findings may not be generalizable to hospitals that serve younger patients or have a large number of specialist hospitalists (who were not included in our definition of hospitalists). For example, compared with older patients with multiple comorbidities, relatively healthy younger patients may derive less benefit from an ever‐present hospitalist who can explain discharge plans or an attentive nurse.
In summary, we found that US hospitals varied widely in their use of hospitalist physicians, and those which a greater proportion of care was delivered by hospitalists generally had better scores on patient experience, especially on the global assessment of satisfaction and in discharge care. Our findings suggest that adoption of the hospitalist modelone of the strategies employed by US hospitals in the past 2 decades to provide efficient careshould not detract from achieving the goal of more patient‐centered care.
Disclosures
Dr. Chen's work is supported in part by the National Institutes of Health/National Institute on Aging (AG024824, University of Michigan Claude D. Pepper Older Americans Independence Center), and the National Institutes of Health/National Center for Research Resources (UL1‐RR024986, Michigan Institute for Clinical and Health Research). Dr. Chen is also supported by a Career Development Grant Award (K08HS020671) from the Agency for Healthcare Research and Quality.
- Growth in the care of older patients by hospitalists in the United States. N Engl J Med. 2009;360(11):1102–1112. , , , .
- How do patients view the role of the primary care physician in inpatient care? Dis Mon. 2002;48(4):230–238. , , .
- Effects of survey mode, patient mix, and nonresponse on CAHPS hospital survey scores. Health Serv Res. 2009;44(2 pt 1):501–518. , , , et al.
- Development, implementation, and public reporting of the HCAHPS survey. Med Care Res Rev. 2010;67(1):27–37. , , , , .
- Patients' perception of hospital care in the United States. N Engl J Med. 2008;359(18):1921–1931. , , , .
- Agency for Healthcare Research and Quality. Healthcare Cost and Utilization Project (HCUP). HCUP Comorbidity Software. http://www.hcup‐us.ahrq.gov/toolssoftware/comorbidity/comorbidity.jsp Accessed November 12, 2012.
- Comorbidity measures for use with administrative data. Med Care. 1998;36(1):8–27. , , , .
- Physician views on caring for hospitalized patients and the hospitalist model of inpatient care. J Gen Intern Med. 2001;16(2):116–119. , , .
- How physicians perceive hospitalist services after implementation: anticipation vs reality. Arch Intern Med. 2003;163(19):2330–2336. , , , .
- Association of communication between hospital‐based physicians and primary care providers with patient outcomes. J Gen Intern Med. 2009;24(3):381–386. , , , et al.
- Passing the clinical baton: 6 principles to guide the hospitalist. Dis Mon. 2002;48(4):260–266. , , .
- Primary care physician attitudes regarding communication with hospitalists. Am J Med. 2001;111(9B):15S–20S. , , , .
- Transitions of Care Consensus policy statement: American College of Physicians, Society of General Internal Medicine, Society of Hospital Medicine, American Geriatrics Society, American College Of Emergency Physicians, and Society for Academic Emergency Medicine. J Hosp Med. 2009;4(6):364–370. , , , et al.
- Hospital survey shows improvements in patient experience. Health Aff (Millwood). 2010;29(11):2061–2067. , , , et al.
- Characteristics of hospitals demonstrating superior performance in patient experience and clinical process measures of care. Med Care Res Rev. 2010;67(1):38–55. , , , , , .
- The impact of hospitalists on the cost and quality of inpatient care in the United States: a research synthesis. Med Care Res Rev. 2005;62(4):379–406. , .
- Quality of care for patients hospitalized with heart failure: assessing the impact of hospitalists. Arch Intern Med. 2002;162(11):1251–1256. , , , , .
- Hospitalists and the quality of care in hospitals. Arch Intern Med. 2009;169(15):1389–1394. , , , , .
- Quality of care for decompensated heart failure: comparable performance between academic hospitalists and non‐hospitalists. J Gen Intern Med. 2008;23(9):1399–1406. , , , et al.
- Patient satisfaction with hospital care provided by hospitalists and primary care physicians. J Hosp Med. 2012;7(2):131–136. , , , et al.
Payers and policymakers are increasingly holding hospitals accountable for patients' experiences with their care. Since 2006, the Centers for Medicare and Medicaid Services (CMS) have collected data on patients' experiences with inpatient care using the Hospital Consumer Assessment of Healthcare Providers and Systems (HCAHPS) survey, a well‐validated and widely used tool. In 2008, these data on patient experience began to be publicly reported, and CMS now plans to base part of its payments to hospitals on HCAHPS performance scores. In this context, hospitals are looking for ways to improve patient satisfaction.
The effort to hold hospitals accountable for patient experience may conflict with another major trend in US hospitals: the increasing use of hospitalists.[1] Although hospitalists may have greater expertise in the day‐to‐day care of the hospitalized patient, they generally do not know the patient and cannot cater to patients' preferences in ways that the primary‐care provider might. Therefore, given that patients may prefer to be seen by their primary‐care provider,[2] greater use of hospitalists may actually lead to a decrease in patient satisfaction. Unfortunately, we are unaware of any national examination of the relationship between hospitalist use in an institution and that entity's performance on patient‐experience scores.
To better understand the relationship between greater hospitalist staffing and patient‐centered care, we examined the association between hospitalist staffing and patient satisfaction with both overall care and specific domains of patient‐centered care. We hypothesized that hospitals that used a high proportion of hospitalists would generally have lower patient‐experience scores. Further, we expected that the relationship would be monotonic (greater use of hospitalists associated with lower scores) and particularly pronounced in 2 domains: patient experience with discharge planning and patient experience with physician communication.
METHODS
Data
We sought to identify acute‐care hospitals with elderly medical patients cared for by hospitalists, non‐hospitalists, or some combination of the 2. To construct this cohort, we used 3 2009 Medicare files. The Beneficiary Summary File contains demographic information on Medicare beneficiaries and data on enrollment in managed‐care plans. To identify medical hospitalizations, we used the Medicare Provider Analysis and Review (MedPAR) 100% Files, which contain the clinical diagnoses and payments for all fee‐for‐service Medicare beneficiaries discharged from acute‐care hospitals. To identify hospitalists and non‐hospitalists, we used the 5% Carrier File, which contains physician billing data for a 5% random sample of fee‐for‐service Medicare beneficiaries. We also obtained information on hospital characteristics from the American Hospital Association (AHA) Annual Survey. We supplemented this with hospital‐level data on patient satisfaction from the HCAHPS survey conducted in 2009. The HCAHPS is a standard survey developed by the Agency for Healthcare Research and Quality (AHRQ) and administered by hospitals to a random sample of adult patients 48 hours to 6 weeks after discharge. The HCAHPS results are adjusted for patient mix and have been tested for nonresponse bias.[3] Details about the development and design of HCAHPS have been described previously.[4]
Patient and Hospital Sample
We started with 48,861,000 Medicare beneficiaries in the Beneficiary Summary File and excluded 38% either because their age was <65 years or they were members of an HMO. At the same time, from the 1,850,000 patients in the 5% Carrier File, we excluded 55% who had not been cared for by a general internist. Finally, we used the MedPAR File to identify 17,387,000 hospital admissions by fee‐for‐service Medicare beneficiaries. From MedPAR, we excluded admissions to a facility other than an acute‐care hospital (24%), surgical admissions identified by diagnosis‐related group (DRG) (29%), and admissions to hospitals with <5 medicine admissions in 2009 (<0.1%). After merging these 3 files (Beneficiary Summary, MedPAR, and 5% Carrier), we were left with 229,496 admissions among 180,399 patients at 3365 hospitals. We subsequently excluded readmissions and were left with 156,333 admissions at 3244 hospitals. Finally, we excluded those patients cared for by both hospitalists and non‐hospitalists during the same hospitalization, and those hospitals missing AHA or HCAHPS data, leaving us with 132,814 patients at 2843 hospitals.
Definition of Hospitalist
We used the claims‐based definition developed and validated by Kuo and Goodwin in earlier work.[1] Hospitalists are defined as those general internists (providers in general practice or internal medicine) who had 5 evaluation and management (E&M) billings (in a 5% sample of Medicare beneficiaries) in 2009 and generated >90% of their claims from the care of hospitalized patients in 2009.
Measures of Patient Satisfaction
There are 2 HCAHPS questions about overall satisfaction, one that asks patients to rate their experience on a scale of 0 to 10 and another that asks whether they would recommend the hospital. Not surprisingly, hospitals' performance on these 2 questions is highly correlated.[5] We measured overall patient experience using commonly used approaches: the proportion of patients who gave the hospital a 9 or 10 (on the 10‐point scale) or the proportion of patients who reported that they would definitely recommend the hospital. The HCAHPS also contains 24 questions, which are reported by CMS in 8 domains: communication with nurse, communication with physician, responsiveness of the staff, pain control, communication about medications, adequacy of discharge planning, cleanliness of the room, and quietness of the room. The patient‐satisfaction score for each of these domains represents the proportion of patients who answered always to each of the questions, or who answered yes to the question about discharge.
Potentially Confounding Variables
Because we were worried that hospitals with hospitalists would be different from hospitals without hospitalists, we identified a series of covariates for adjustment in a multivariable model. We extracted data from the AHA on hospitals' structural characteristics that we assumed might be associated both with having a hospitalist and with patient experience. These variables were size (number of beds), teaching status (membership in the Council of Teaching Hospitals vs no membership), location (urban vs rural), region (the 4 census regions), ownership (for profit, private nonprofit, or public), and presence of advanced clinical capabilities (as measured by having a medical, surgical, and/or cardiac intensive care unit [ICU]). We also used information about the patient population (proportion of patients with Medicare or with Medicaid) as well as nurse‐staffing level (ratio of full‐time equivalent registered nurses to total hospital beds).
Statistical Analyses
We first quantified hospital variation in the proportion of general‐medicine patients cared for by hospitalists, using basic descriptive statistics. Based on these analyses, we categorized hospitals into 3 groups: non‐hospitalist, mixed, and hospitalist (corresponding to lowest, middle, and highest tertile of hospitalist use respectively). We used bivariate techniques to describe the patient and hospital characteristics of hospitals in each group. Patient characteristics included number of comorbidities, which were calculated using software from the Healthcare Cost and Utilization Project (HCUP),[6] based on methods developed by Elixhauser et al.[7] We used the ‐square test to assess whether hospital or patient characteristics differed between hospitalist, mixed, and non‐hospitalist hospitals.
To examine the association between hospitalist care and patient satisfaction, we first constructed bivariate models for each measure of patient satisfaction. In these models, hospital type (hospitalist, mixed, and non‐hospitalist) was our predictor. We next constructed multivariable models, which adjusted for each of the hospital characteristics described above in order to assess the independent relationship between hospitalist care and HCAHPS performance.
In sensitivity analyses, we first examined hospitalist use as a continuous variable and had qualitatively very similar results. Those data are not presented. Additionally, we conducted a propensity score analysis, with results presented in the Appendix (see Supporting Information, Appendix 1, in the online version of this article). In our first‐stage logistic regression model, being a hospitalist hospital (defined as being in the top tertile of hospitalist use vs bottom 2 tertiles) was the outcome. Hospital structural factors were covariates. Based on this first‐stage model, each hospital was assigned a propensity of being a hospitalist hospital. We divided the hospitals into 3 groups (highest propensity tertile, middle propensity tertile, and lowest propensity tertile). In a second‐stage linear regression model, patient satisfaction score was the outcome. The predictors were hospital type (dichotomized, and defined as being in the top tertile of hospitalist use vs bottom 2 tertiles), and propensity of being a hospitalist hospital (3 categories, with low propensity as the reference).
All analyses were performed using SAS version 9.2. The project was reviewed by the Institutional Review Board at the University of Michigan and determined to be not regulated given our use of publicly available datasets.
RESULTS
Among all hospitals, the median proportion of general‐medicine admissions cared for by hospitalists was 41.2% (interquartile range [IQR], 11.5%67.4%). However, US hospitals varied widely in the proportion of general‐medicine patients cared for by hospitalists (Figure 1). Whereas 3.5% of hospitals had all of their general‐medicine patients cared for by hospitalists, 16.6% had none of their general‐medicine patients seen by hospitalists. For hospitals with at least some hospitalist care, the proportion of patients cared for by hospitalists was distributed fairly evenly across the range of possibilities (Figure 1).
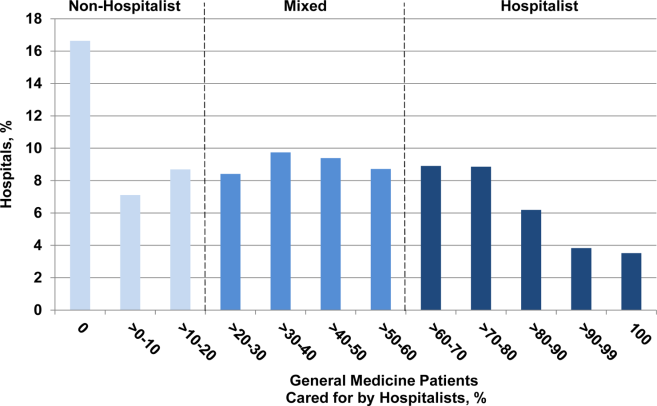
Because hospitalist care varied widely among hospitals, we categorized hospitals into 3 groups (non‐hospitalist, mixed, and hospitalist). The median proportion of patients cared for by hospitalists in the 3 groups was 0%, 39.5%, and 76.5%, respectively (Table 1). The non‐hospitalist hospitals, when compared with mixed and hospitalist hospitals, were more likely to be small, nonteaching, for‐profit institutions located in the Midwestern United States. They also were less likely to have an ICU and had lower nurse‐to‐bed ratios.
Hospital Characteristics | Hospital Type | P Value | ||
---|---|---|---|---|
Non‐Hospitalist (N = 943) | Mixed (N = 948) | Hospitalist (N = 952) | ||
| ||||
GM admissions cared for by hospitalists, median (range), % | 0 (021) | 40 (2158) | 77 (58100) | <0.001 |
Nurse‐to‐bed ratio | 1 | 1 | 2 | <0.001 |
Presence of MICU, % | 79 | 84 | 85 | 0.001 |
Medicaid patients, % | 19 | 18 | 18 | 0.06 |
Hospital beds, % | <0.001 | |||
Small (99) | 36 | 16 | 24 | |
Medium (100399) | 59 | 64 | 58 | |
Large (400) | 6 | 21 | 18 | |
COTH membership, % | <0.001 | |||
Yes | 3 | 13 | 11 | |
No | 97 | 87 | 89 | |
Urban, % | 0.10 | |||
Yes | 88 | 89 | 91 | |
No | 12 | 11 | 9 | |
Profit status, % | <0.001 | |||
For profit | 21 | 17 | 18 | |
Not for profit, private | 62 | 71 | 67 | |
Other | 18 | 12 | 15 | |
Region, % | <0.001 | |||
South | 41 | 42 | 42 | |
Northeast | 14 | 21 | 16 | |
Midwest | 30 | 22 | 18 | |
West | 15 | 15 | 24 |
The types of patients cared for at all 3 hospital types (non‐hospitalist, mixed, and hospitalist) were similar in age and day of admission (Table 2). Patients cared for at non‐hospitalist hospitals were slightly more likely to be female and non‐White, and less likely to be admitted from the emergency department or another hospital or healthcare facility.
Patient Characteristics | Hospital Type | P Value | ||
---|---|---|---|---|
Non‐Hospitalist (N = 33,265) | Mixed (N = 52,844) | Hospitalist (N = 46,705) | ||
| ||||
Age, y | 0.51 | |||
6574 | 27 | 27 | 27 | |
7584 | 39 | 39 | 39 | |
85 | 34 | 34 | 34 | |
Sex | <0.001 | |||
M | 35 | 35 | 36 | |
F | 65 | 65 | 64 | |
Race/ethnicity | <0.001 | |||
White | 85 | 85 | 87 | |
Black | 10 | 11 | 9 | |
Other | 5 | 4 | 4 | |
Unknown | 0 | 0 | 0 | |
Comorbidities, % | <0.001 | |||
0 | 8 | 8 | 7 | |
1 | 23 | 23 | 22 | |
2+ | 69 | 69 | 71 | |
Day of admission | 0.08 | |||
Weekday | 73 | 73 | 73 | |
Weekend | 27 | 27 | 27 | |
Admission source | <0.001 | |||
ED | 75 | 78 | 80 | |
Another ACH | 1 | 2 | 3 | |
Other healthcare facility | 4 | 4 | 4 | |
Other | 20 | 17 | 13 | |
ICU stay | <0.001 | |||
Yes | 13 | 12 | 12 | |
No | 87 | 88 | 88 | |
Length of stay, d | <0.001 | |||
Median (Q1, Q3) | 4 (3, 6) | 4 (2, 6) | 3 (2, 5) | |
DRG | <0.001 | |||
Septicemia or severe sepsis | 3 | 4 | 4 | |
Esophagitis, gastroenteritis | 3 | 3 | 3 | |
Kidney and urinary tract infections | 3 | 3 | 3 | |
Syncope | 3 | 3 | 3 | |
Pneumonia | 3 | 3 | 3 |
When we examined unadjusted relationships between type of hospital and patient experience, we found that patients at hospitalist vs non‐hospitalist hospitals were more likely to recommend the hospital (69.4% vs 65.1%: P < 0.001), and report higher overall satisfaction (65.9% vs 63.6%: P < 0.001) ((see Supporting Information, Appendix, Table A1, in the online version of this article)). Care at hospitalist hospitals was associated with higher satisfaction with discharge, but lower satisfaction with room cleanliness and communication with doctors. These differences were statistically significant at the P < 0.05 level.
When we examined the relationship between having more hospitalists and patient experience using multivariable models that accounted for differences in hospital characteristics, we found largely similar results: The proportion of patients who were satisfied with their overall care was still higher at hospitalist compared with non‐hospitalist hospitals (65.6% vs 63.9%: P < 0.001) (Figure 2). Similarly, patients were more likely to definitely recommend their hospital if they had been cared for at a hospitalist vs non‐hospitalist hospital (66.0% vs 63.4%: P < 0.001).
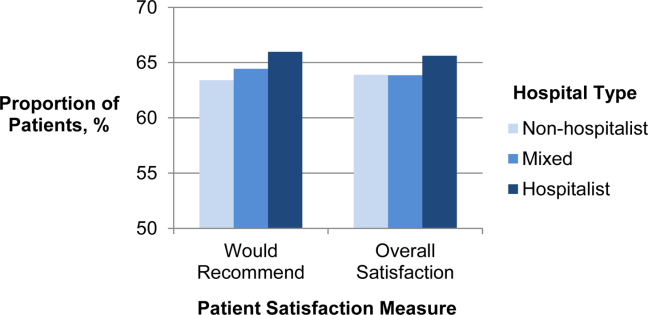
To better understand which domains of care might be contributing to greater overall satisfaction, we also examined patient satisfaction with specific domains of care at hospitalist vs non‐hospitalist hospitals (Table 3) in our adjusted analyses. Among 8 domains, the largest difference in satisfaction between patients cared for at hospitalist vs non‐hospitalist hospitals occurred with discharge. At hospitalist hospitals, 80.3% of patients said they were satisfied with the quality of the discharge planning compared with 78.1% at non‐hospitalist hospitals (P < 0.001). Patients at hospitalist hospitals were more satisfied with most other domains of care as well. Patients cared for at hospitalist hospitals were slightly less likely to be satisfied with communication with doctors, but this difference was not statistically significant (P = 0.45). Results were qualitatively similar in propensity‐score analyses (see Supporting Information, Appendix, Table A2, in the online version of this article).
Specific Domains of Care | Hospital Type, % Satisfied | Hospitalist vs Non‐Hospitalist | |||
---|---|---|---|---|---|
Non‐Hospitalist | Mixed | Hospitalist | Difference in % Satisfied | P Value | |
| |||||
Discharge | 78.1 | 79.1 | 80.3 | 2.1 | <0.001 |
Nursing services | 66.0 | 65.8 | 67.1 | 1.1 | <0.001 |
Quiet | 63.3 | 63.1 | 64.4 | 1.1 | 0.001 |
Communication, nurse | 76.7 | 76.7 | 77.7 | 1.0 | <0.001 |
Pain control | 69.7 | 69.7 | 70.4 | 0.7 | 0.001 |
Medications | 60.5 | 60.5 | 61.2 | 0.7 | 0.002 |
Cleanliness | 72.7 | 72.1 | 72.9 | 0.2 | 0.56 |
Communication, physician | 83.6 | 83.1 | 83.5 | 0.2 | 0.45 |
DISCUSSION
We found that in 2009, US hospitals varied widely in the proportion of general medicine patients cared for by hospitalists. Hospitals with higher levels of hospitalist care did better on most measures of patient satisfaction. Differences were largest in overall satisfaction and for discharge planning. In 5 other domains of care, differences were smaller, but hospitals with more hospitalist care consistently performed better than non‐hospitalist hospitals. Hospitalist care was not associated with patient satisfaction in 2 domains: communication with doctors and cleanliness of room.
Our findings of modestly higher patient satisfaction at hospitalist hospitals along most dimensions of care are surprising and reassuring. Indeed, when hospitalists first began caring for inpatients, some expressed concerns that hospitalist care would decrease patient satisfaction.[8, 9] Though this has been an ongoing concern, we found no evidence to support this contention. It may be that as a response to the concern, hospitals with hospitalists have paid particular attention to issues such as effective handoffs to primary‐care providers.[10, 11, 12, 13] Whether due to these efforts or other factors such as the 24/7 inpatient presence of hospitalists, we found that patients at hospitalist hospitals were more likely to be satisfied with their inpatient care, including their experience at discharge. In contrast, one area that may offer room for improvement for hospitalist hospitals is communication with physicians. It may be that patients cared for by hospitalists do not know their physicians as well as patients whose care is being orchestrated by their primary‐care provider, and thus the benefits of having an ever‐present hospitalist are diminished.
The magnitude of the associations that we found should also be placed in the context of existing research on patient satisfaction. Prior work has described baseline hospital performance, changes over time, and factors associated with greater inpatient satisfaction.[5, 14, 15] The associations that we found between hospitalist care and satisfaction with care at discharge were larger than those found for teaching (vs non‐teaching) hospitals.[5] However, compared with other hospital characteristics such as nurse staffing or profit status, hospitalist care was associated with smaller differences in patient satisfaction. In one study, hospitals in the highest quartile of nurse staffing had HCAHPS scores (ie, willingness to recommend measure) that were 6.7 points higher than those in the lowest quartile of nurse staffing, and similar differences existed between not‐for‐profit, public hospitals vs for‐profit hospitals.[5]
Taken together, our findings address an important gap in knowledge about hospitalist care. Prior research has documented growth in the use of hospitalist care[1] and described the association of hospitalist care with outcomes such as mortality and resource use, and receipt of recommended care.[16, 17, 18, 19] However, we are unaware of any national study that has examined the association of hospitalist care with patient satisfaction. One study surveyed patients in a single health system and found that patients were similarly satisfied with care provided by hospitalists and primary‐care physicians.[20] Our findings should be reassuring to clinical leaders and policymakers who have advocated greater use of hospitalists: the results suggest that there need be no tradeoff between greater use of hospitalist services and patient satisfaction. Indeed, patients appear to be even more satisfied in hospitals that have greater use of hospitalist physicians.
Our study has several limitations. First, it was a cross‐sectional study, and thus we cannot make any conclusions about causality. Although we adjusted for several potential confounders (eg, teaching status, advanced care capabilities, nurse staffing), it is possible that hospitalist care is a surrogate marker for features of hospitals that we could not measure but that directly influence patient experience. In addition, it is possible that patients cared for at hospitalist hospitals differ in unmeasured ways from patients cared for at other types of hospitals. Second, we constructed our primary predictor and outcome from different cohorts. Our primary predictor was derived from the proportion of general‐medicine patients cared for by hospitalists in Medicare claims data. In contrast, our primary outcome was based on HCAHPS responses from a random sampling of all hospital admissions. This misclassification likely would have biased us towards finding small or no associations. Therefore, we are likely underestimating the true association between hospitalist use and patient experience. Third, our findings may not be generalizable to hospitals that serve younger patients or have a large number of specialist hospitalists (who were not included in our definition of hospitalists). For example, compared with older patients with multiple comorbidities, relatively healthy younger patients may derive less benefit from an ever‐present hospitalist who can explain discharge plans or an attentive nurse.
In summary, we found that US hospitals varied widely in their use of hospitalist physicians, and those which a greater proportion of care was delivered by hospitalists generally had better scores on patient experience, especially on the global assessment of satisfaction and in discharge care. Our findings suggest that adoption of the hospitalist modelone of the strategies employed by US hospitals in the past 2 decades to provide efficient careshould not detract from achieving the goal of more patient‐centered care.
Disclosures
Dr. Chen's work is supported in part by the National Institutes of Health/National Institute on Aging (AG024824, University of Michigan Claude D. Pepper Older Americans Independence Center), and the National Institutes of Health/National Center for Research Resources (UL1‐RR024986, Michigan Institute for Clinical and Health Research). Dr. Chen is also supported by a Career Development Grant Award (K08HS020671) from the Agency for Healthcare Research and Quality.
Payers and policymakers are increasingly holding hospitals accountable for patients' experiences with their care. Since 2006, the Centers for Medicare and Medicaid Services (CMS) have collected data on patients' experiences with inpatient care using the Hospital Consumer Assessment of Healthcare Providers and Systems (HCAHPS) survey, a well‐validated and widely used tool. In 2008, these data on patient experience began to be publicly reported, and CMS now plans to base part of its payments to hospitals on HCAHPS performance scores. In this context, hospitals are looking for ways to improve patient satisfaction.
The effort to hold hospitals accountable for patient experience may conflict with another major trend in US hospitals: the increasing use of hospitalists.[1] Although hospitalists may have greater expertise in the day‐to‐day care of the hospitalized patient, they generally do not know the patient and cannot cater to patients' preferences in ways that the primary‐care provider might. Therefore, given that patients may prefer to be seen by their primary‐care provider,[2] greater use of hospitalists may actually lead to a decrease in patient satisfaction. Unfortunately, we are unaware of any national examination of the relationship between hospitalist use in an institution and that entity's performance on patient‐experience scores.
To better understand the relationship between greater hospitalist staffing and patient‐centered care, we examined the association between hospitalist staffing and patient satisfaction with both overall care and specific domains of patient‐centered care. We hypothesized that hospitals that used a high proportion of hospitalists would generally have lower patient‐experience scores. Further, we expected that the relationship would be monotonic (greater use of hospitalists associated with lower scores) and particularly pronounced in 2 domains: patient experience with discharge planning and patient experience with physician communication.
METHODS
Data
We sought to identify acute‐care hospitals with elderly medical patients cared for by hospitalists, non‐hospitalists, or some combination of the 2. To construct this cohort, we used 3 2009 Medicare files. The Beneficiary Summary File contains demographic information on Medicare beneficiaries and data on enrollment in managed‐care plans. To identify medical hospitalizations, we used the Medicare Provider Analysis and Review (MedPAR) 100% Files, which contain the clinical diagnoses and payments for all fee‐for‐service Medicare beneficiaries discharged from acute‐care hospitals. To identify hospitalists and non‐hospitalists, we used the 5% Carrier File, which contains physician billing data for a 5% random sample of fee‐for‐service Medicare beneficiaries. We also obtained information on hospital characteristics from the American Hospital Association (AHA) Annual Survey. We supplemented this with hospital‐level data on patient satisfaction from the HCAHPS survey conducted in 2009. The HCAHPS is a standard survey developed by the Agency for Healthcare Research and Quality (AHRQ) and administered by hospitals to a random sample of adult patients 48 hours to 6 weeks after discharge. The HCAHPS results are adjusted for patient mix and have been tested for nonresponse bias.[3] Details about the development and design of HCAHPS have been described previously.[4]
Patient and Hospital Sample
We started with 48,861,000 Medicare beneficiaries in the Beneficiary Summary File and excluded 38% either because their age was <65 years or they were members of an HMO. At the same time, from the 1,850,000 patients in the 5% Carrier File, we excluded 55% who had not been cared for by a general internist. Finally, we used the MedPAR File to identify 17,387,000 hospital admissions by fee‐for‐service Medicare beneficiaries. From MedPAR, we excluded admissions to a facility other than an acute‐care hospital (24%), surgical admissions identified by diagnosis‐related group (DRG) (29%), and admissions to hospitals with <5 medicine admissions in 2009 (<0.1%). After merging these 3 files (Beneficiary Summary, MedPAR, and 5% Carrier), we were left with 229,496 admissions among 180,399 patients at 3365 hospitals. We subsequently excluded readmissions and were left with 156,333 admissions at 3244 hospitals. Finally, we excluded those patients cared for by both hospitalists and non‐hospitalists during the same hospitalization, and those hospitals missing AHA or HCAHPS data, leaving us with 132,814 patients at 2843 hospitals.
Definition of Hospitalist
We used the claims‐based definition developed and validated by Kuo and Goodwin in earlier work.[1] Hospitalists are defined as those general internists (providers in general practice or internal medicine) who had 5 evaluation and management (E&M) billings (in a 5% sample of Medicare beneficiaries) in 2009 and generated >90% of their claims from the care of hospitalized patients in 2009.
Measures of Patient Satisfaction
There are 2 HCAHPS questions about overall satisfaction, one that asks patients to rate their experience on a scale of 0 to 10 and another that asks whether they would recommend the hospital. Not surprisingly, hospitals' performance on these 2 questions is highly correlated.[5] We measured overall patient experience using commonly used approaches: the proportion of patients who gave the hospital a 9 or 10 (on the 10‐point scale) or the proportion of patients who reported that they would definitely recommend the hospital. The HCAHPS also contains 24 questions, which are reported by CMS in 8 domains: communication with nurse, communication with physician, responsiveness of the staff, pain control, communication about medications, adequacy of discharge planning, cleanliness of the room, and quietness of the room. The patient‐satisfaction score for each of these domains represents the proportion of patients who answered always to each of the questions, or who answered yes to the question about discharge.
Potentially Confounding Variables
Because we were worried that hospitals with hospitalists would be different from hospitals without hospitalists, we identified a series of covariates for adjustment in a multivariable model. We extracted data from the AHA on hospitals' structural characteristics that we assumed might be associated both with having a hospitalist and with patient experience. These variables were size (number of beds), teaching status (membership in the Council of Teaching Hospitals vs no membership), location (urban vs rural), region (the 4 census regions), ownership (for profit, private nonprofit, or public), and presence of advanced clinical capabilities (as measured by having a medical, surgical, and/or cardiac intensive care unit [ICU]). We also used information about the patient population (proportion of patients with Medicare or with Medicaid) as well as nurse‐staffing level (ratio of full‐time equivalent registered nurses to total hospital beds).
Statistical Analyses
We first quantified hospital variation in the proportion of general‐medicine patients cared for by hospitalists, using basic descriptive statistics. Based on these analyses, we categorized hospitals into 3 groups: non‐hospitalist, mixed, and hospitalist (corresponding to lowest, middle, and highest tertile of hospitalist use respectively). We used bivariate techniques to describe the patient and hospital characteristics of hospitals in each group. Patient characteristics included number of comorbidities, which were calculated using software from the Healthcare Cost and Utilization Project (HCUP),[6] based on methods developed by Elixhauser et al.[7] We used the ‐square test to assess whether hospital or patient characteristics differed between hospitalist, mixed, and non‐hospitalist hospitals.
To examine the association between hospitalist care and patient satisfaction, we first constructed bivariate models for each measure of patient satisfaction. In these models, hospital type (hospitalist, mixed, and non‐hospitalist) was our predictor. We next constructed multivariable models, which adjusted for each of the hospital characteristics described above in order to assess the independent relationship between hospitalist care and HCAHPS performance.
In sensitivity analyses, we first examined hospitalist use as a continuous variable and had qualitatively very similar results. Those data are not presented. Additionally, we conducted a propensity score analysis, with results presented in the Appendix (see Supporting Information, Appendix 1, in the online version of this article). In our first‐stage logistic regression model, being a hospitalist hospital (defined as being in the top tertile of hospitalist use vs bottom 2 tertiles) was the outcome. Hospital structural factors were covariates. Based on this first‐stage model, each hospital was assigned a propensity of being a hospitalist hospital. We divided the hospitals into 3 groups (highest propensity tertile, middle propensity tertile, and lowest propensity tertile). In a second‐stage linear regression model, patient satisfaction score was the outcome. The predictors were hospital type (dichotomized, and defined as being in the top tertile of hospitalist use vs bottom 2 tertiles), and propensity of being a hospitalist hospital (3 categories, with low propensity as the reference).
All analyses were performed using SAS version 9.2. The project was reviewed by the Institutional Review Board at the University of Michigan and determined to be not regulated given our use of publicly available datasets.
RESULTS
Among all hospitals, the median proportion of general‐medicine admissions cared for by hospitalists was 41.2% (interquartile range [IQR], 11.5%67.4%). However, US hospitals varied widely in the proportion of general‐medicine patients cared for by hospitalists (Figure 1). Whereas 3.5% of hospitals had all of their general‐medicine patients cared for by hospitalists, 16.6% had none of their general‐medicine patients seen by hospitalists. For hospitals with at least some hospitalist care, the proportion of patients cared for by hospitalists was distributed fairly evenly across the range of possibilities (Figure 1).
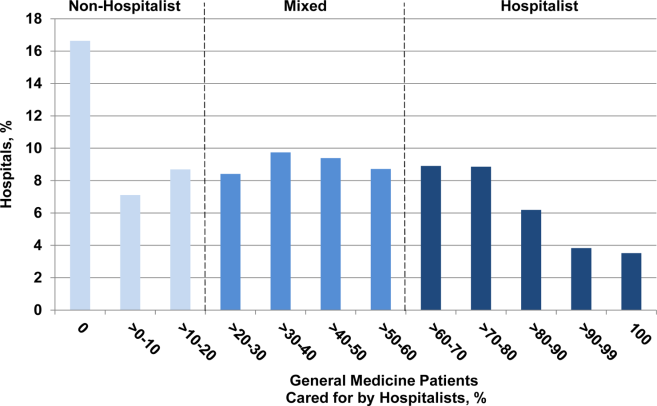
Because hospitalist care varied widely among hospitals, we categorized hospitals into 3 groups (non‐hospitalist, mixed, and hospitalist). The median proportion of patients cared for by hospitalists in the 3 groups was 0%, 39.5%, and 76.5%, respectively (Table 1). The non‐hospitalist hospitals, when compared with mixed and hospitalist hospitals, were more likely to be small, nonteaching, for‐profit institutions located in the Midwestern United States. They also were less likely to have an ICU and had lower nurse‐to‐bed ratios.
Hospital Characteristics | Hospital Type | P Value | ||
---|---|---|---|---|
Non‐Hospitalist (N = 943) | Mixed (N = 948) | Hospitalist (N = 952) | ||
| ||||
GM admissions cared for by hospitalists, median (range), % | 0 (021) | 40 (2158) | 77 (58100) | <0.001 |
Nurse‐to‐bed ratio | 1 | 1 | 2 | <0.001 |
Presence of MICU, % | 79 | 84 | 85 | 0.001 |
Medicaid patients, % | 19 | 18 | 18 | 0.06 |
Hospital beds, % | <0.001 | |||
Small (99) | 36 | 16 | 24 | |
Medium (100399) | 59 | 64 | 58 | |
Large (400) | 6 | 21 | 18 | |
COTH membership, % | <0.001 | |||
Yes | 3 | 13 | 11 | |
No | 97 | 87 | 89 | |
Urban, % | 0.10 | |||
Yes | 88 | 89 | 91 | |
No | 12 | 11 | 9 | |
Profit status, % | <0.001 | |||
For profit | 21 | 17 | 18 | |
Not for profit, private | 62 | 71 | 67 | |
Other | 18 | 12 | 15 | |
Region, % | <0.001 | |||
South | 41 | 42 | 42 | |
Northeast | 14 | 21 | 16 | |
Midwest | 30 | 22 | 18 | |
West | 15 | 15 | 24 |
The types of patients cared for at all 3 hospital types (non‐hospitalist, mixed, and hospitalist) were similar in age and day of admission (Table 2). Patients cared for at non‐hospitalist hospitals were slightly more likely to be female and non‐White, and less likely to be admitted from the emergency department or another hospital or healthcare facility.
Patient Characteristics | Hospital Type | P Value | ||
---|---|---|---|---|
Non‐Hospitalist (N = 33,265) | Mixed (N = 52,844) | Hospitalist (N = 46,705) | ||
| ||||
Age, y | 0.51 | |||
6574 | 27 | 27 | 27 | |
7584 | 39 | 39 | 39 | |
85 | 34 | 34 | 34 | |
Sex | <0.001 | |||
M | 35 | 35 | 36 | |
F | 65 | 65 | 64 | |
Race/ethnicity | <0.001 | |||
White | 85 | 85 | 87 | |
Black | 10 | 11 | 9 | |
Other | 5 | 4 | 4 | |
Unknown | 0 | 0 | 0 | |
Comorbidities, % | <0.001 | |||
0 | 8 | 8 | 7 | |
1 | 23 | 23 | 22 | |
2+ | 69 | 69 | 71 | |
Day of admission | 0.08 | |||
Weekday | 73 | 73 | 73 | |
Weekend | 27 | 27 | 27 | |
Admission source | <0.001 | |||
ED | 75 | 78 | 80 | |
Another ACH | 1 | 2 | 3 | |
Other healthcare facility | 4 | 4 | 4 | |
Other | 20 | 17 | 13 | |
ICU stay | <0.001 | |||
Yes | 13 | 12 | 12 | |
No | 87 | 88 | 88 | |
Length of stay, d | <0.001 | |||
Median (Q1, Q3) | 4 (3, 6) | 4 (2, 6) | 3 (2, 5) | |
DRG | <0.001 | |||
Septicemia or severe sepsis | 3 | 4 | 4 | |
Esophagitis, gastroenteritis | 3 | 3 | 3 | |
Kidney and urinary tract infections | 3 | 3 | 3 | |
Syncope | 3 | 3 | 3 | |
Pneumonia | 3 | 3 | 3 |
When we examined unadjusted relationships between type of hospital and patient experience, we found that patients at hospitalist vs non‐hospitalist hospitals were more likely to recommend the hospital (69.4% vs 65.1%: P < 0.001), and report higher overall satisfaction (65.9% vs 63.6%: P < 0.001) ((see Supporting Information, Appendix, Table A1, in the online version of this article)). Care at hospitalist hospitals was associated with higher satisfaction with discharge, but lower satisfaction with room cleanliness and communication with doctors. These differences were statistically significant at the P < 0.05 level.
When we examined the relationship between having more hospitalists and patient experience using multivariable models that accounted for differences in hospital characteristics, we found largely similar results: The proportion of patients who were satisfied with their overall care was still higher at hospitalist compared with non‐hospitalist hospitals (65.6% vs 63.9%: P < 0.001) (Figure 2). Similarly, patients were more likely to definitely recommend their hospital if they had been cared for at a hospitalist vs non‐hospitalist hospital (66.0% vs 63.4%: P < 0.001).
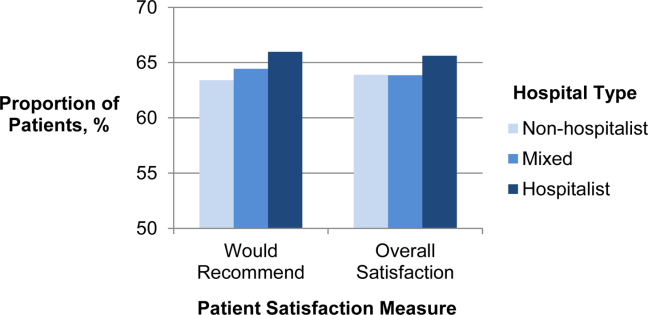
To better understand which domains of care might be contributing to greater overall satisfaction, we also examined patient satisfaction with specific domains of care at hospitalist vs non‐hospitalist hospitals (Table 3) in our adjusted analyses. Among 8 domains, the largest difference in satisfaction between patients cared for at hospitalist vs non‐hospitalist hospitals occurred with discharge. At hospitalist hospitals, 80.3% of patients said they were satisfied with the quality of the discharge planning compared with 78.1% at non‐hospitalist hospitals (P < 0.001). Patients at hospitalist hospitals were more satisfied with most other domains of care as well. Patients cared for at hospitalist hospitals were slightly less likely to be satisfied with communication with doctors, but this difference was not statistically significant (P = 0.45). Results were qualitatively similar in propensity‐score analyses (see Supporting Information, Appendix, Table A2, in the online version of this article).
Specific Domains of Care | Hospital Type, % Satisfied | Hospitalist vs Non‐Hospitalist | |||
---|---|---|---|---|---|
Non‐Hospitalist | Mixed | Hospitalist | Difference in % Satisfied | P Value | |
| |||||
Discharge | 78.1 | 79.1 | 80.3 | 2.1 | <0.001 |
Nursing services | 66.0 | 65.8 | 67.1 | 1.1 | <0.001 |
Quiet | 63.3 | 63.1 | 64.4 | 1.1 | 0.001 |
Communication, nurse | 76.7 | 76.7 | 77.7 | 1.0 | <0.001 |
Pain control | 69.7 | 69.7 | 70.4 | 0.7 | 0.001 |
Medications | 60.5 | 60.5 | 61.2 | 0.7 | 0.002 |
Cleanliness | 72.7 | 72.1 | 72.9 | 0.2 | 0.56 |
Communication, physician | 83.6 | 83.1 | 83.5 | 0.2 | 0.45 |
DISCUSSION
We found that in 2009, US hospitals varied widely in the proportion of general medicine patients cared for by hospitalists. Hospitals with higher levels of hospitalist care did better on most measures of patient satisfaction. Differences were largest in overall satisfaction and for discharge planning. In 5 other domains of care, differences were smaller, but hospitals with more hospitalist care consistently performed better than non‐hospitalist hospitals. Hospitalist care was not associated with patient satisfaction in 2 domains: communication with doctors and cleanliness of room.
Our findings of modestly higher patient satisfaction at hospitalist hospitals along most dimensions of care are surprising and reassuring. Indeed, when hospitalists first began caring for inpatients, some expressed concerns that hospitalist care would decrease patient satisfaction.[8, 9] Though this has been an ongoing concern, we found no evidence to support this contention. It may be that as a response to the concern, hospitals with hospitalists have paid particular attention to issues such as effective handoffs to primary‐care providers.[10, 11, 12, 13] Whether due to these efforts or other factors such as the 24/7 inpatient presence of hospitalists, we found that patients at hospitalist hospitals were more likely to be satisfied with their inpatient care, including their experience at discharge. In contrast, one area that may offer room for improvement for hospitalist hospitals is communication with physicians. It may be that patients cared for by hospitalists do not know their physicians as well as patients whose care is being orchestrated by their primary‐care provider, and thus the benefits of having an ever‐present hospitalist are diminished.
The magnitude of the associations that we found should also be placed in the context of existing research on patient satisfaction. Prior work has described baseline hospital performance, changes over time, and factors associated with greater inpatient satisfaction.[5, 14, 15] The associations that we found between hospitalist care and satisfaction with care at discharge were larger than those found for teaching (vs non‐teaching) hospitals.[5] However, compared with other hospital characteristics such as nurse staffing or profit status, hospitalist care was associated with smaller differences in patient satisfaction. In one study, hospitals in the highest quartile of nurse staffing had HCAHPS scores (ie, willingness to recommend measure) that were 6.7 points higher than those in the lowest quartile of nurse staffing, and similar differences existed between not‐for‐profit, public hospitals vs for‐profit hospitals.[5]
Taken together, our findings address an important gap in knowledge about hospitalist care. Prior research has documented growth in the use of hospitalist care[1] and described the association of hospitalist care with outcomes such as mortality and resource use, and receipt of recommended care.[16, 17, 18, 19] However, we are unaware of any national study that has examined the association of hospitalist care with patient satisfaction. One study surveyed patients in a single health system and found that patients were similarly satisfied with care provided by hospitalists and primary‐care physicians.[20] Our findings should be reassuring to clinical leaders and policymakers who have advocated greater use of hospitalists: the results suggest that there need be no tradeoff between greater use of hospitalist services and patient satisfaction. Indeed, patients appear to be even more satisfied in hospitals that have greater use of hospitalist physicians.
Our study has several limitations. First, it was a cross‐sectional study, and thus we cannot make any conclusions about causality. Although we adjusted for several potential confounders (eg, teaching status, advanced care capabilities, nurse staffing), it is possible that hospitalist care is a surrogate marker for features of hospitals that we could not measure but that directly influence patient experience. In addition, it is possible that patients cared for at hospitalist hospitals differ in unmeasured ways from patients cared for at other types of hospitals. Second, we constructed our primary predictor and outcome from different cohorts. Our primary predictor was derived from the proportion of general‐medicine patients cared for by hospitalists in Medicare claims data. In contrast, our primary outcome was based on HCAHPS responses from a random sampling of all hospital admissions. This misclassification likely would have biased us towards finding small or no associations. Therefore, we are likely underestimating the true association between hospitalist use and patient experience. Third, our findings may not be generalizable to hospitals that serve younger patients or have a large number of specialist hospitalists (who were not included in our definition of hospitalists). For example, compared with older patients with multiple comorbidities, relatively healthy younger patients may derive less benefit from an ever‐present hospitalist who can explain discharge plans or an attentive nurse.
In summary, we found that US hospitals varied widely in their use of hospitalist physicians, and those which a greater proportion of care was delivered by hospitalists generally had better scores on patient experience, especially on the global assessment of satisfaction and in discharge care. Our findings suggest that adoption of the hospitalist modelone of the strategies employed by US hospitals in the past 2 decades to provide efficient careshould not detract from achieving the goal of more patient‐centered care.
Disclosures
Dr. Chen's work is supported in part by the National Institutes of Health/National Institute on Aging (AG024824, University of Michigan Claude D. Pepper Older Americans Independence Center), and the National Institutes of Health/National Center for Research Resources (UL1‐RR024986, Michigan Institute for Clinical and Health Research). Dr. Chen is also supported by a Career Development Grant Award (K08HS020671) from the Agency for Healthcare Research and Quality.
- Growth in the care of older patients by hospitalists in the United States. N Engl J Med. 2009;360(11):1102–1112. , , , .
- How do patients view the role of the primary care physician in inpatient care? Dis Mon. 2002;48(4):230–238. , , .
- Effects of survey mode, patient mix, and nonresponse on CAHPS hospital survey scores. Health Serv Res. 2009;44(2 pt 1):501–518. , , , et al.
- Development, implementation, and public reporting of the HCAHPS survey. Med Care Res Rev. 2010;67(1):27–37. , , , , .
- Patients' perception of hospital care in the United States. N Engl J Med. 2008;359(18):1921–1931. , , , .
- Agency for Healthcare Research and Quality. Healthcare Cost and Utilization Project (HCUP). HCUP Comorbidity Software. http://www.hcup‐us.ahrq.gov/toolssoftware/comorbidity/comorbidity.jsp Accessed November 12, 2012.
- Comorbidity measures for use with administrative data. Med Care. 1998;36(1):8–27. , , , .
- Physician views on caring for hospitalized patients and the hospitalist model of inpatient care. J Gen Intern Med. 2001;16(2):116–119. , , .
- How physicians perceive hospitalist services after implementation: anticipation vs reality. Arch Intern Med. 2003;163(19):2330–2336. , , , .
- Association of communication between hospital‐based physicians and primary care providers with patient outcomes. J Gen Intern Med. 2009;24(3):381–386. , , , et al.
- Passing the clinical baton: 6 principles to guide the hospitalist. Dis Mon. 2002;48(4):260–266. , , .
- Primary care physician attitudes regarding communication with hospitalists. Am J Med. 2001;111(9B):15S–20S. , , , .
- Transitions of Care Consensus policy statement: American College of Physicians, Society of General Internal Medicine, Society of Hospital Medicine, American Geriatrics Society, American College Of Emergency Physicians, and Society for Academic Emergency Medicine. J Hosp Med. 2009;4(6):364–370. , , , et al.
- Hospital survey shows improvements in patient experience. Health Aff (Millwood). 2010;29(11):2061–2067. , , , et al.
- Characteristics of hospitals demonstrating superior performance in patient experience and clinical process measures of care. Med Care Res Rev. 2010;67(1):38–55. , , , , , .
- The impact of hospitalists on the cost and quality of inpatient care in the United States: a research synthesis. Med Care Res Rev. 2005;62(4):379–406. , .
- Quality of care for patients hospitalized with heart failure: assessing the impact of hospitalists. Arch Intern Med. 2002;162(11):1251–1256. , , , , .
- Hospitalists and the quality of care in hospitals. Arch Intern Med. 2009;169(15):1389–1394. , , , , .
- Quality of care for decompensated heart failure: comparable performance between academic hospitalists and non‐hospitalists. J Gen Intern Med. 2008;23(9):1399–1406. , , , et al.
- Patient satisfaction with hospital care provided by hospitalists and primary care physicians. J Hosp Med. 2012;7(2):131–136. , , , et al.
- Growth in the care of older patients by hospitalists in the United States. N Engl J Med. 2009;360(11):1102–1112. , , , .
- How do patients view the role of the primary care physician in inpatient care? Dis Mon. 2002;48(4):230–238. , , .
- Effects of survey mode, patient mix, and nonresponse on CAHPS hospital survey scores. Health Serv Res. 2009;44(2 pt 1):501–518. , , , et al.
- Development, implementation, and public reporting of the HCAHPS survey. Med Care Res Rev. 2010;67(1):27–37. , , , , .
- Patients' perception of hospital care in the United States. N Engl J Med. 2008;359(18):1921–1931. , , , .
- Agency for Healthcare Research and Quality. Healthcare Cost and Utilization Project (HCUP). HCUP Comorbidity Software. http://www.hcup‐us.ahrq.gov/toolssoftware/comorbidity/comorbidity.jsp Accessed November 12, 2012.
- Comorbidity measures for use with administrative data. Med Care. 1998;36(1):8–27. , , , .
- Physician views on caring for hospitalized patients and the hospitalist model of inpatient care. J Gen Intern Med. 2001;16(2):116–119. , , .
- How physicians perceive hospitalist services after implementation: anticipation vs reality. Arch Intern Med. 2003;163(19):2330–2336. , , , .
- Association of communication between hospital‐based physicians and primary care providers with patient outcomes. J Gen Intern Med. 2009;24(3):381–386. , , , et al.
- Passing the clinical baton: 6 principles to guide the hospitalist. Dis Mon. 2002;48(4):260–266. , , .
- Primary care physician attitudes regarding communication with hospitalists. Am J Med. 2001;111(9B):15S–20S. , , , .
- Transitions of Care Consensus policy statement: American College of Physicians, Society of General Internal Medicine, Society of Hospital Medicine, American Geriatrics Society, American College Of Emergency Physicians, and Society for Academic Emergency Medicine. J Hosp Med. 2009;4(6):364–370. , , , et al.
- Hospital survey shows improvements in patient experience. Health Aff (Millwood). 2010;29(11):2061–2067. , , , et al.
- Characteristics of hospitals demonstrating superior performance in patient experience and clinical process measures of care. Med Care Res Rev. 2010;67(1):38–55. , , , , , .
- The impact of hospitalists on the cost and quality of inpatient care in the United States: a research synthesis. Med Care Res Rev. 2005;62(4):379–406. , .
- Quality of care for patients hospitalized with heart failure: assessing the impact of hospitalists. Arch Intern Med. 2002;162(11):1251–1256. , , , , .
- Hospitalists and the quality of care in hospitals. Arch Intern Med. 2009;169(15):1389–1394. , , , , .
- Quality of care for decompensated heart failure: comparable performance between academic hospitalists and non‐hospitalists. J Gen Intern Med. 2008;23(9):1399–1406. , , , et al.
- Patient satisfaction with hospital care provided by hospitalists and primary care physicians. J Hosp Med. 2012;7(2):131–136. , , , et al.
Copyright © 2012 Society of Hospital Medicine